Derivative-Free Methods for Policy Optimization: Guarantees for Linear Quadratic Systems
22ND INTERNATIONAL CONFERENCE ON ARTIFICIAL INTELLIGENCE AND STATISTICS, VOL 89(2020)
摘要
We study derivative-free methods for policy optimization over the class of linear policies. We focus on characterizing the convergence rate of these methods when applied to linear-quadratic systems, and study various settings of driving noise and reward feedback. Our main theoretical result provides an explicit bound on the sample or evaluation complexity: we show that these methods are guaranteed to converge to within any pre-specified tolerance of the optimal policy with a number of zero-order evaluations that is an explicit polynomial of the error tolerance, dimension, and curvature properties of the problem. Our analysis reveals some interesting differences between the settings of additive driving noise and random initialization, as well as the settings of one-point and two-point reward feedback. Our theory is corroborated by simulations of derivative-free methods in application to these systems. Along the way, we derive convergence rates for stochastic zero-order optimization algorithms when applied to a certain class of non-convex problems.
更多查看译文
关键词
Derivative-Free Optimization, Linear Quadratic Control, Non-Convex Optimization
AI 理解论文
溯源树
样例
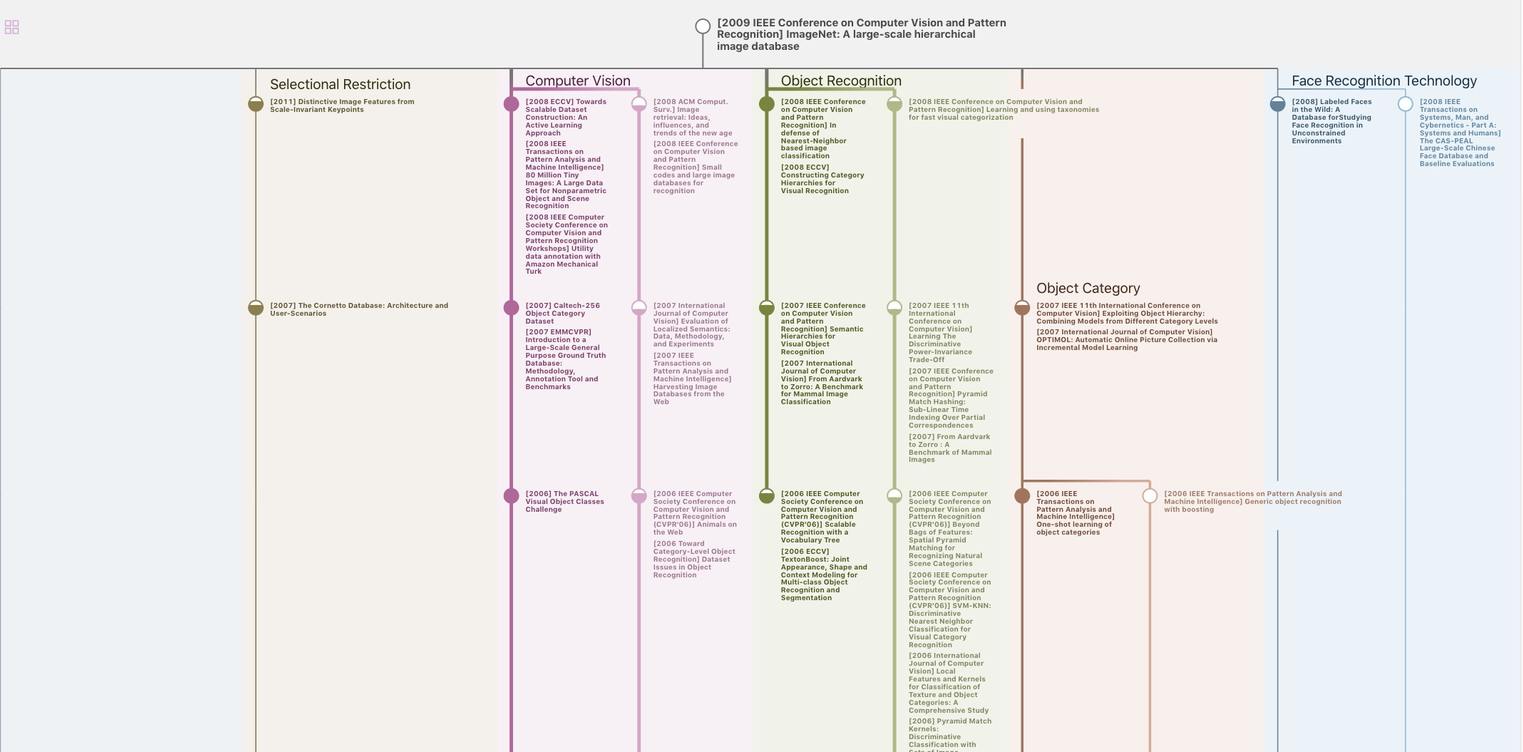
生成溯源树,研究论文发展脉络
Chat Paper
正在生成论文摘要