Improved appearance loss for deep estimation of image depth
Electronics Letters(2019)
摘要
Several recent deep network architectures tried to handle the depth estimation process as an image reconstruction problem, in order to overcome the shortfall that the ground truth depth data is not sufficiently available. The authors introduce and validate an efficient deeper network architecture for unsupervised depth estimation with an automated parameter optimisation. In addition, a hybrid appearance loss function is also proposed to improve the depth estimation accuracy and effectiveness. The authors' proposed model achieves the advantage that individual element of the loss function is weighted using normal distribution characteristics of a Gaussian model. The proposed ideas are validated on KITTI dataset achieving best reported results among recent state-of-the-art methods.
更多查看译文
关键词
image reconstruction,optimisation,unsupervised learning,neural net architecture,estimation theory
AI 理解论文
溯源树
样例
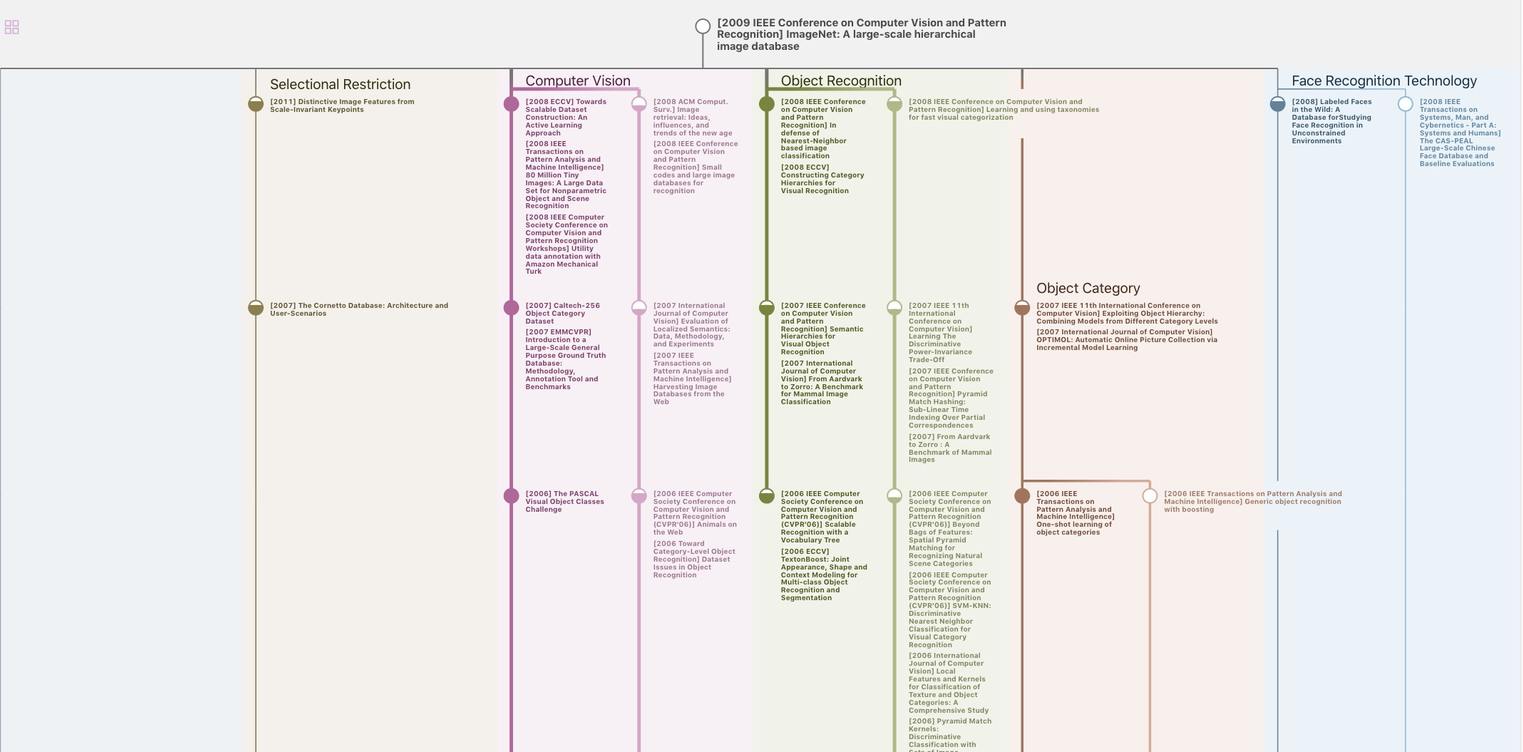
生成溯源树,研究论文发展脉络
Chat Paper
正在生成论文摘要