Domain agnostic online semantic segmentation for multi-dimensional time series.
Data mining and knowledge discovery(2018)
摘要
Unsupervised semantic segmentation in the time series domain is a much studied problem due to its potential to detect unexpected regularities and regimes in poorly understood data. However, the current techniques have several shortcomings, which have limited the adoption of time series semantic segmentation beyond academic settings for four primary reasons. First, most methods require setting/learning many parameters and thus may have problems generalizing to novel situations. Second, most methods implicitly assume that all the data is segmentable and have difficulty when that assumption is unwarranted. Thirdly, many algorithms are only defined for the single dimensional case, despite the ubiquity of multi-dimensional data. Finally, most research efforts have been confined to the batch case, but online segmentation is clearly more useful and actionable. To address these issues, we present a multi-dimensional algorithm, which is domain agnostic, has only one, easily-determined parameter, and can handle data streaming at a high rate. In this context, we test the algorithm on the largest and most diverse collection of time series datasets ever considered for this task and demonstrate the algorithm's superiority over current solutions.
更多查看译文
关键词
Online algorithms,Semantic segmentation,Time series
AI 理解论文
溯源树
样例
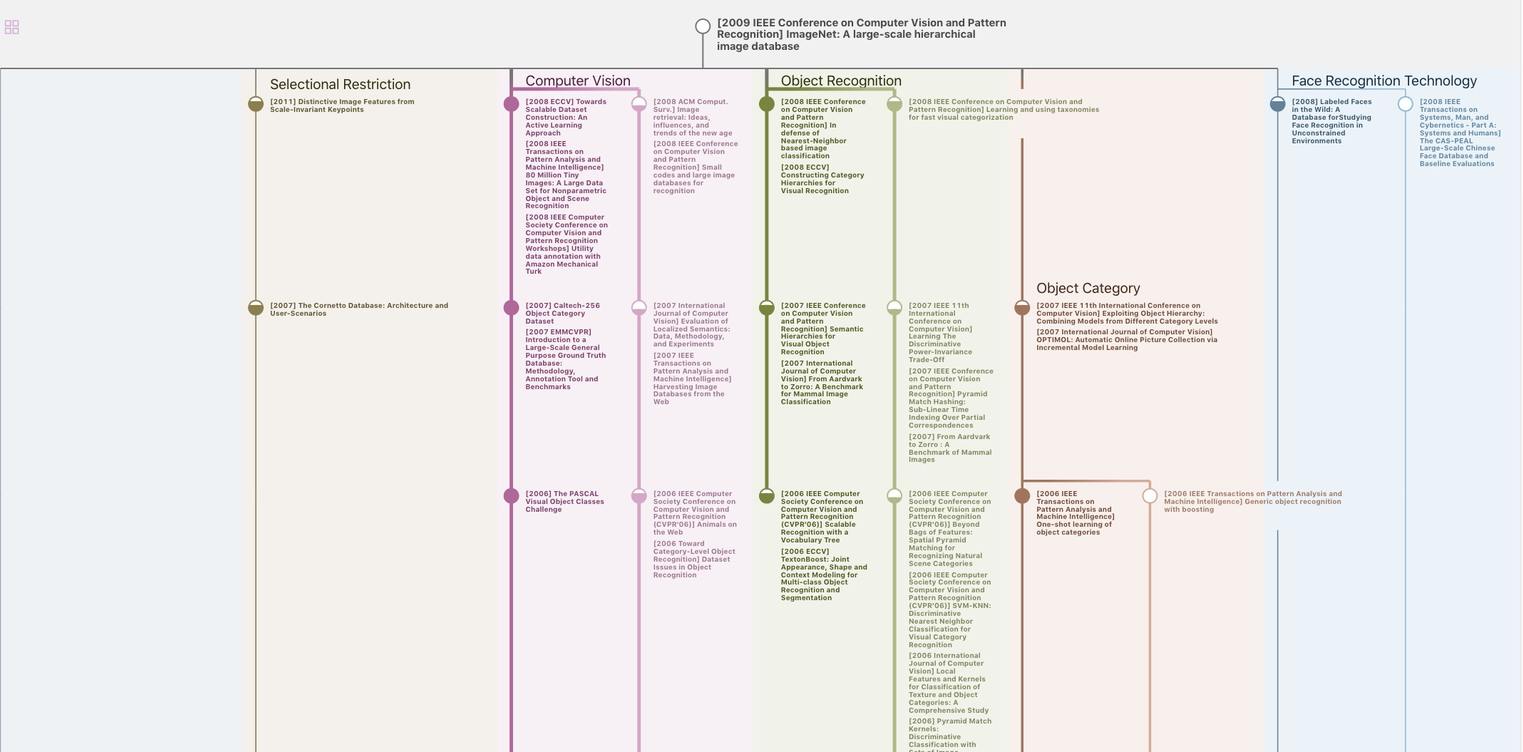
生成溯源树,研究论文发展脉络
Chat Paper
正在生成论文摘要