Abstract 19246: Histology-Validated Neural Networks Enable Accurate Plaque Tissue and Thin-Capped Fibroatheroma Characterization Through Intravascular Optical Coherence Tomography
Circulation(2016)
摘要
Introduction: Although intravascular ultrasound can successfully classify plaque composition in an automated fashion through ultrasound virtual histology, this has not been accomplished with intravascular optical coherence tomography (IVOCT). The complexity of IVOCT images has limited its clinical acceptance despite its greater resolution. This is particularly true with thin-capped fibroatheroma (TCFA), which are uniquely recognized by IVOCT, but prone to be falsely identified by expert viewers. Hypothesis: We assessed the hypothesis that histology validated supervised neural networks can reliably characterize arterial tissue and TCFA in IVOCT images. Methods: Neural network features and nodes were optimized to best classify arterial fibrous, calcium and lipid tissue in IVOCT images. Grey level co-occurrence matrix and windowed texture analysis was used to develop network features. Lipid pixels were used to isolate TCFA. The network was trained on 81000 pixels and tested on 61000 pixels, sampled separatel...
更多查看译文
AI 理解论文
溯源树
样例
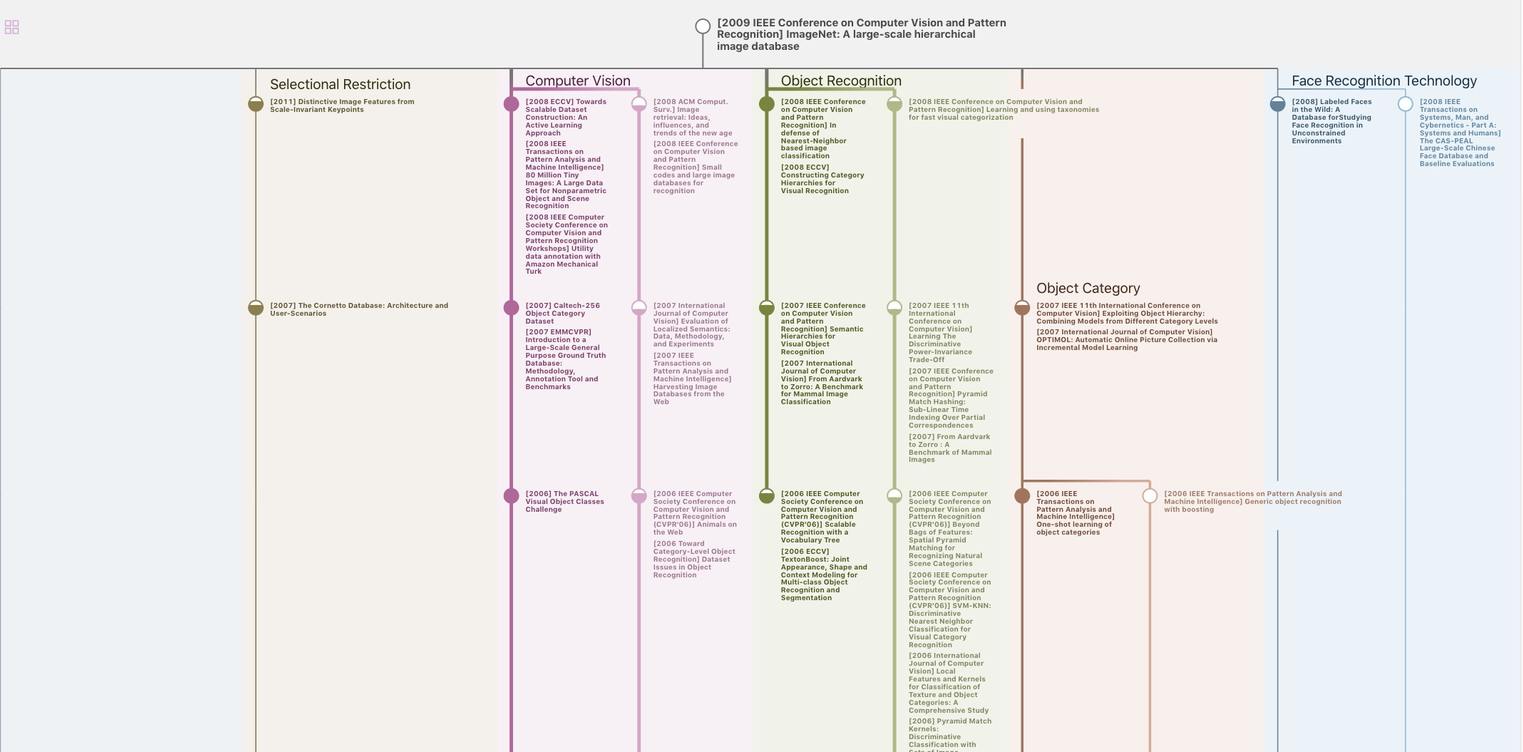
生成溯源树,研究论文发展脉络
Chat Paper
正在生成论文摘要