Feature Selection using a Genetic Algorithm applied on an Air Quality Forecasting Problem
Ai Communications(2003)
摘要
Feature selection is a process followed in order to improve the generalization and the performance of several classification and/or regression algorithms. Feature selection processes are divided in two categories, the filter and the wrapper approach. The formal is performed independently of the learning algorithm while the later makes use of the algorithm in an iterative way. As (1) describe, the feature weighting algorithms are divided into two categories: the filtering methods and the wrapper methods. The former is a no-feedback, pre-selection approach where the selection of the feature subset is performed independently of the learning algorithm. The later is an iterative method that encapsulates the learning algorithm in the feature selection process. This paper focuses on the exploitation of a genetic algorithm used to extract an optimal feature subset of a large database containing pollutant concentration measurements, following the wrapper approach. The feature subset feeds a nearest neighbor algorithm in order to predict the daily maximum concentration for two pollutants. The encoding problem of the complexity of representation of the features in the genomes is tackled. Results of the experimentation on an air quality forecasting problem will be presented, as well as slight alterations on the standard simple genetic algorithm paradigm that guided the algorithm to a mature convergence and gave good solutions.
更多查看译文
关键词
iteration method,feature selection,nearest neighbor,genetic algorithm
AI 理解论文
溯源树
样例
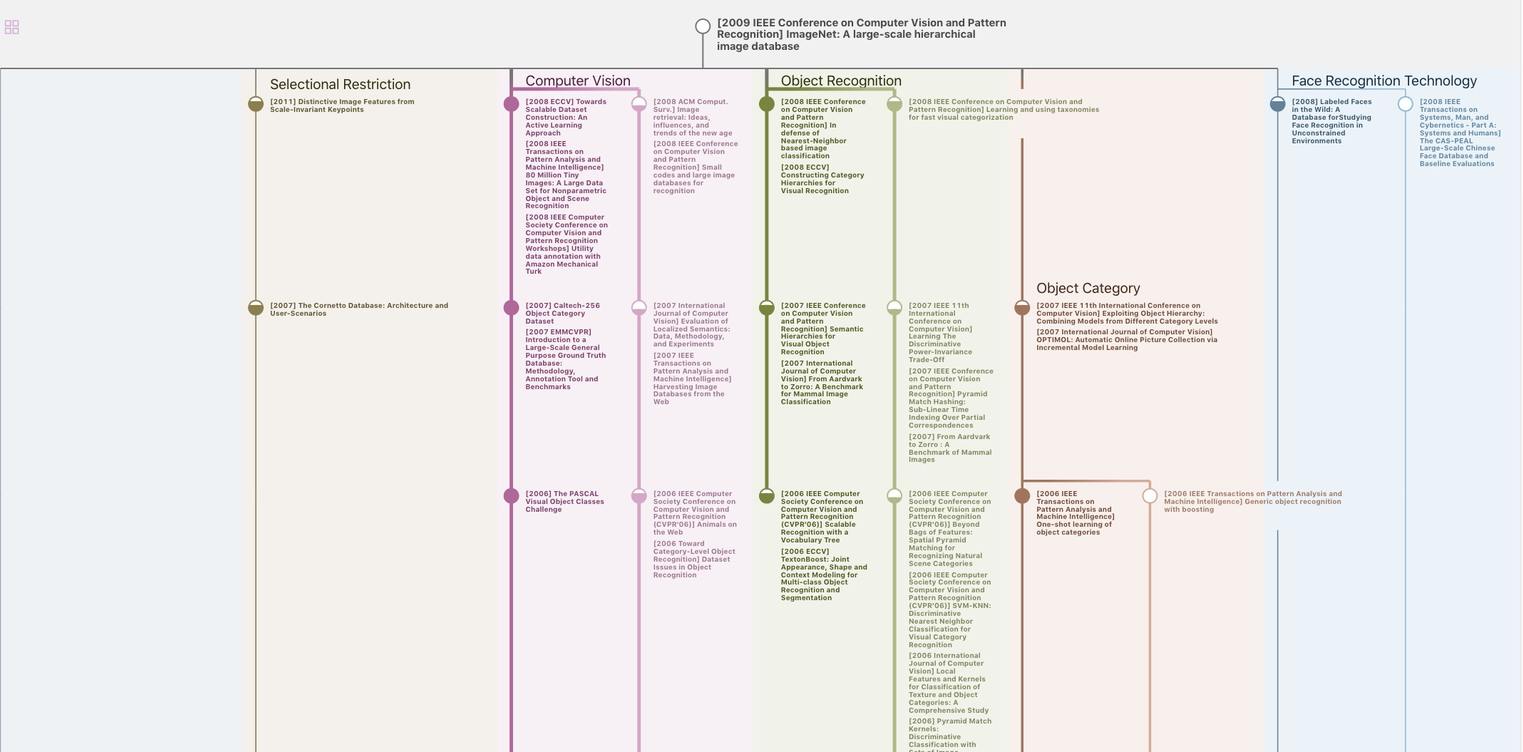
生成溯源树,研究论文发展脉络
Chat Paper
正在生成论文摘要