A deep learning framework for efficient analysis of breast volume and fibroglandular tissue using MR data with strong artifacts
International Journal of Computer Assisted Radiology and Surgery(2019)
摘要
Purpose The main purpose of this work is to develop, apply, and evaluate an efficient approach for breast density estimation in magnetic resonance imaging data, which contain strong artifacts including intensity inhomogeneities. Methods We present a pipeline for breast density estimation, which consists of intensity inhomogeneity correction, breast volume segmentation, nipple extraction, and fibroglandular tissue segmentation. For the segmentation steps, a well-known deep learning architecture is employed. Results The average Dice coefficient for the breast parenchyma is 92.5%± 0.011 , which outperforms the classical state-of-the-art approach by a margin of 9% . Conclusion The proposed solution is accurate and highly efficient and has potential to be applied for big epidemiological data with thousands of participants.
更多查看译文
关键词
Breast density, MRI, Segmentation, Deep learning
AI 理解论文
溯源树
样例
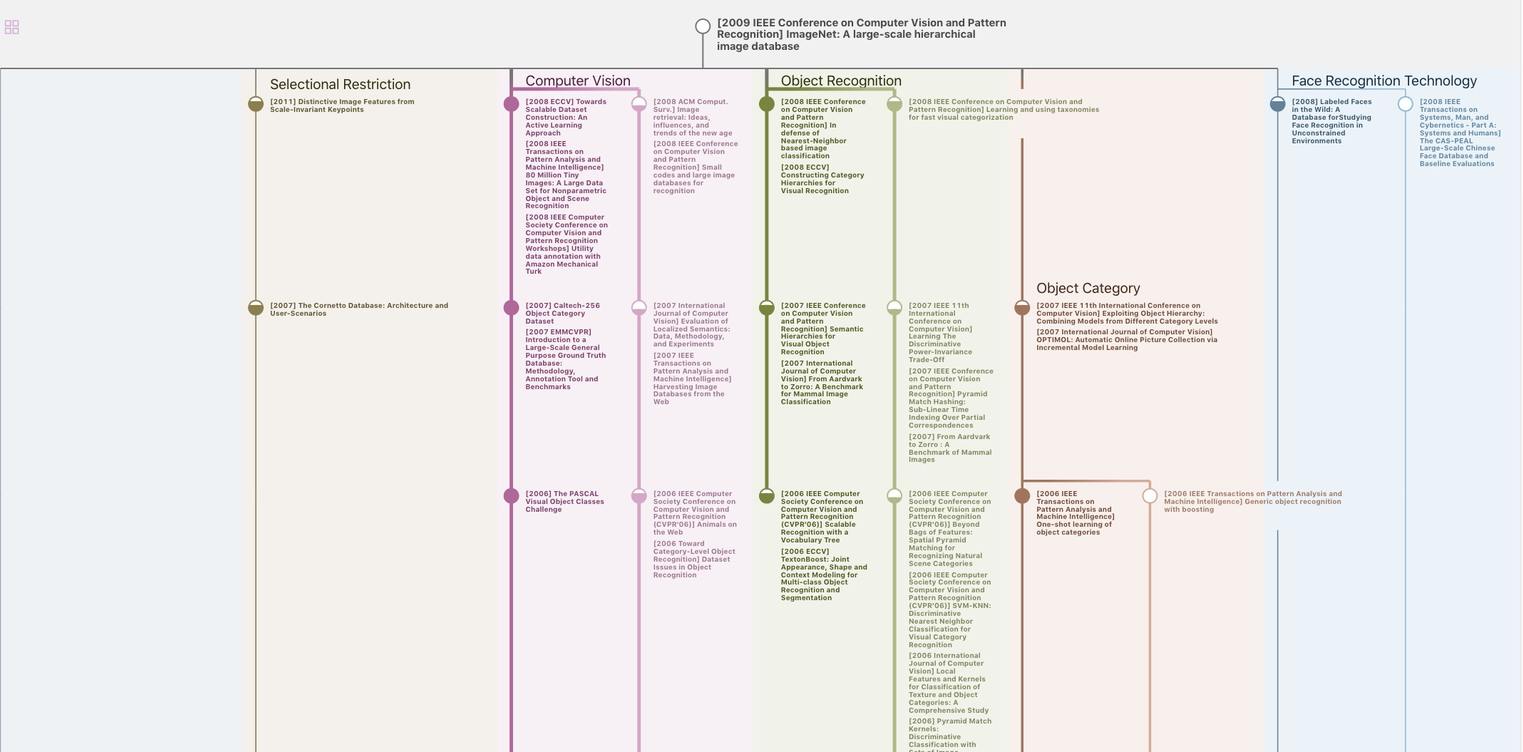
生成溯源树,研究论文发展脉络
Chat Paper
正在生成论文摘要