Deepturbo: Deep Turbo Decoder
2019 IEEE 20TH INTERNATIONAL WORKSHOP ON SIGNAL PROCESSING ADVANCES IN WIRELESS COMMUNICATIONS (SPAWC 2019)(2019)
摘要
Present-day communication systems routinely use codes that approach the channel capacity when coupled with a computationally efficient decoder. However, the decoder is typically designed for the Gaussian noise channel, and is known to be sub-optimal for non-Gaussian noise distribution. Deep learning methods offer a new approach for designing decoders that can be trained and tailored for arbitrary channel statistics. We focus on Turbo codes, and propose (DEEPTURBO), a novel deep learning based architecture for Turbo decoding.The standard Turbo decoder (TURBO) iteratively applies the Bahl-Cocke-Jelinek-Raviv (BCJR) algorithm with an inter-leaver in the middle. A neural architecture for Turbo decoding, termed (NEURALBCJR), was proposed recently to create a module that imitates the BCJR algorithm using supervised learning, and to use the interleaver architecture along with this module, which is then fine-tuned using end-to-end training. However, knowledge of the BCJR algorithm is required to design such an architecture, which also constrains the resulting learnt decoder. Here we remedy this requirement and propose a fully end-to-end trained neural decoder - Deep Turbo Decoder (DEEPTURBO). With novel learnable decoder structure and training methodology, DEEPTURBO reveals superior performance under both AWGN and non-AWGN settings as compared to the other two decoders - TURBO and NEURALBCJR.
更多查看译文
关键词
DEEPTURBO,channel capacity,computationally efficient decoder,Gaussian noise channel,nonGaussian noise distribution,arbitrary channel statistics,Turbo codes,deep learning based architecture,Bahl-Cocke-Jelinek-Raviv algorithm,neural architecture,TURBO decoding,BCJR algorithm,supervised learning,interleaver architecture,end-to-end training,learnable decoder structure,deep turbo decoder
AI 理解论文
溯源树
样例
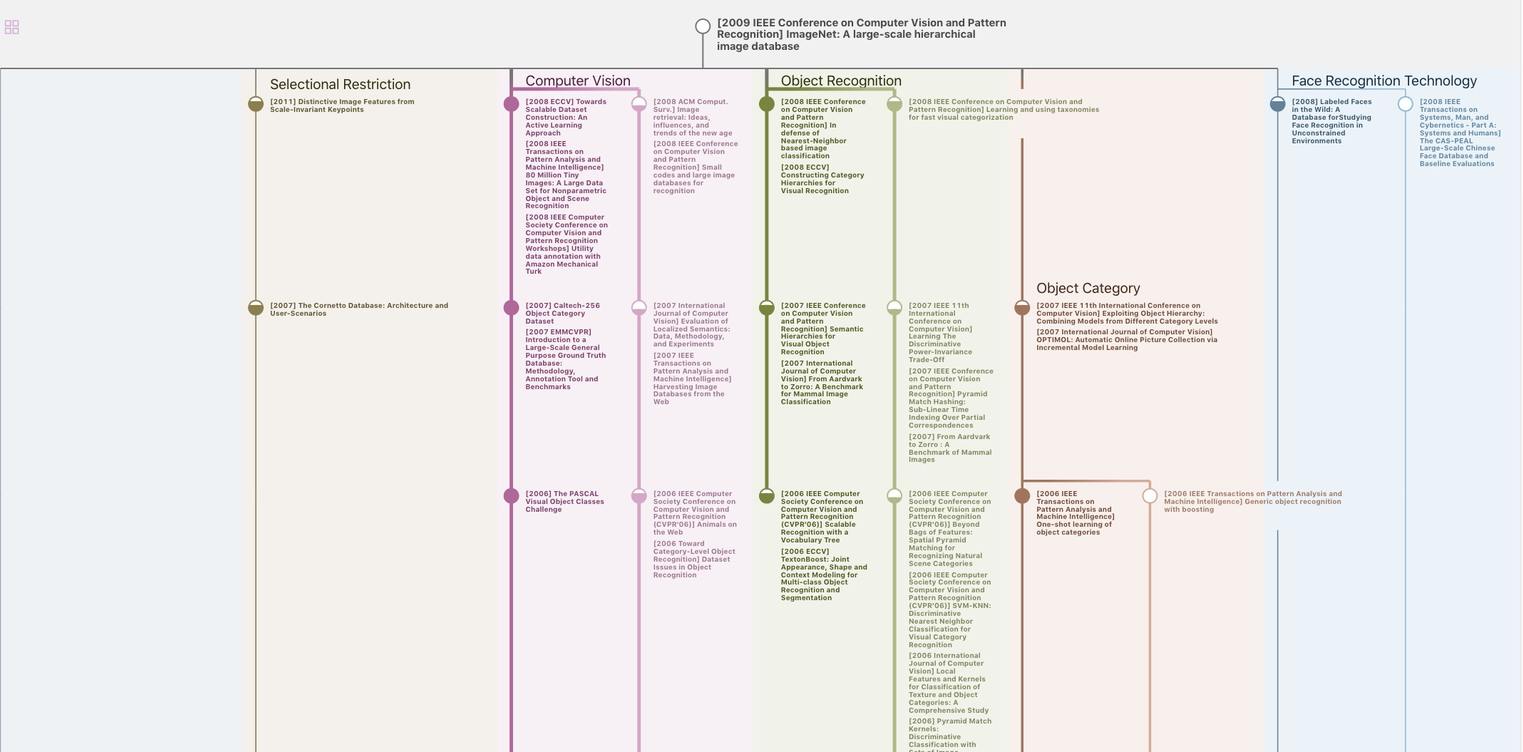
生成溯源树,研究论文发展脉络
Chat Paper
正在生成论文摘要