Learning Regularity In Skeleton Trajectories For Anomaly Detection In Videos
2019 IEEE/CVF CONFERENCE ON COMPUTER VISION AND PATTERN RECOGNITION (CVPR 2019)(2019)
摘要
Appearance features have been widely used in video anomaly detection even though they contain complex entangled factors. We propose a new method to model the normal patterns of human movements in surveillance video for anomaly detection using dynamic skeleton features. We decompose the skeletal movements into two sub-components: global body movement and local body posture. We model the dynamics and interaction of the coupled features in our novel Message-Passing Encoder-Decoder Recurrent Network. We observed that the decoupled features collaboratively interact in our spatio-temporal model to accurately identify human-related irregular events from surveillance video sequences. Compared to traditional appearance based models, our method achieves superior outlier detection performance. Our model also offers "open-box" examination and decision explanation made possible by the semantically understandable features and a network architecture supporting interpretability.
更多查看译文
关键词
Video Analytics,Deep Learning,Face,Gesture,and Body Pose,Motion and Tracking,Scene Analysis and Understanding
AI 理解论文
溯源树
样例
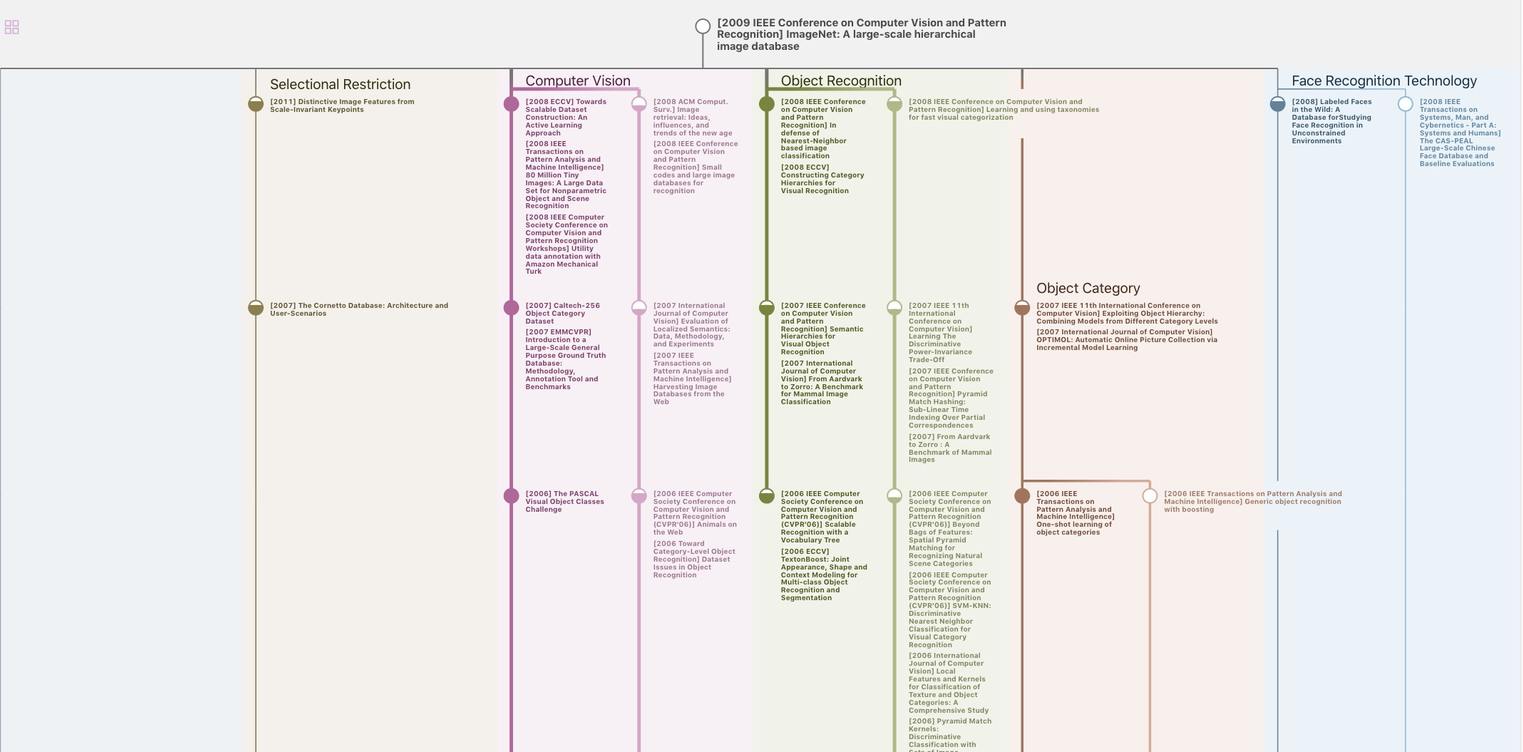
生成溯源树,研究论文发展脉络
Chat Paper
正在生成论文摘要