Extremum Seeking Control For Multi-Objective Optimization Problems
2016 IEEE 55TH CONFERENCE ON DECISION AND CONTROL (CDC)(2016)
摘要
Many real world applications require multiple conflicting objectives to be optimized. However, explicit functions mapping the system variables to outputs are often unknown, making traditional optimization approaches impossible. Extremum Seeking Control (ESC) is one method to tackle this problem by estimating the local gradient of the objective functions. Extensive literature on ESC focuses on single objective optimization, which use sinusoids as the dither signal. An inherent disadvantage with the sinusoidal nature of the dither is that after convergence the output continues to move around in a neighborhood of the optimal set-point, with the size of the neighborhood proportional to the amplitude of the dither signal. This paper focuses on using square waves as dithers and proves that the square wave can produce a constant output, in contrast to the typical sinusoid where the undesirable dithering effect still exists at the output. The basic ESC scheme is extended to execute Multiple Gradient Descent Algorithm (MGDA) to solve a Multi Objective Optimization Problem (MOOP). A two-stage ESC estimates the local gradient of each objective function, then estimates the optimal weighting of gradients to move to the Pareto front. This eliminates the need for a decision maker as required in a priori scalarization solutions a MOOP. Simulation results show that ESC using MGDA is able to find the Pareto optimal solutions, starting from different initial conditions.
更多查看译文
关键词
Convergence,Linear programming,Pareto optimization,Gallium nitride,Simulation,Filtering theory
AI 理解论文
溯源树
样例
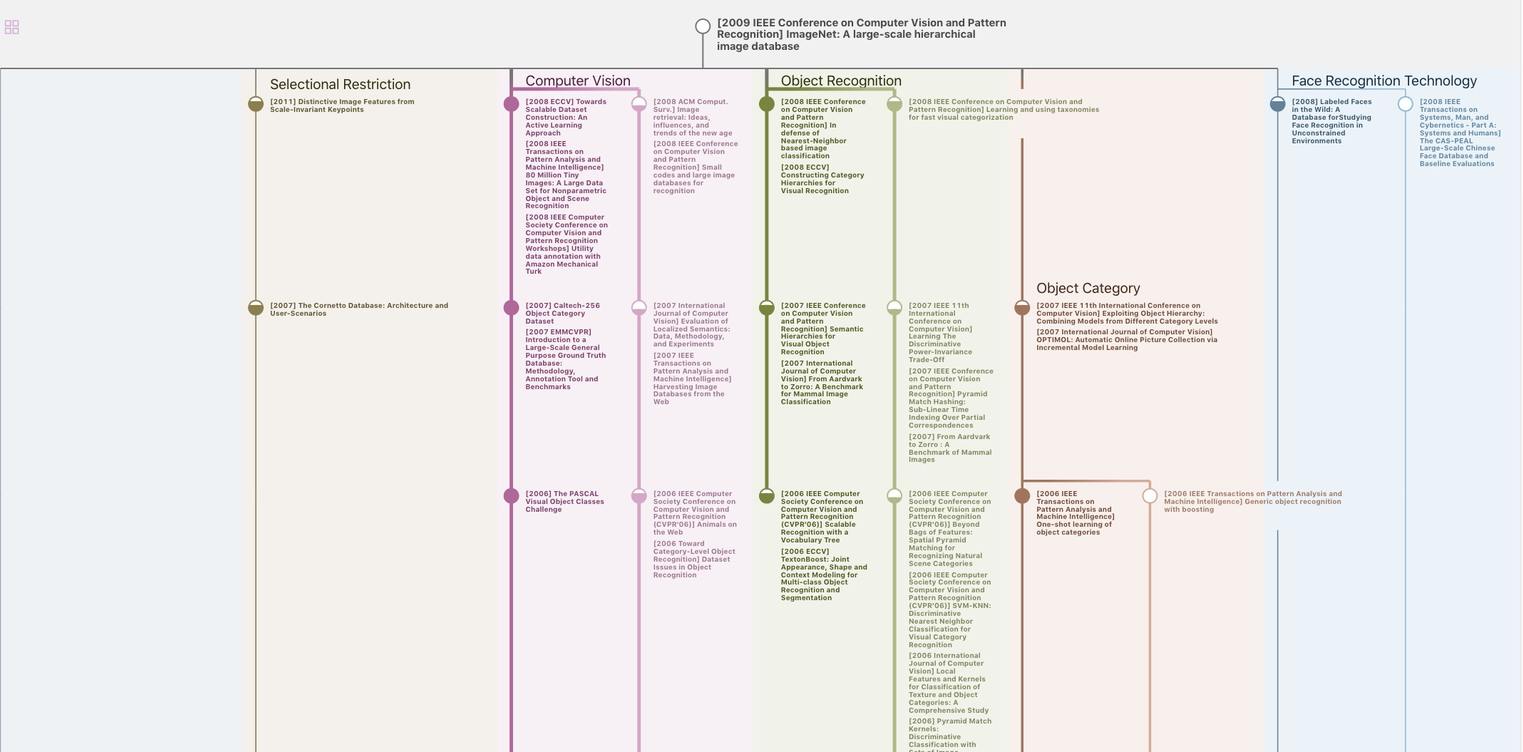
生成溯源树,研究论文发展脉络
Chat Paper
正在生成论文摘要