Sliced Wasserstein Discrepancy For Unsupervised Domain Adaptation
2019 IEEE/CVF CONFERENCE ON COMPUTER VISION AND PATTERN RECOGNITION (CVPR 2019)(2019)
摘要
In this work, we connect two distinct concepts for unsupervised domain adaptation: feature distribution alignment between domains by utilizing the task-specific decision boundary [57] and the Wasserstein metric [72]. Our proposed sliced Wasserstein discrepancy (SWD) is designed to capture the natural notion of dissimilarity between the outputs of task-specific classifiers. It provides a geometrically meaningful guidance to detect target samples that are far from the support of the source and enables efficient distribution alignment in an end-to-end trainable fashion. In the experiments, we validate the effectiveness and genericness of our method on digit and sign recognition, image classification, semantic segmentation, and object detection.
更多查看译文
关键词
Representation Learning,Deep Learning , Recognition: Detection,Categorization,Retrieval, Scene Analysis and Understanding
AI 理解论文
溯源树
样例
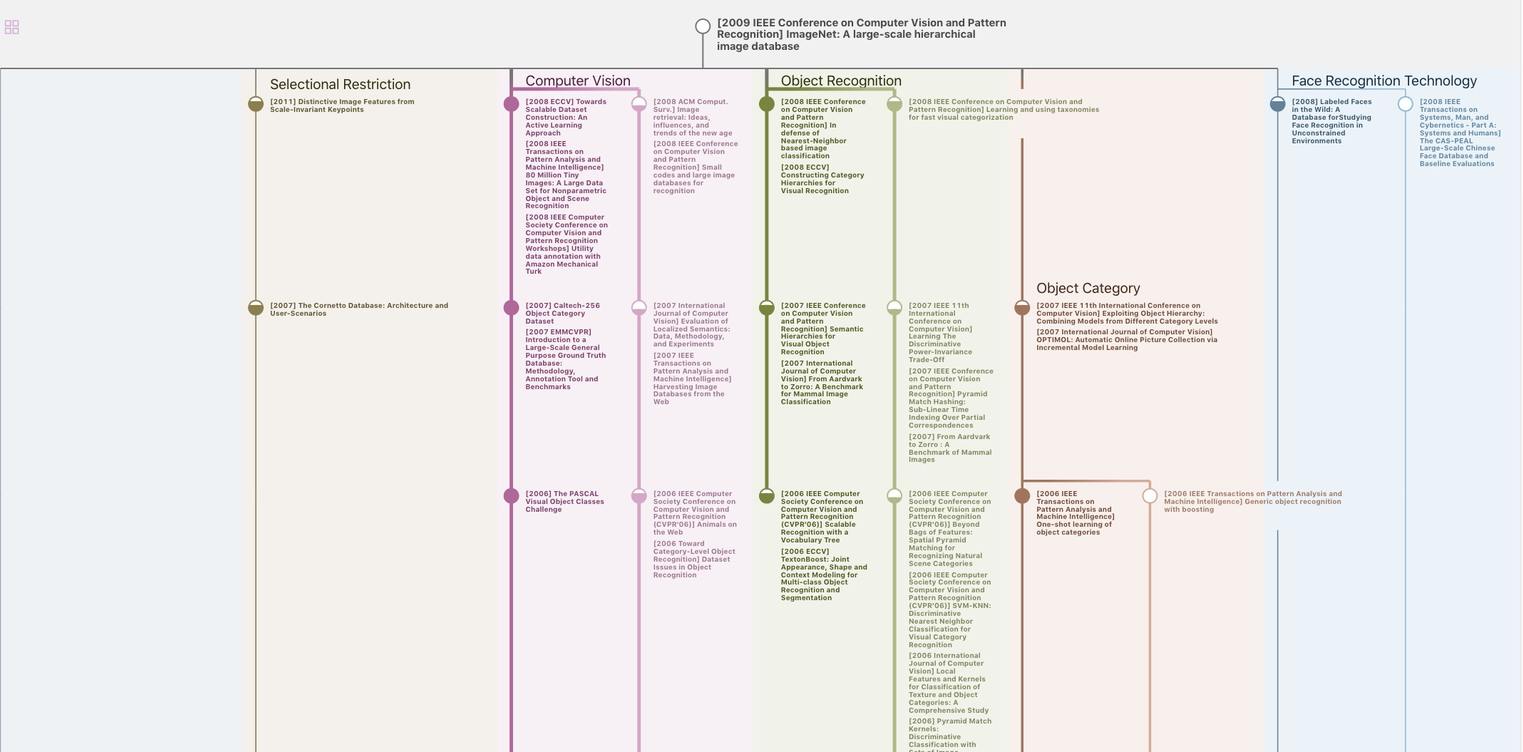
生成溯源树,研究论文发展脉络
Chat Paper
正在生成论文摘要