Neural Networks for Exact Constrained Optimization
ICANN(1996)
摘要
The paper is concerned with analog neural networks which can solve nonlinear constrained optimization tasks using the penalty function approach. The neural model developed can be regarded as asymptotically exact dynamic solver in a sense that the equilibrium state represents a solution which can be arbitrarily close to that of the original constrained optimization task. Although it is a quite natural requirement, generally it can be fulfilled only with arbitrarily large penalty multipliers. The neural network presented overcomes this problem with the use of special nonlinearities, that is it can produce solutions arbitrarily close to the exact one at finite penalty multipliers. Stability analyses validating the usefulness of the model are also outlined.
更多查看译文
关键词
exact constrained optimization,neural networks,constrained optimization,penalty function,neural network,equilibrium state
AI 理解论文
溯源树
样例
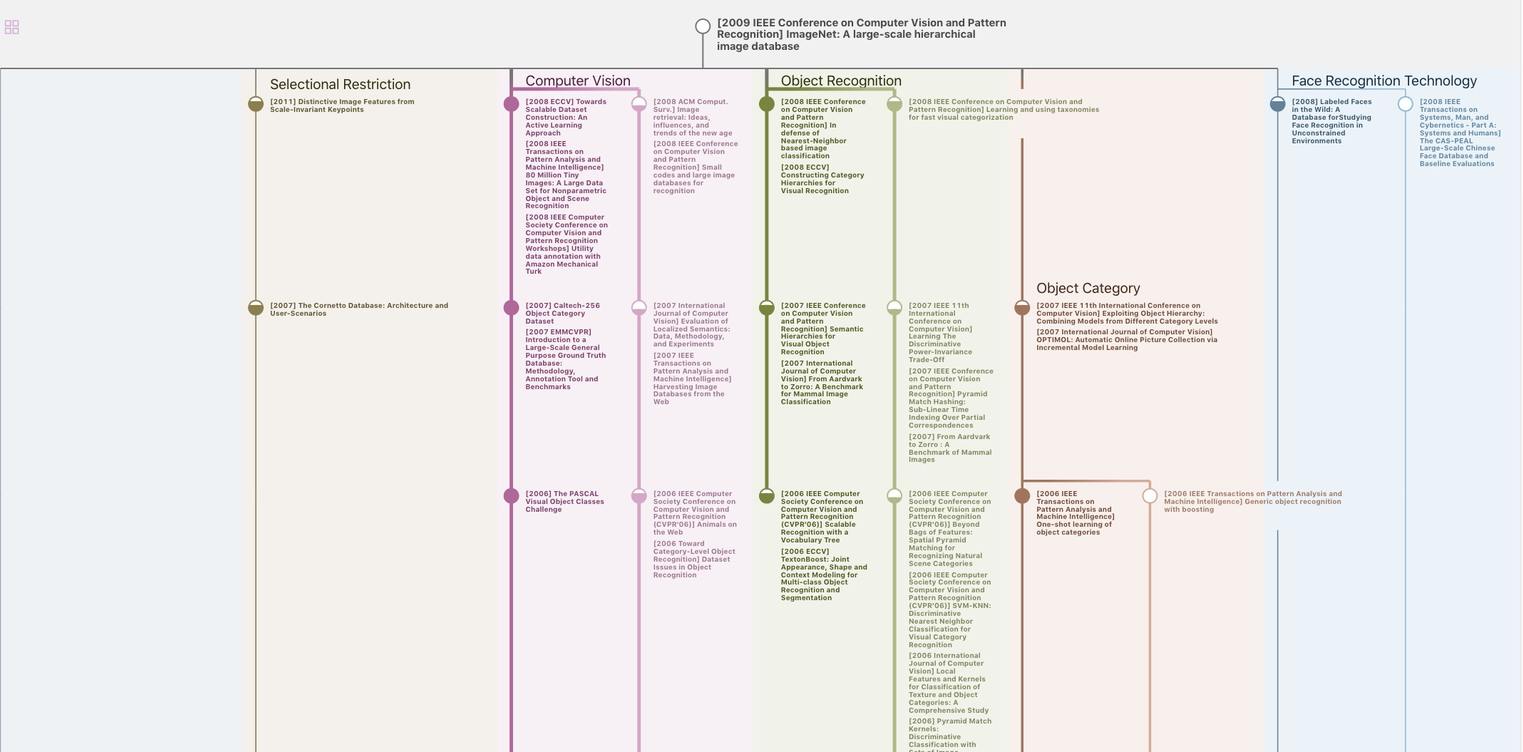
生成溯源树,研究论文发展脉络
Chat Paper
正在生成论文摘要