Hierarchy Denoising Recursive Autoencoders For 3d Scene Layout Prediction
2019 IEEE/CVF CONFERENCE ON COMPUTER VISION AND PATTERN RECOGNITION (CVPR 2019)(2019)
摘要
Indoor scenes exhibit rich hierarchical structure in 3D object layouts. Many tasks in 3D scene understanding can benefit from reasoning jointly about the hierarchical context of a scene, and the identities of objects. We present a variational denoising recursive autoencoder (VDRAE) that generates and iteratively refines a hierarchical representation of 3D object layouts, interleaving bottom-up encoding for context aggregation and top-down decoding for propagation. We train our VDRAE on large-scale 3D scene datasets to predict both instance-level segmentations and a 3D object detections from an over-segmentation of an input point cloud. We show that our VDRAE improves object detection performance on real-world 3D point cloud datasets compared to baselines from prior work.
更多查看译文
关键词
Scene Analysis and Understanding,Recognition: Detection,Categorization,Retrieval,Segmentation,Grouping and Shape
AI 理解论文
溯源树
样例
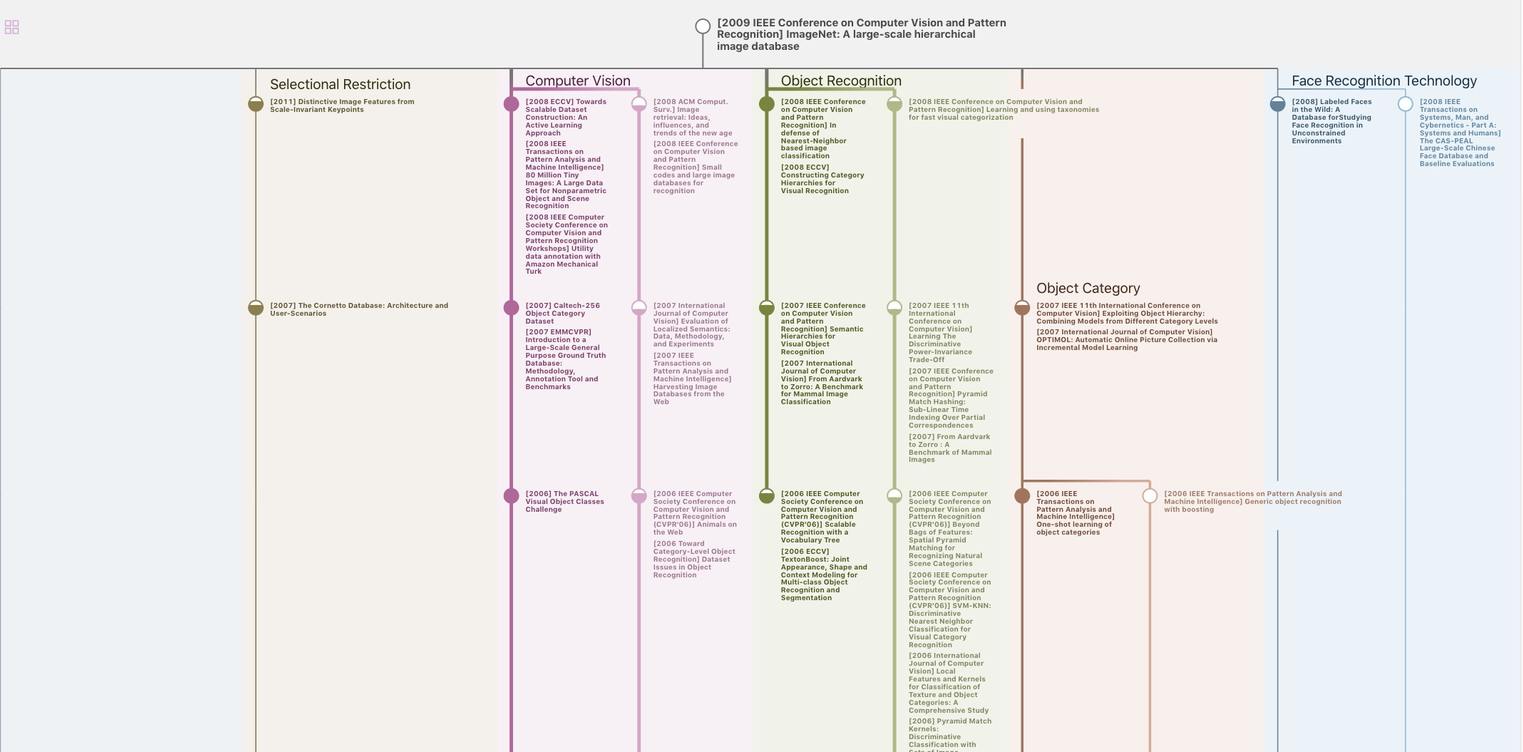
生成溯源树,研究论文发展脉络
Chat Paper
正在生成论文摘要