Underdetermined convolutive blind separation of sources integrating tensor factorization and expectation maximization.
Digital Signal Processing(2019)
摘要
Underdetermined convolutive blind separation of sources is a challenging topic in speech and audio processing. In plenty of conventional separation algorithms, the source signal estimation is performed in the frequency-domain, thus leading to permutation problems and poor separation results. In this paper, to solve the permutation alignment and obtain better source separation results, we exploit the algebraic structure of tensor factorization model and expectation–maximization (EM) to provide a new time-frequency algorithm. This is because tensor factorization has an advantage to estimate the channel for the underdetermined convolutive mixture case, and EM algorithm is conducive to faster converge to the desired solution and better source separating property. In the proposed algorithm, we first estimate the mixing matrix by using tensor decomposition, while permutation alignment algorithm is used to deal with the permutation problems. Then, the model parameters are updated using EM algorithm for improving source separation performance. At the same time, the spatial images of source signals are obtained using Wiener filters constructed from the estimated parameters. Furthermore, the time-domain source signals can be obtained through inverse short-time Fourier transform. Finally, a series of simulations show that compared with some existing separation algorithms, the proposed algorithm achieves better separation performance.
更多查看译文
关键词
Underdetermined blind separation of sources (UBSS),Convolutive mixtures,Tensor factorization,Permutation alignment,Expectation–maximization (EM)
AI 理解论文
溯源树
样例
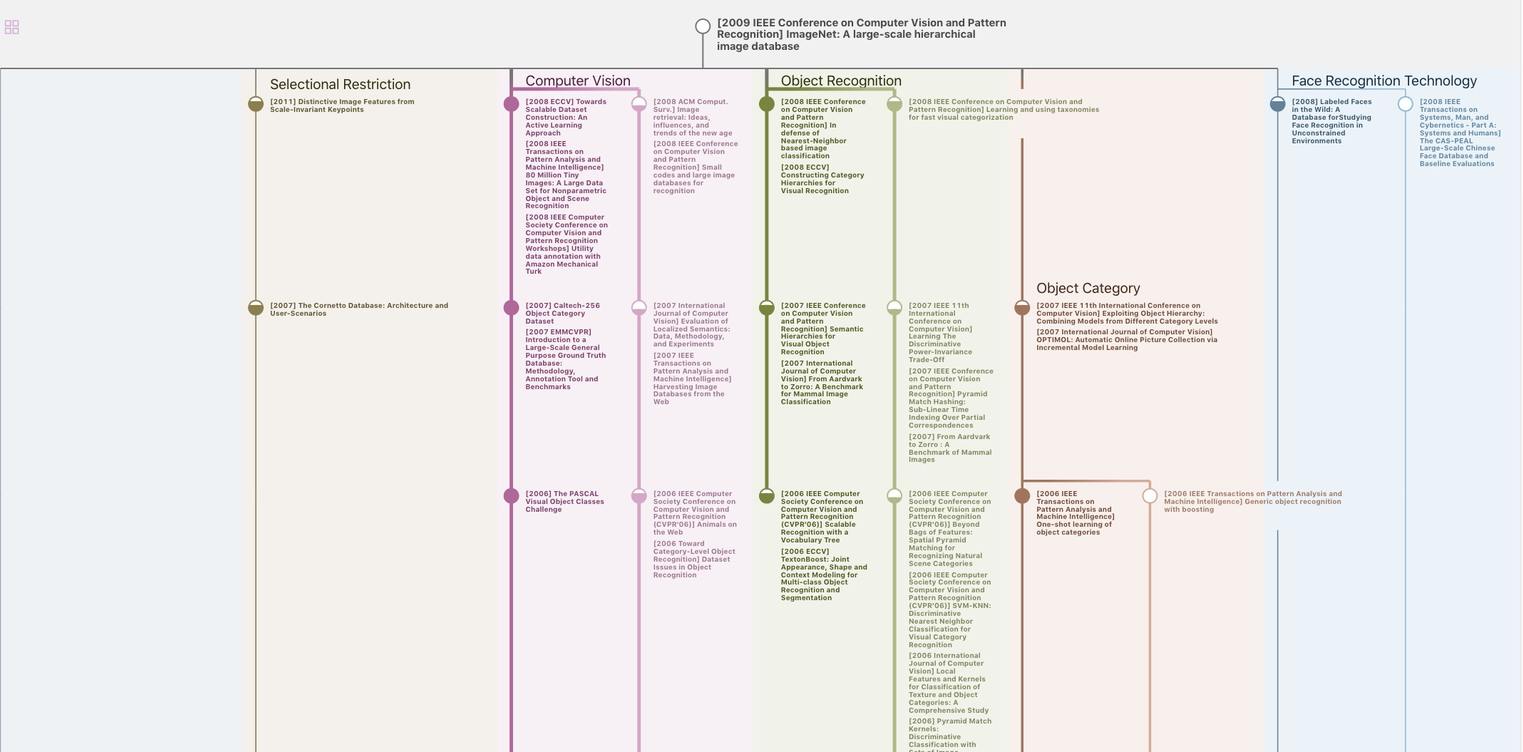
生成溯源树,研究论文发展脉络
Chat Paper
正在生成论文摘要