3D HIGH-RESOLUTION CARDIAC SEGMENTATION RECONSTRUCTION FROM 2D VIEWS USING CONDITIONAL VARIATIONAL AUTOENCODERS
2019 IEEE 16TH INTERNATIONAL SYMPOSIUM ON BIOMEDICAL IMAGING (ISBI 2019)(2019)
摘要
Accurate segmentation of heart structures imaged by cardiac MR is key for the quantitative analysis of pathology. High resolution 3D MR sequences enable whole-heart structural imaging but are time-consuming, expensive to acquire and they often require long breath holds that are not suitable for patients. Consequently, multiplanar breath-hold 2D cines sequences are standard practice but are disadvantaged by lack of whole-heart coverage and low through-plane resolution. To address this, we propose a conditional variational autoencoder architecture able to learn a generative model of 3D high-resolution left ventricular (LV) segmentations which is conditioned on three 21) LV segmentations of one short-axis and two long-axis images. By only employing these three 2D segmentations, our model can efficiently reconstruct the 3D high-resolution LV segmentation of a subject. When evaluated on 400 unseen healthy volunteers, our model yielded an average Dice score of 87.92 +/- 0.15 and outperformed competing architectures (TL-net, Dice score = 82.60 +/- 0.23, p = 2.2 . 10(-16)).
更多查看译文
关键词
Cardiac MR,Variational Autoencoder,3D Segmentation Reconstruction,Deep Learning
AI 理解论文
溯源树
样例
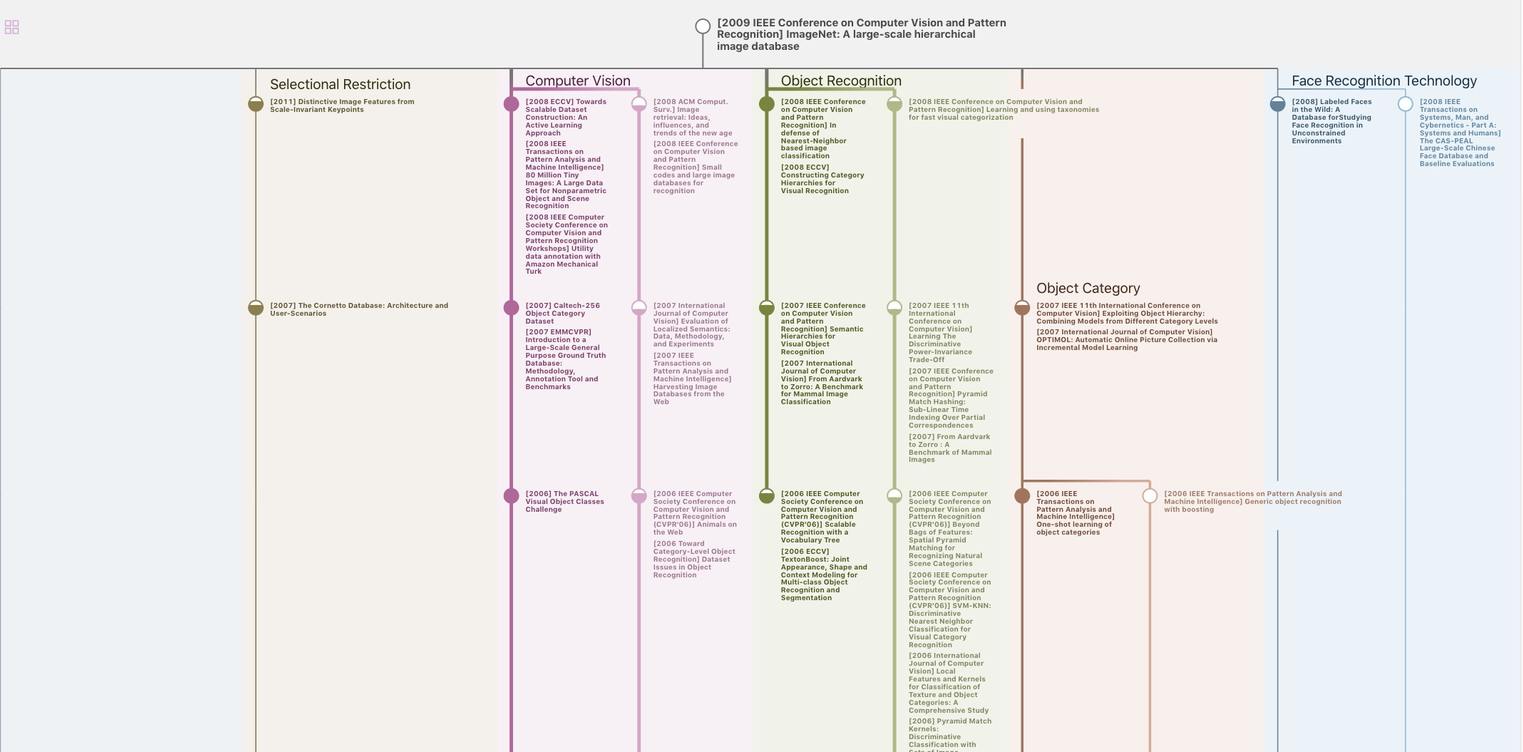
生成溯源树,研究论文发展脉络
Chat Paper
正在生成论文摘要