Anomaly Detection for an E-commerce Pricing System
KDD'19: PROCEEDINGS OF THE 25TH ACM SIGKDD INTERNATIONAL CONFERENCCE ON KNOWLEDGE DISCOVERY AND DATA MINING(2019)
摘要
Online retailers execute a very large number of price updates when compared to brick-and-mortar stores. Even a few mis-priced items can have a significant business impact and result in a loss of customer trust. Early detection of anomalies in an automated real-time fashion is an important part of such a pricing system. In this paper, we describe unsupervised and supervised anomaly detection approaches we developed and deployed for a large-scale online pricing system at Walmart. Our system detects anomalies both in batch and real-time streaming settings, and the items flagged are reviewed and actioned based on priority and business impact. We found that having the right architecture design was critical to facilitate model performance at scale, and business impact and speed were important factors influencing model selection, parameter choice, and prioritization in a production environment for a large-scale system. We conducted analyses on the performance of various approaches on a test set using real-world retail data and fully deployed our approach into production. We found that our approach was able to detect the most important anomalies with high precision.
更多查看译文
关键词
anomaly detection,e-commerce,pricing
AI 理解论文
溯源树
样例
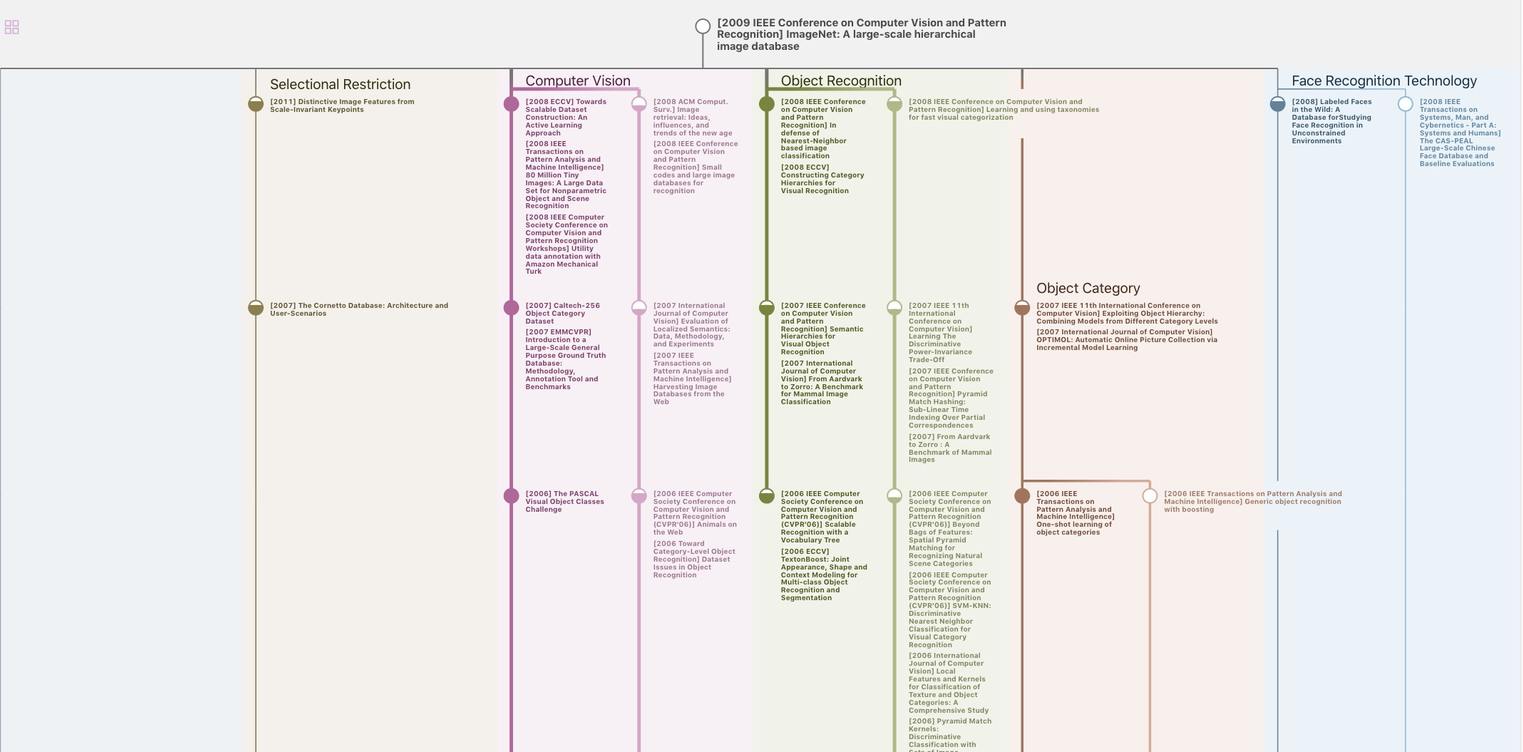
生成溯源树,研究论文发展脉络
Chat Paper
正在生成论文摘要