Parallel Multi-view Low-rank and Sparse Subspace Clustering for Unsupervised Hyperspectral Image Classification
2018 Asia-Pacific Signal and Information Processing Association Annual Summit and Conference (APSIPA ASC)(2018)
摘要
In this paper, parallel multi-view low-rank sparse subspace clustering (MLRSSC) is investigated for unsupervised classification of remotely sensed hyperspectral imagery. A 3-dimensional (3D) hyperspectral image contains abundant spectral and spatial information. Such diverse information can be considered as multiple views during the clustering process. In this paper, multiple spectral views are generated from correlated spectral band groups, a decorrelated and denoised view from principal components, and spatial views from morphological features. To make such a computational expensive clustering technique applicable to large-scale remote sensing images, parallel MLRSSC is implemented to non-overlapping 3D blocks. Experimental results demonstrate that the performance of the MLRSSC is better than other subspace clustering based algorithms.
更多查看译文
AI 理解论文
溯源树
样例
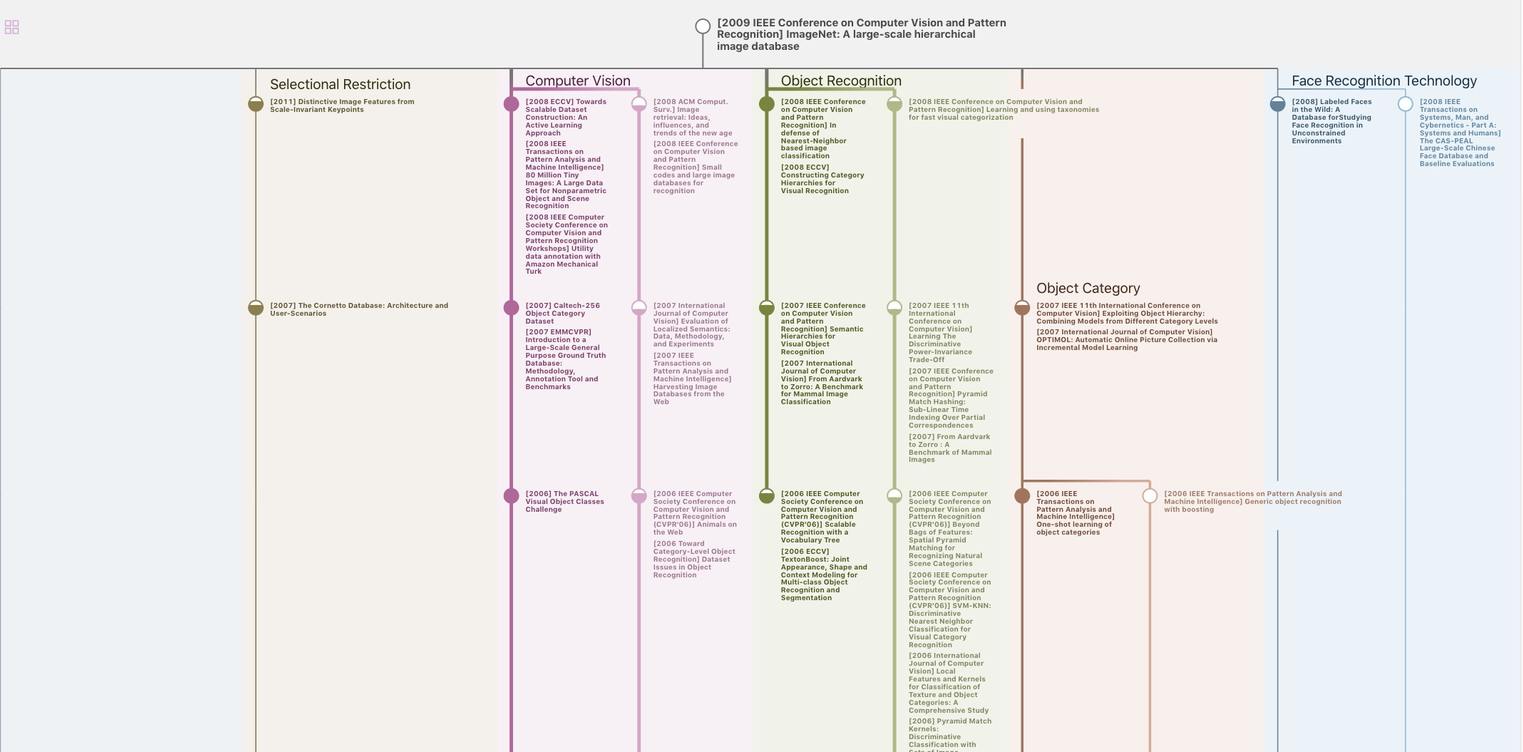
生成溯源树,研究论文发展脉络
Chat Paper
正在生成论文摘要