Group-constrained manifold learning
Pattern Recognition(2017)
摘要
The exploration of high-dimensional medical imaging datasets often requires the use of dimensionality reduction techniques. Generally, individual instances in longitudinal studies are not independent as several measurements of the same subjects, acquired at several time points, are included. Most non-linear dimensionality reduction techniques, that rely on defining a neighborhood graph to uncover local geometry, neglect potentially valuable grouping information and thus can lead to a poor estimation of the underlying manifold. Incorporating a-priori grouping knowledge of the data can lead to an improved low-dimensional representation. In this work, an approach to leverage such a-priori information is proposed, with the main interest in generating longitudinal biomarkers to assess a subject's risk of developing Alzheimer's disease (AD), and hence estimate time-to-conversion (TTC) and the mini mental state examination (MMSE) of subjects that develop AD. This is achieved via a novel formulation of the Laplacian Eigenmaps and Isomap manifold learning algorithms, that allows the incorporation of group-constraints. The potential of the proposed formulation was demonstrated on a swiss-roll toy example that clearly illustrates the problems faced by traditional techniques when additional structure is observed in the data. A real data experiment showed that the proposed constrained methods yielded improvements of ∼16% and ∼6% over the original unconstrained methods in k NN estimation of the TTC and MMSE on the ADNI database. • Laplacian Eigenmaps and Isomap novel formulation to incorporate group-constraints. • Improved time-to-conversion and MMSE estimation of MCI to AD converter subjects. • Coherent movement of the subjects in low-dimensional space as disease progresses.
更多查看译文
关键词
Alzheimer's disease,Manifold learning,Longitudinal,Neurodegeneration
AI 理解论文
溯源树
样例
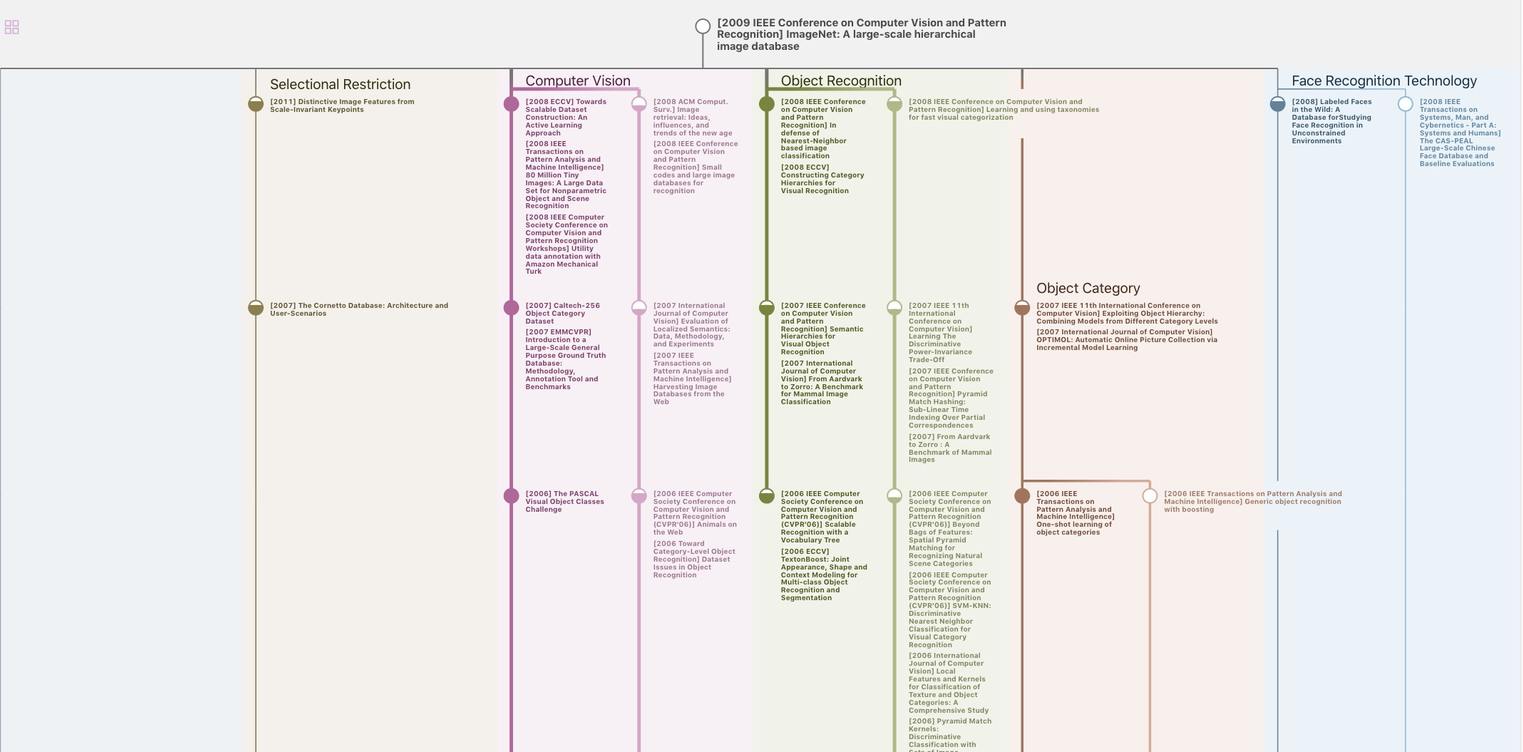
生成溯源树,研究论文发展脉络
Chat Paper
正在生成论文摘要