Time Context-Aware IPTV Program Recommendation Based on Tensor Learning.
IEEE Global Communications Conference(2018)
摘要
IPTV provides a variety of services to users, e.g., Live TV, Video on Demand (VOD), and Catch-up TV, which allows users to freely select from a huge pool of program genres. A high-quality program recommendation system that can well predict users' dynamic preferences is desirable to improve user satisfaction. In this paper, we make the first attempt to design a personalized TV program recommendation system based on tensor learning that leverages temporal context information and implicit feedback. We establish the user preference model by carefully analyzing the influence of program genres and time context on users' viewing behavior in IPTV viewing logs. To obtain the optimal program recommendation, we adopt tensor decomposition to mine the latent relationship among users, program genres and the time contexts, while address issues of data sparsity and missing information. Our extensive experimental results on a real dataset from a major IPTV service provider in China have confirmed the superiority of our proposed recommendation algorithm over baseline algorithms in achieving a higher recommendation accuracy and covering a wider range of programs.
更多查看译文
关键词
temporal context information,video-on-demand,VOD,users dynamic preferences,China,data sparsity,time context-aware IPTV program recommendation,recommendation algorithm,IPTV service provider,tensor decomposition,optimal program recommendation,IPTV viewing logs,user preference model,tensor learning,personalized TV program recommendation system,user satisfaction,high-quality program recommendation system,program genres,Catch-up TV,Live TV
AI 理解论文
溯源树
样例
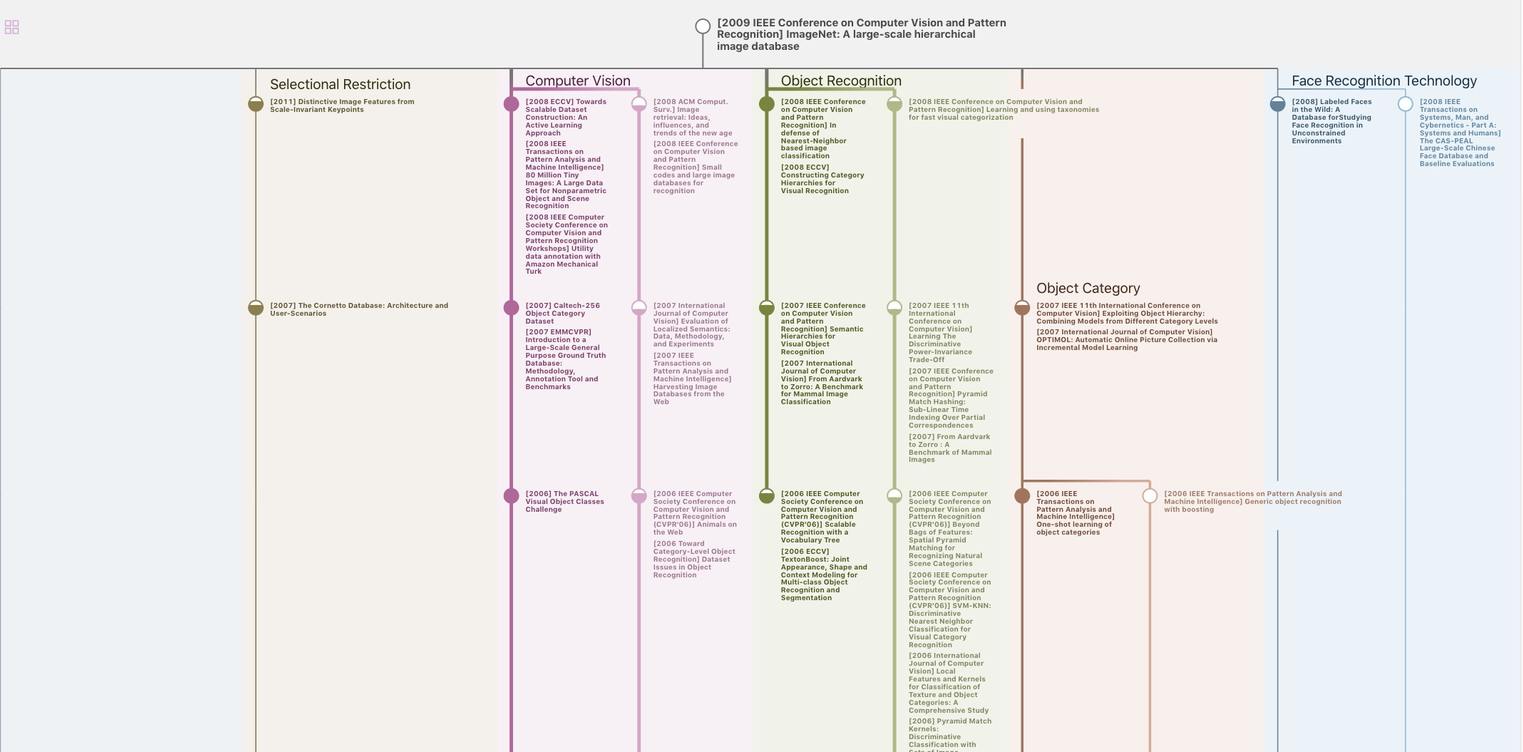
生成溯源树,研究论文发展脉络
Chat Paper
正在生成论文摘要