Anti-Noise Twin-Hyperspheres with Density Fuzzy for Binary Classification to Imbalanced Data with Noise
SSRN Electronic Journal(2023)
摘要
Abstract This paper presents twin-hyperspheres of resisting noise for binary classification to imbalanced data with noise. First, employing the decision of evaluating the contributions created by points for the training of the hyperspheres, then the label density estimator is introduced into the fuzzy membership to quantize the provided contributions, and finally, unknown points can be assigned into corresponding classes. Utilizing the decision, the interference created by the noise hidden in the data is suppressed. Experiment results show that when noise ratio reaches 90%, classification accuracies of the model are 0.802, 0.611 on the synthetic datasets and UCI datasets containing Gaussian noise, respectively. Classification results of the model outperform these of the competitors, and these boundaries learned by the model to separate noise from majority classes and minority classes are superior to these learned by the competitors. Moreover, efforts gained by the proposed density fuzzy are effectiveness in noise resistance; meanwhile, the density fuzzy does not rely on specific classifiers or specific scenarios.
更多查看译文
关键词
Binary classification,Density fuzzy,Hyperspheres,Imbalanced data,Noise
AI 理解论文
溯源树
样例
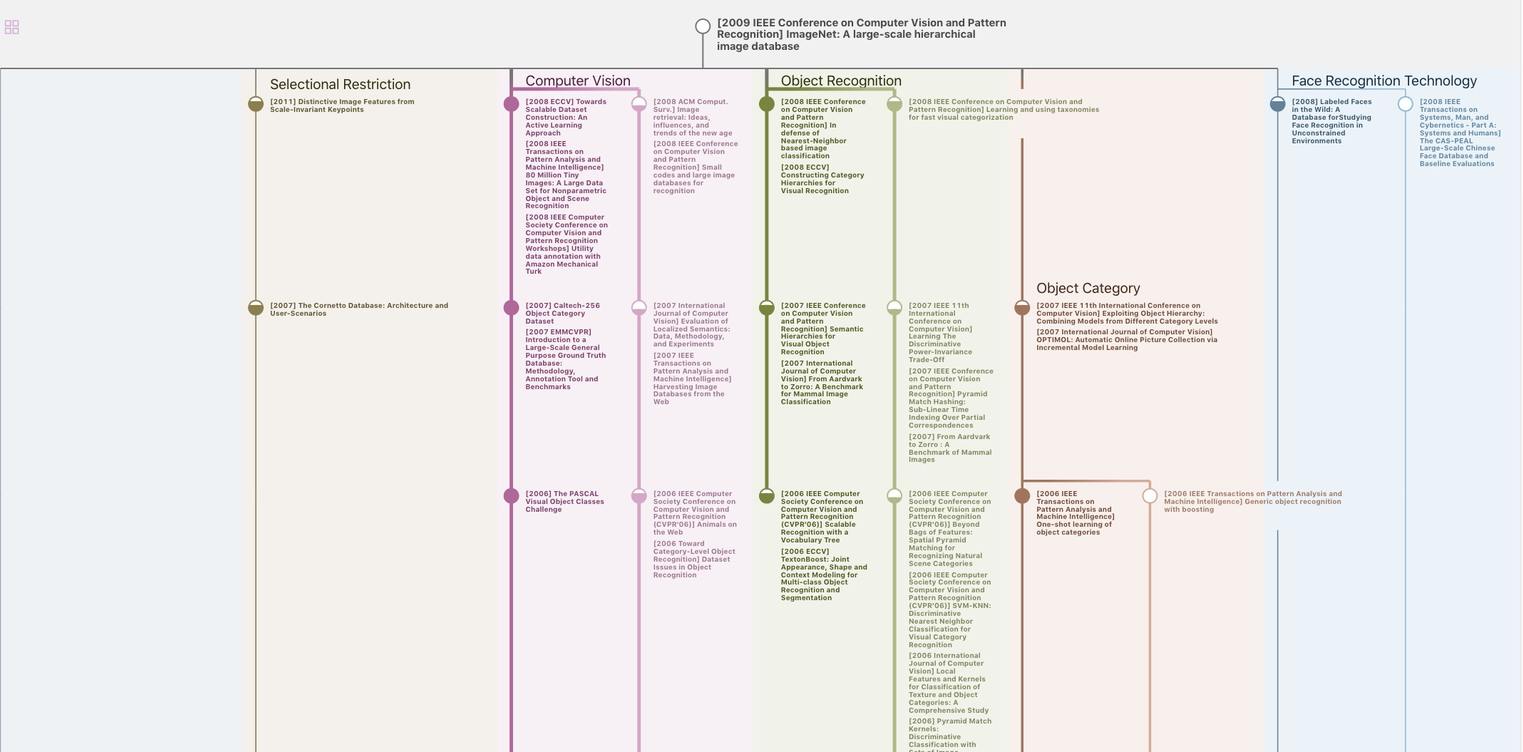
生成溯源树,研究论文发展脉络
Chat Paper
正在生成论文摘要