What if we ignore the random effects when analyzing RNA-seq data in a multifactor experiment.
STATISTICAL APPLICATIONS IN GENETICS AND MOLECULAR BIOLOGY(2016)
摘要
Identifying differentially expressed (DE) genes between different conditions is one of the main goals of RNA-seq data analysis. Although a large amount of RNA-seq data were produced for two-group comparison with small sample sizes at early stage, more and more RNA-seq data are being produced in the setting of complex experimental designs such as split-plot designs and repeated measure designs. Data arising from such experiments are traditionally analyzed by mixed-effects models. Therefore an appropriate statistical approach for analyzing RNA-seq data from such designs should be generalized linear mixed models (GLMM) or similar approaches that allow for random effects. However, common practices for analyzing such data in literature either treat random effects as fixed or completely ignore the experimental design and focus on two- group comparison using partial data. In this paper, we examine the effect of ignoring the random effects when analyzing RNA-seq data. We accomplish this goal by comparing the standard GLMM model to the methods that ignore the random effects through simulation studies and real data analysis. Our studies show that, ignoring random effects in a multi-factor experiment can lead to the increase of the false positives among the top selected genes or lower power when the nominal FDR level is controlled.
更多查看译文
关键词
generalized linear mixed models,method comparison,multifactor experiments,random effects,RNA-seq data analysis
AI 理解论文
溯源树
样例
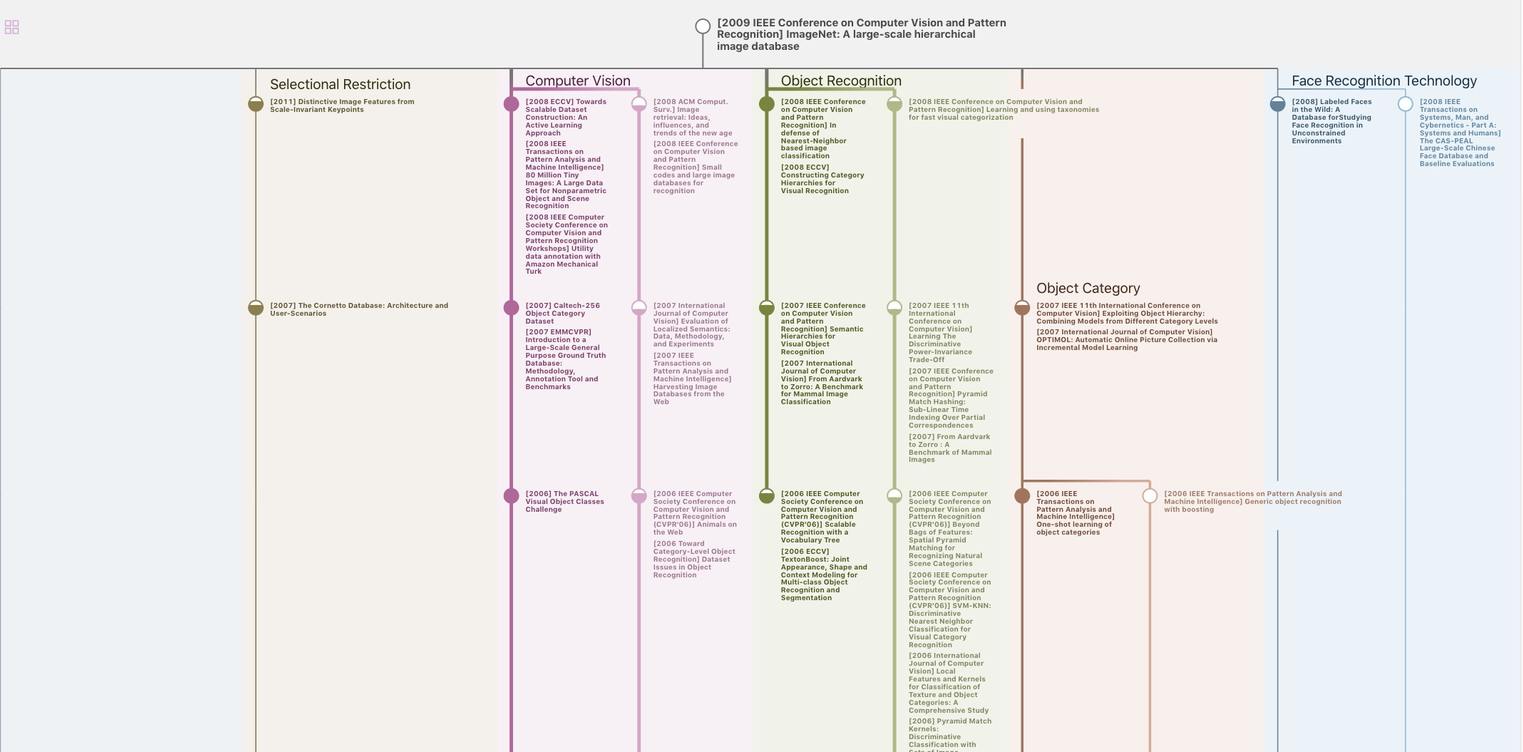
生成溯源树,研究论文发展脉络
Chat Paper
正在生成论文摘要