AdaSampling for Positive-Unlabeled and Label Noise Learning With Bioinformatics Applications.
IEEE transactions on cybernetics(2019)
摘要
Class labels are required for supervised learning but may be corrupted or missing in various applications. In binary classification, for example, when only a subset of positive instances is labeled whereas the remaining are unlabeled, positive-unlabeled (PU) learning is required to model from both positive and unlabeled data. Similarly, when class labels are corrupted by mislabeled instances, methods are needed for learning in the presence of class label noise (LN). Here we propose adaptive sampling (AdaSampling), a framework for both PU learning and learning with class LN. By iteratively estimating the class mislabeling probability with an adaptive sampling procedure, the proposed method progressively reduces the risk of selecting mislabeled instances for model training and subsequently constructs highly generalizable models even when a large proportion of mislabeled instances is present in the data. We demonstrate the utilities of proposed methods using simulation and benchmark data, and compare them to alternative approaches that are commonly used for PU learning and/or learning with LN. We then introduce two novel bioinformatics applications where AdaSampling is used to: 1) identify kinase-substrates from mass spectrometry-based phosphoproteomics data and 2) predict transcription factor target genes by integrating various next-generation sequencing data.
更多查看译文
关键词
Data models,Noise measurement,Bioinformatics,Training,Biological system modeling,Robustness,Australia
AI 理解论文
溯源树
样例
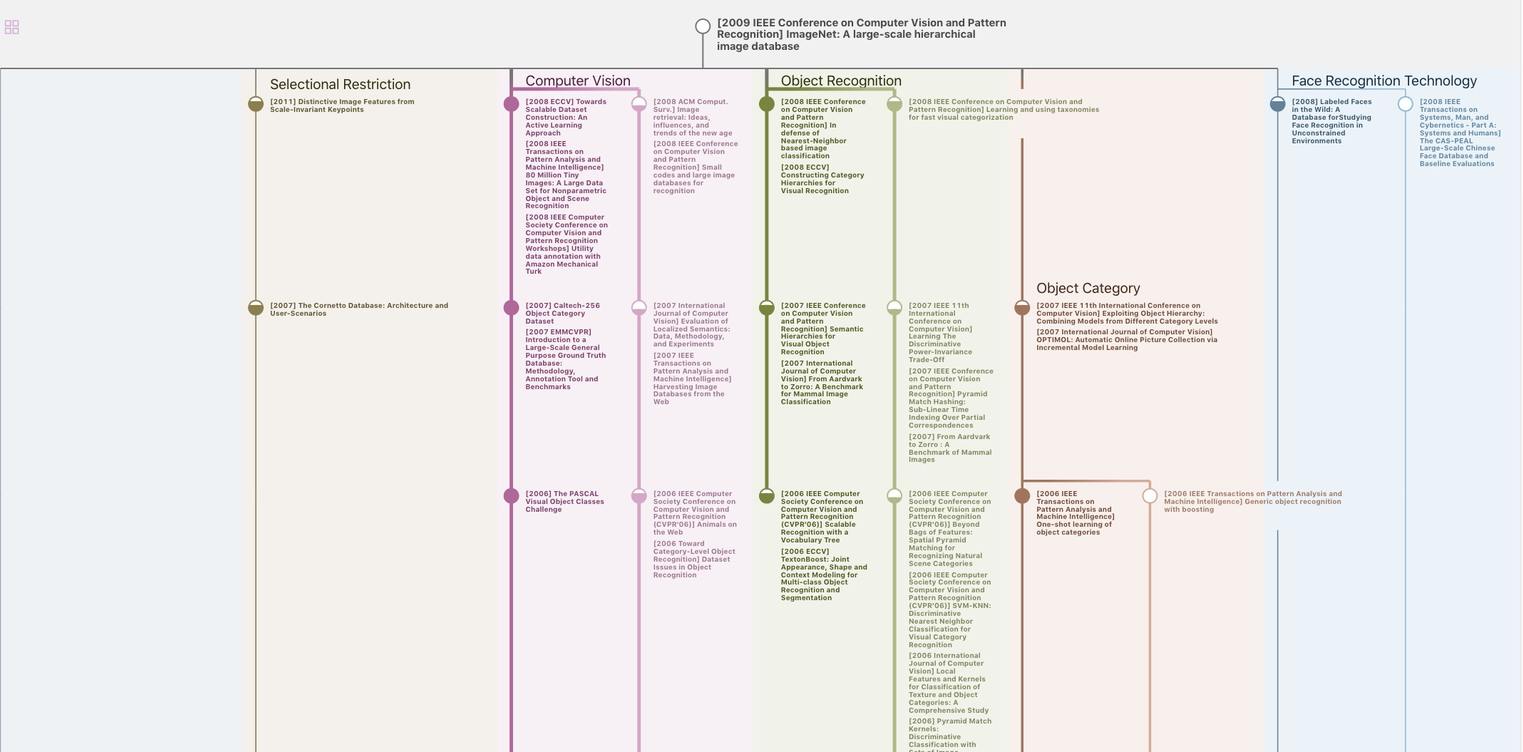
生成溯源树,研究论文发展脉络
Chat Paper
正在生成论文摘要