Divisively Normalized Sparse Coding: Toward Perceptual Visual Signal Representation
IEEE Transactions on Cybernetics(2021)
摘要
Sparse representation has been shown to be highly correlated with the visual perception of natural images, which can be characterized by a linear combination of neuronal responses in the visual cortex. Divisive normalization transform (DNT) has been proven to be an effective method in reducing statistical and perceptual dependencies for nonlinear properties in primary visual cortex. In this paper, we develop a divisively normalized sparse coding scheme, aiming to further bridge the gap between sparse representation and human visual perception. We show that such a scheme is perceptually meaningful for representing visual signals, with which the pixel-domain image representation and processing tasks can be feasibly and efficiently achieved in the divisively normalized sparse-domain. Specifically, we develop a sparse-domain similarity (SDS) index for perceptual quality evaluation, where the DNT is employed for transforming image signals into a perceptually uniform space. Furthermore, the proposed SDS index is employed to optimize the sparse coding process when representing natural images. The experimental results indicate that the SDS can provide accurate and consistent predictions of perceived image quality, and the performance of sparse coding can be significantly improved in terms of both objective and subjective quality evaluations.
更多查看译文
关键词
Divisive normalization,image quality assessment (IQA),sparse representation,visual perception
AI 理解论文
溯源树
样例
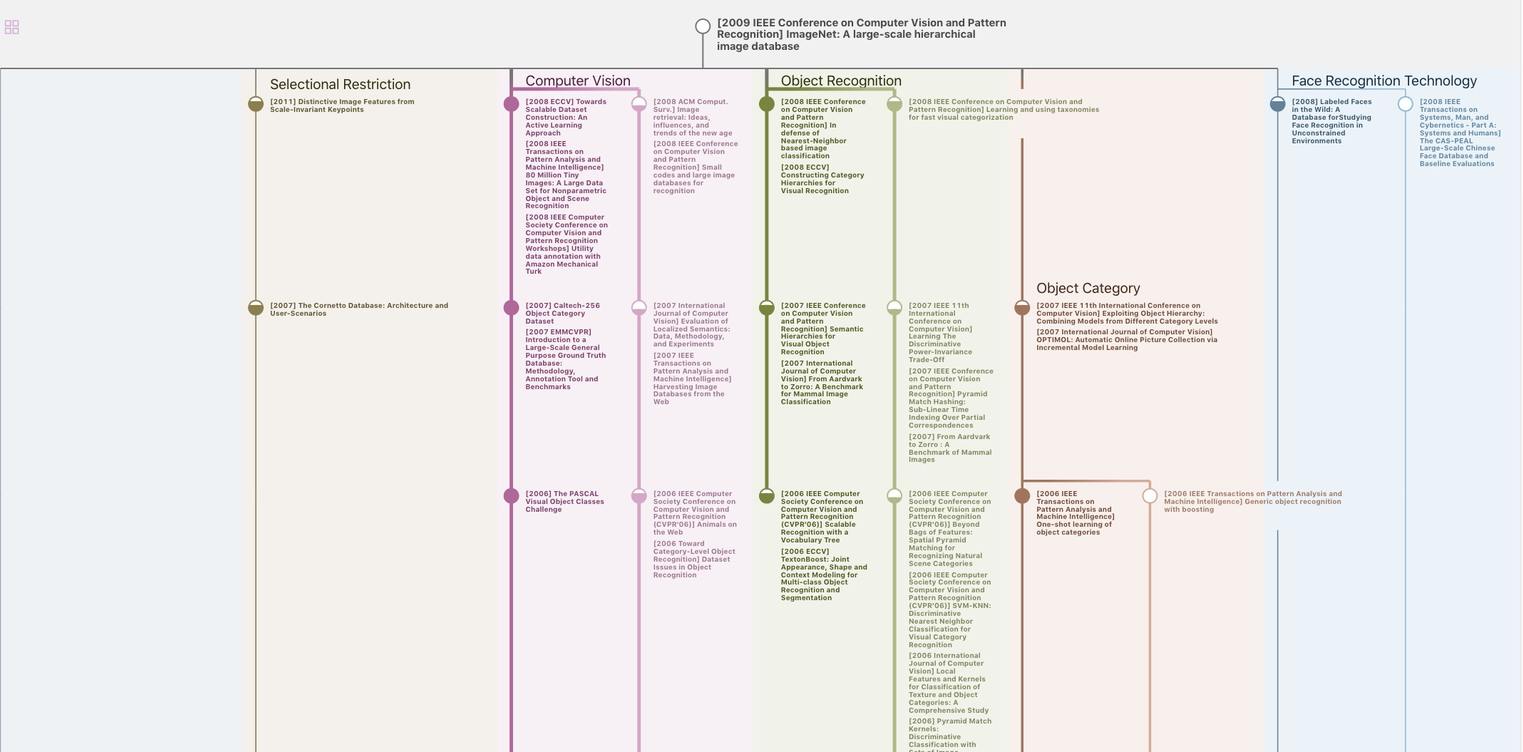
生成溯源树,研究论文发展脉络
Chat Paper
正在生成论文摘要