Ground-Based Cloud-Type Recognition Using Manifold Kernel Sparse Coding and Dictionary Learning
ADVANCES IN METEOROLOGY(2018)
摘要
Recognizing cloud type of ground-based images automatically has a great influence on the weather service but poses a significant challenge. Based on the symmetric positive definite (SPD) matrix manifold, a novel method named "manifold kernel sparse coding and dictionary learning" (MKSCDL) is proposed for cloud classification. Different from classical features extracted in the Euclidean space, the SPD matrix fuses multiple features and represents non-Euclidean geometric characteristics. MKSCDL is composed of three steps: feature extraction, dictionary learning, and classification. With the learned dictionary, the SPD matrix of the cloud image can be described with the sparse code. The experiments are conducted on two different ground-based cloud image datasets. Benefitting from the sparse representation on the Riemannian matrix manifold, compared to the recent baselines, experimental results demonstrate that MKSCDL possesses a more competitive performance on both grayscale and colour image datasets.
更多查看译文
关键词
manifold kernel sparse coding,recognition,ground-based,cloud-type
AI 理解论文
溯源树
样例
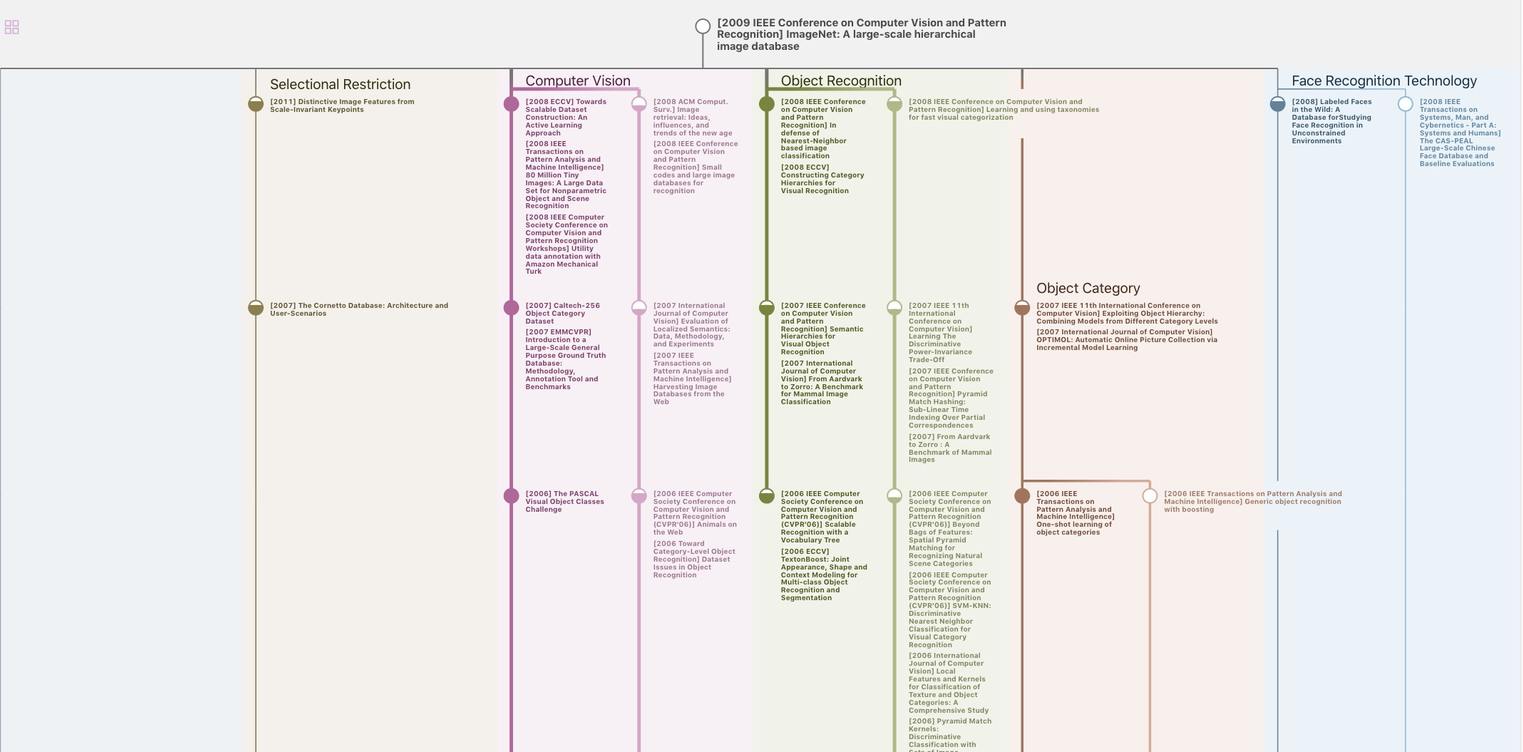
生成溯源树,研究论文发展脉络
Chat Paper
正在生成论文摘要