Locally Adaptive Kernel Estimation Using Sparse Functional Programming
2018 CONFERENCE RECORD OF 52ND ASILOMAR CONFERENCE ON SIGNALS, SYSTEMS, AND COMPUTERS(2018)
摘要
Reproducing Kernel Hilbert Space (RKHS)-based methods are widely used in signal processing and machine learning applications. Yet, they suffer from a parameter selection issue: selecting the RKHS in which to operate (or even the kernel parameter) is often a significant challenge. Moreover, since the RKHS determines properties such as shape and smoothness of the learned function, its choice affects the effectiveness of these techniques. Likewise, due to the homogeneous smoothness of functions promoted by these solutions, they are poor estimators for functions with varying degrees of smoothness. To overcome these limitations, we propose to locally adapt the RKHS (more specifically, its smoothness parameter) over which we seek to perform function estimation by using a sparse functional program, Under this formulation, we must solve an infinite dimensional, non-convex optimization problem. This problem, however, has zero duality gap and can therefore be solved exactly and efficiently in the dual domain using, for instance, gradient ascent techniques.
更多查看译文
关键词
Kernel regression, bandwidth adaptation, sparsity, sparse functional optimization
AI 理解论文
溯源树
样例
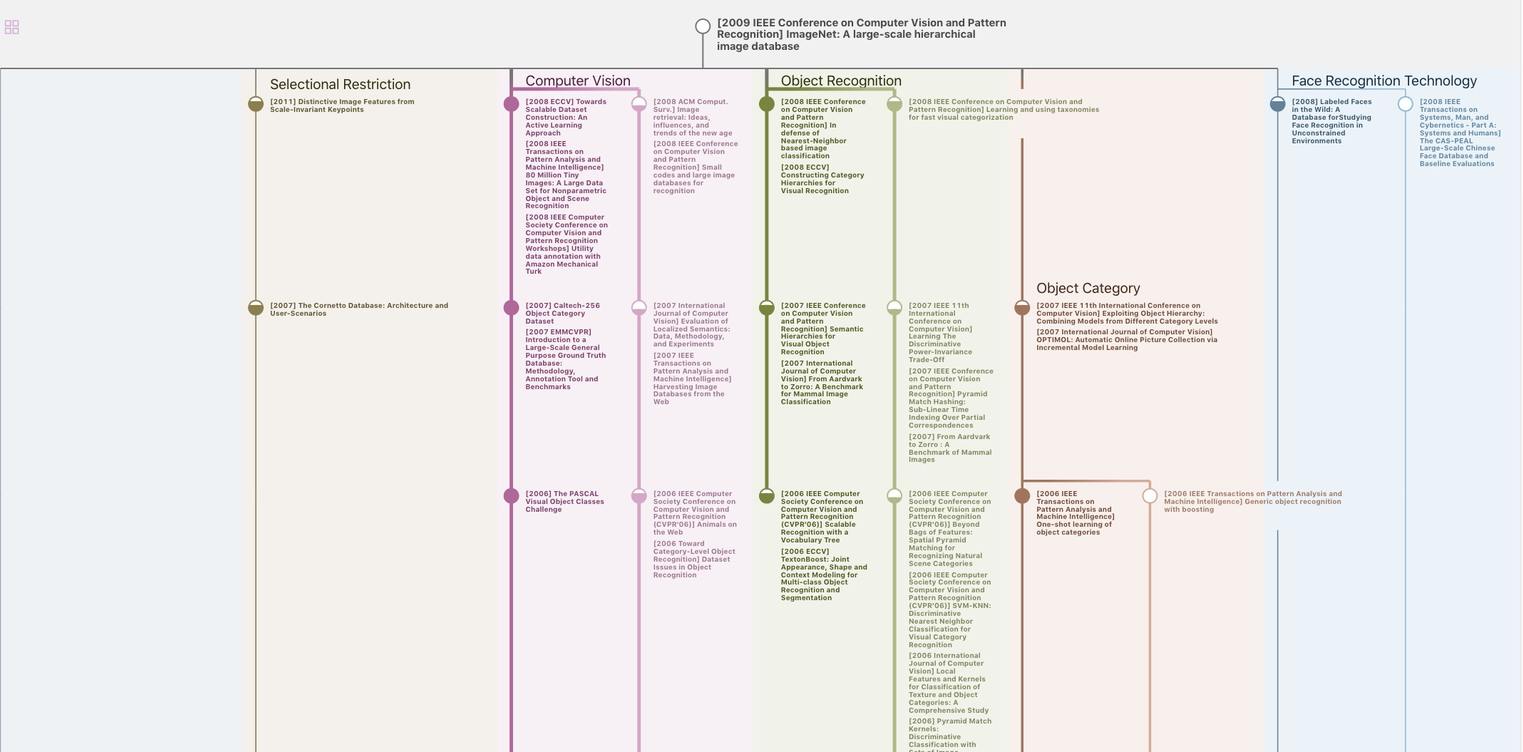
生成溯源树,研究论文发展脉络
Chat Paper
正在生成论文摘要