Efficiently Updating the Discovered Multiple Fuzzy Frequent Itemsets with Transaction Insertion
Int. J. Fuzzy Syst.(2018)
摘要
Most pattern mining approaches such as association rule mining or frequent itemsets mining can only handle, however, the binary database in which each item or attribute is represented as 0 or 1. In real-life situations, data may be represented as more complicatedly. Moreover, it is more meaningful for human begins to understand if the discovered rules can be represented as the linguistic terms. Fuzzy-set theory was adopted to handle the quantitative database, which transforms the quantity value into the linguistic terms with their fuzzy values based on the pre-defined membership functions. Several algorithms were designed to handle this issue, but most of them focus on mining the fuzzy rules in the static database. When the database size is changed, the whole updated database should be rescanned again to obtain the up-to-date information. This progress ignores the already discovered knowledge with its computational cost, which is inefficient in dynamic applications. In this paper, we present an incremental multiple fuzzy frequent itemset mining with transaction INSertion (IMF-INS) algorithm to efficiently update the multiple fuzzy frequent itemsets from the quantitative dataset. The designed IMF-INS utilizes the fuzzy FUP concept to divide the transformed linguistic terms into four cases. Each case is performed by the designed approach to update the discovered information. Also, the fuzzy list structure is utilized to reduce the generation of candidates without multiple database scans. Experiments are conducted to show the performance of the proposed algorithm in terms of runtime, memory consumption, number of determined patterns and scalability. Results indicated that the designed IMF-INS outperforms the state-of-the-art batch mining progresses.
更多查看译文
关键词
Multiple fuzzy regions, Frequent itemset, Dynamic data mining, Transaction insertion, Incremental
AI 理解论文
溯源树
样例
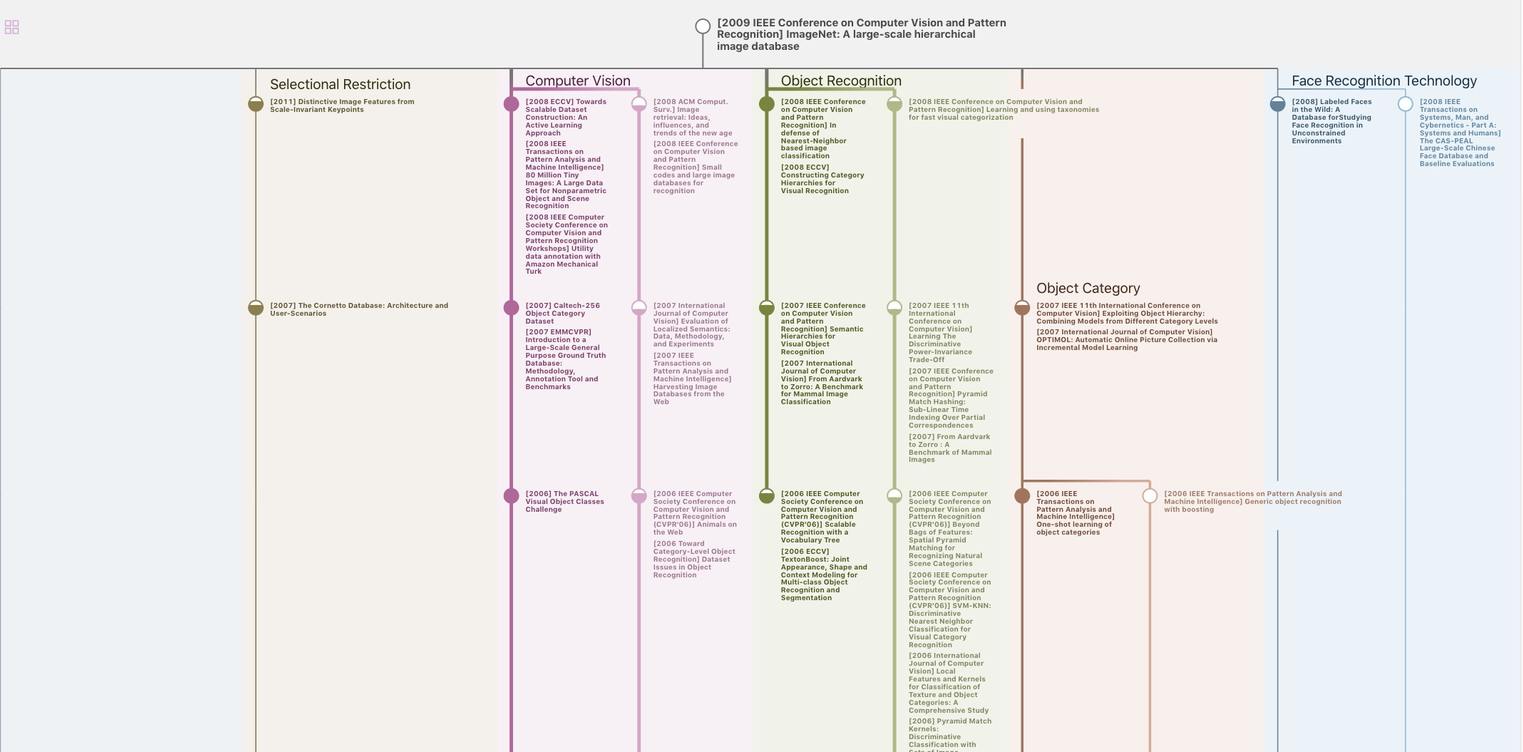
生成溯源树,研究论文发展脉络
Chat Paper
正在生成论文摘要