Kernel Mean Shrinkage Estimators.
Journal of Machine Learning Research(2016)
摘要
A mean function in a reproducing kernel Hilbert space (RKHS), or a kernel mean, is central to kernel methods in that it is used by many classical algorithms such as kernel principal component analysis, and it also forms the core inference step of modern kernel methods that rely on embedding probability distributions in RKHSs. Given a finite sample, an empirical average has been used commonly as a standard estimator of the true kernel mean. Despite a widespread use of this estimator, we show that it can be improved thanks to the well-known Stein phenomenon. We propose a new family of estimators called kernel mean shrinkage estimators (KMSEs), which bene fit from both theoretical justifications and good empirical performance. The results demonstrate that the proposed estimators outperform the standard one, especially in a "large d, small n" paradigm.
更多查看译文
关键词
covariance operator,James-Stein estimators,kernel methods,kernel mean,shrinkage estimators,Stein effect,Tikhonov regularization
AI 理解论文
溯源树
样例
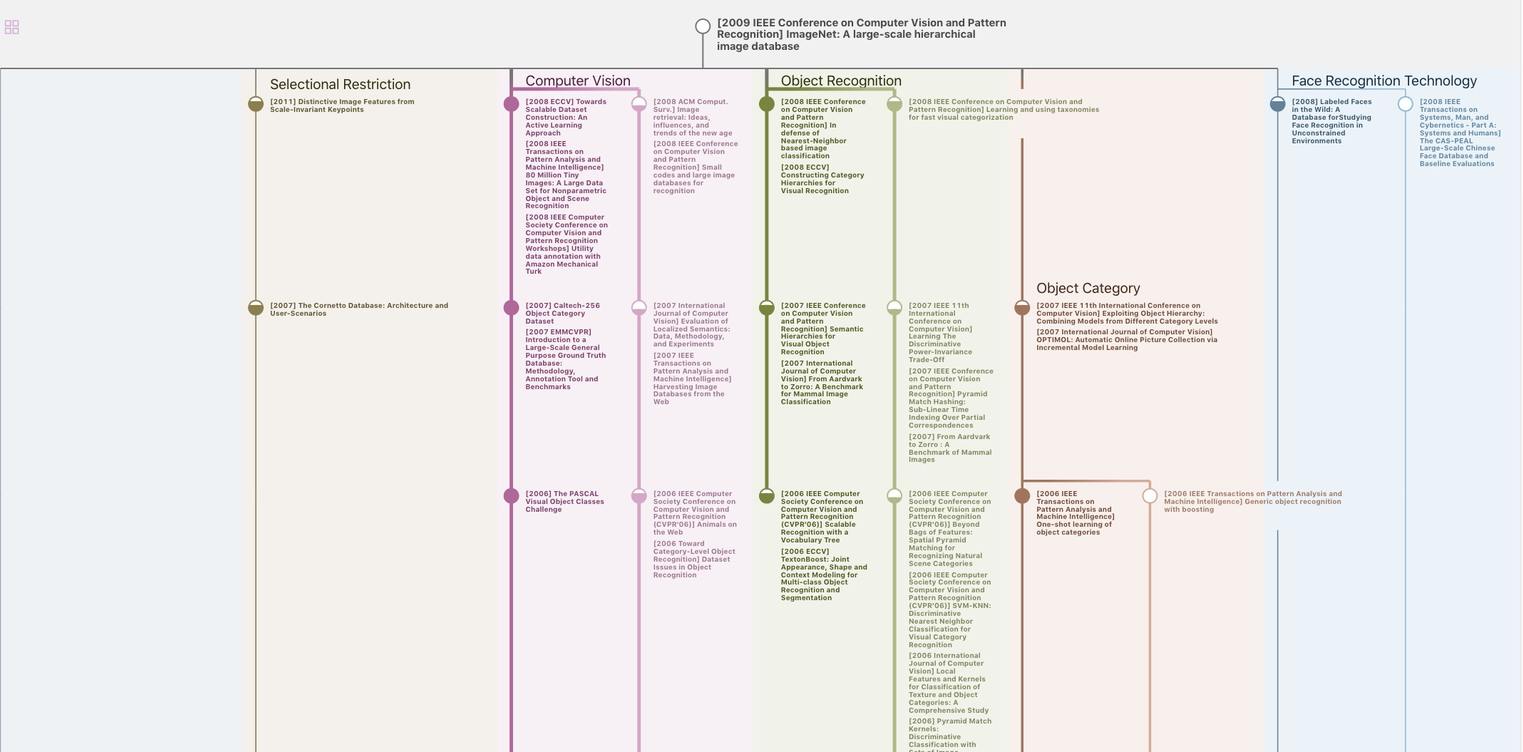
生成溯源树,研究论文发展脉络
Chat Paper
正在生成论文摘要