A weighted multiple-feature fusion classifier for hyperspectral images with limited training samples
EUROPEAN JOURNAL OF REMOTE SENSING(2018)
摘要
In this paper, a novel weighted multiple-feature classifier based on sparse representation and locally dictionary collaborative representation (WMSLC) is put forward to improve the limited training samples' hyperspectral image classification performance. The WMSLC method mainly includes the following steps. Firstly, Spectral feature, Extended Multi-attribute Profile (EMAP) feature and Gabor feature are applied as the multiple feature to describe the hyperspectral image from spectral and different spatial aspects. And weights are utilized to adjust the multiple-feature's proportions to improve the efficiency of the classification. Secondly, a trade-off is given between different regularization residuals, sparse representation residuals and locally dictionary collaborative representation residuals. Here, the locally adaptive dictionary is implemented to reduce the irrelevant atoms to improve the classification performance. Finally, the test sample is assigned to the class, which has the minimal residuals. Experimental results on two real hyperspectral data sets (Indian Pines and Pavia University) demonstrate that the proposed WMSLC method outperforms several corresponding wellknown classifiers when very limited numbers of training samples are available.
更多查看译文
关键词
Hyperspectral,classification,weighted multiple-feature,limited training samples,sparse representation,locally dictionary collaborative representation
AI 理解论文
溯源树
样例
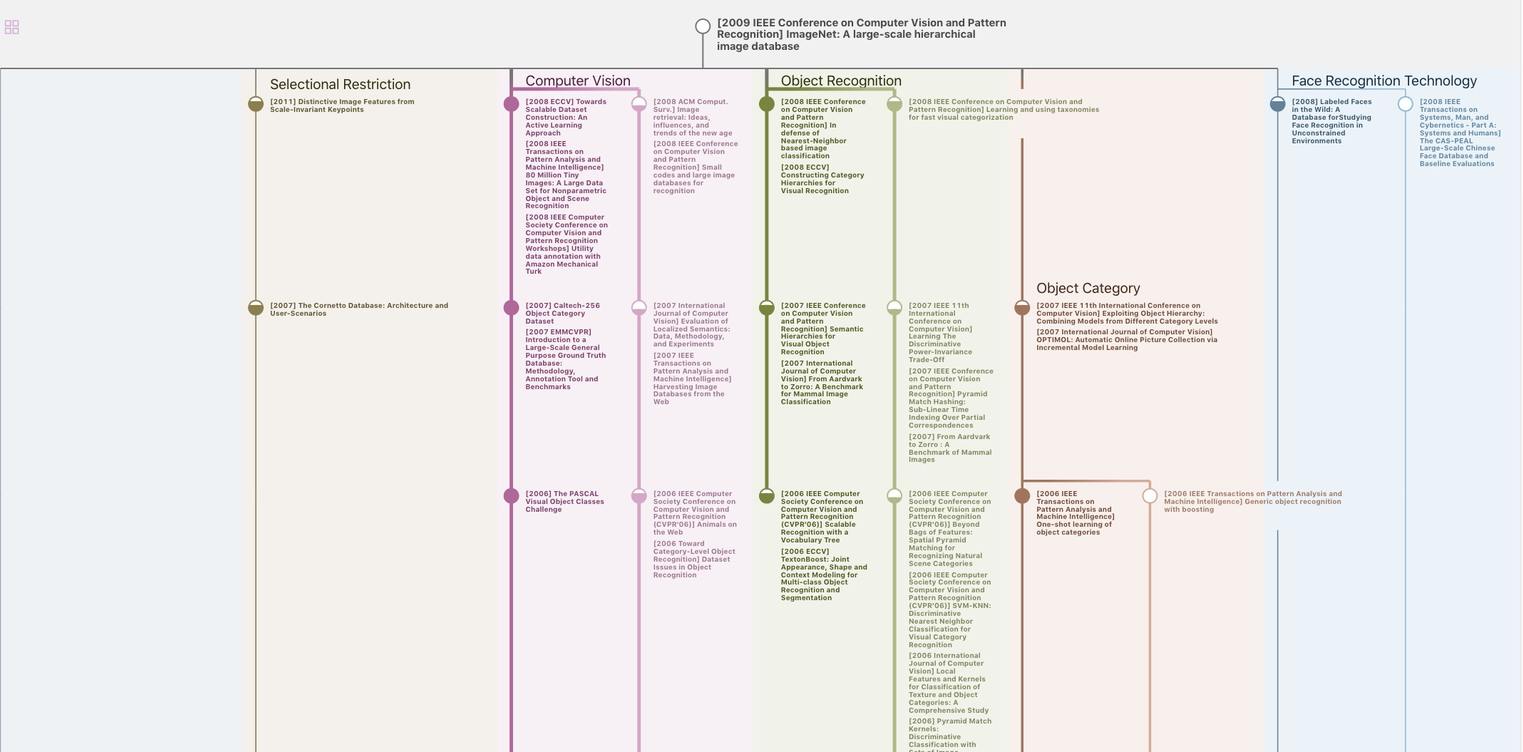
生成溯源树,研究论文发展脉络
Chat Paper
正在生成论文摘要