Unsupervised Abnormality Detection through Mixed Structure Regularization (MSR) in Deep Sparse Autoencoders.
MEDICAL PHYSICS(2019)
摘要
Purpose The purpose of this study is to introduce and evaluate the mixed structure regularization (MSR) approach for a deep sparse autoencoder aimed at unsupervised abnormality detection in medical images. Unsupervised abnormality detection based on identifying outliers using deep sparse autoencoders is a very appealing approach for computer-aided detection systems as it requires only healthy data for training rather than expert annotated abnormality. However, regularization is required to avoid overfitting of the network to the training data. Methods We used coronary computed tomography angiography (CCTA) datasets of 90 subjects with expert annotated centerlines. We segmented coronary lumen and wall using an automatic algorithm with manual corrections where required. We defined normal coronary cross section as cross sections with a ratio between lumen and wall areas larger than 0.8. We divided the datasets into training, validation, and testing groups in a tenfold cross-validation scheme. We trained a deep sparse overcomplete autoencoder model for normality modeling with random structure and noise augmentation. We assessed the performance of our deep sparse autoencoder with MSR without denoising (SAE-MSR) and with denoising (SDAE-MSR) in comparison to deep sparse autoencoder (SAE), and deep sparse denoising autoencoder (SDAE) models in the task of detecting coronary artery disease from CCTA data on the test group. Results The SDAE-MSR achieved the best aggregated area under the curve (AUC) with a 20% improvement and the best aggregated Average Precision (AP) with a 30% improvement upon the SAE and SDAE (AUC: 0.78 to 0.94, AP: 0.66 to 0.86) in distinguishing between coronary cross sections with mild stenosis (stenosis grade < 0.3) and coronary cross sections with severe stenosis (stenosis grade > 0.7). The improvements were statistically significant (Mann-Whitney U-test, P < 0.001). Similarly, The SDAE-MSR achieved the best aggregated AUC (AP) with an 18% (18%) improvement upon the SAE and SDAE (AUC: 0.71 to 0.84, AP: 0.68 to 0.80). The improvements were statistically significant (Mann-Whitney U-test, P < 0.05). Conclusion Deep sparse autoencoders with MSR in addition to explicit sparsity regularization term and stochastic corruption of the input data with Gaussian noise have the potential to improve unsupervised abnormality detection using deep-learning compared to common deep autoencoders.
更多查看译文
关键词
abnormality detection,coronary computed tomography angiography,deep sparse overcomplete autoencoder,regularization
AI 理解论文
溯源树
样例
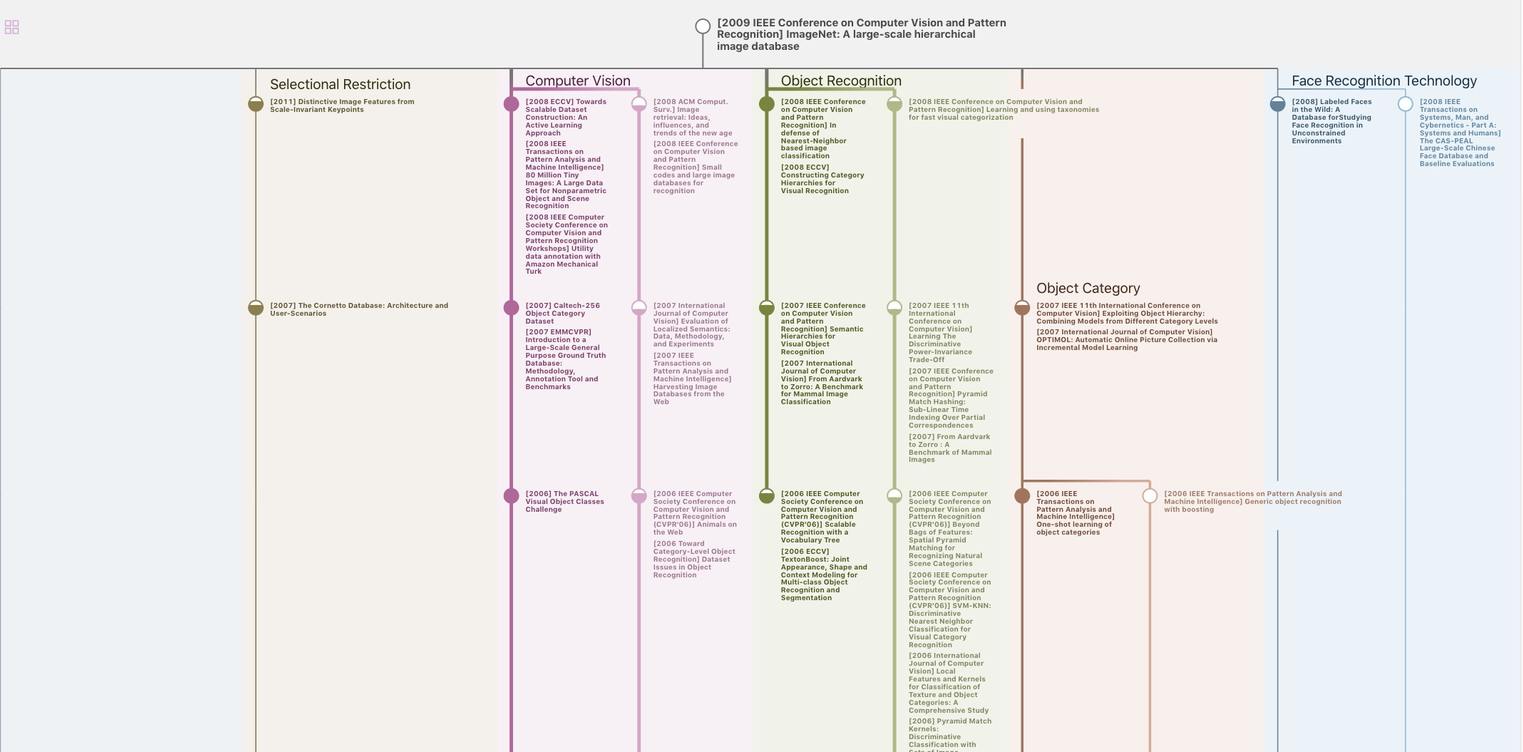
生成溯源树,研究论文发展脉络
Chat Paper
正在生成论文摘要