Optimizing Local Probability Models for Statistical Parsing
Lecture Notes in Artificial Intelligence(2003)
摘要
This paper studies the properties and performance of models for estimating local probability distributions which are used as components of larger probabilistic systems - history-based generative parsing models. We report experimental results showing that memory-based learning outperforms many commonly used methods for this task (Witten-Bell, Jelinek-Mercer with fixed weights, decision trees, and log-linear models). However, we can connect these results with the commonly used general class of deleted interpolation models by showing that certain types of memory-based learning, including the kind that performed so well in our experiments, are instances of this class. In addition, we illustrate the divergences between joint and conditional data likelihood and accuracy performance achieved by such models, suggesting that smoothing based on optimizing accuracy directly might greatly improve performance.
更多查看译文
关键词
computer science,probability distribution,decision tree,log linear model
AI 理解论文
溯源树
样例
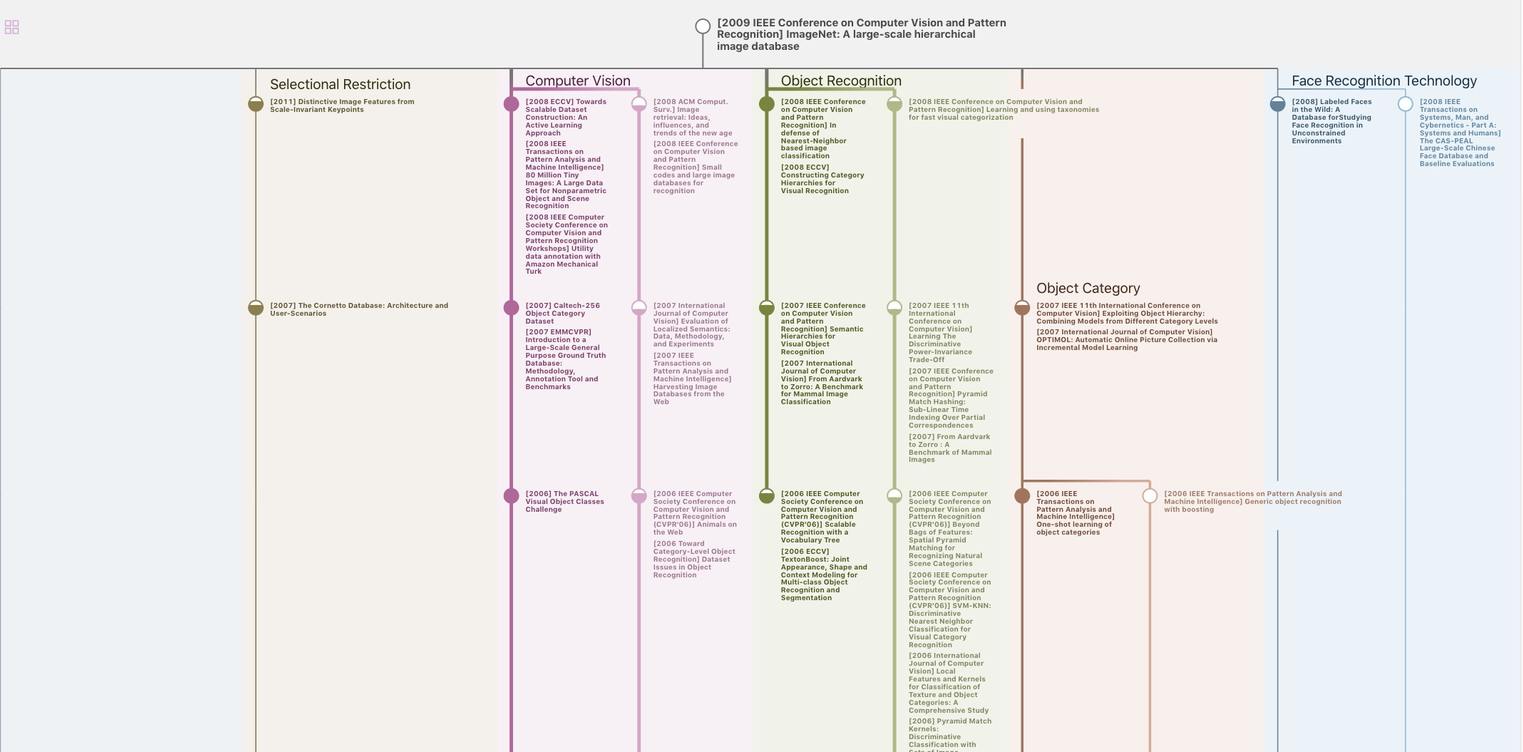
生成溯源树,研究论文发展脉络
Chat Paper
正在生成论文摘要