Granular object morphological generation with genetic algorithms for discrete element simulations
Granular Matter(2018)
摘要
The Discrete Element Method is a popular method for modeling granular materials, however, it is typically limited to geometrically simple objects. A recent extension of this method, the Level Set Discrete Element Method (LS-DEM), overcomes this issue by allowing the use of any particle shape, including morphologically accurate computational grains generated from tomographic images. This method has the ability to provide insight into the physics of granular media that are challenging if granular shape morphology is not accurately represented. One challenge with fully utilizing LS-DEM is gathering the data necessary to reproduce the distinct shapes of grains. In this work, we develop a novel granular generation method that uses genetic algorithms to create new computational grains from a smaller set of input data. This method has the capability of building grains that match any well defined morphological property. We demonstrate the method by generating grains to match sphericity and principal curvature property distributions generated from an existing particle dataset captured with 3D X-Ray tomography.
更多查看译文
关键词
Shape morphology,Genetic algorithms,Granular objects
AI 理解论文
溯源树
样例
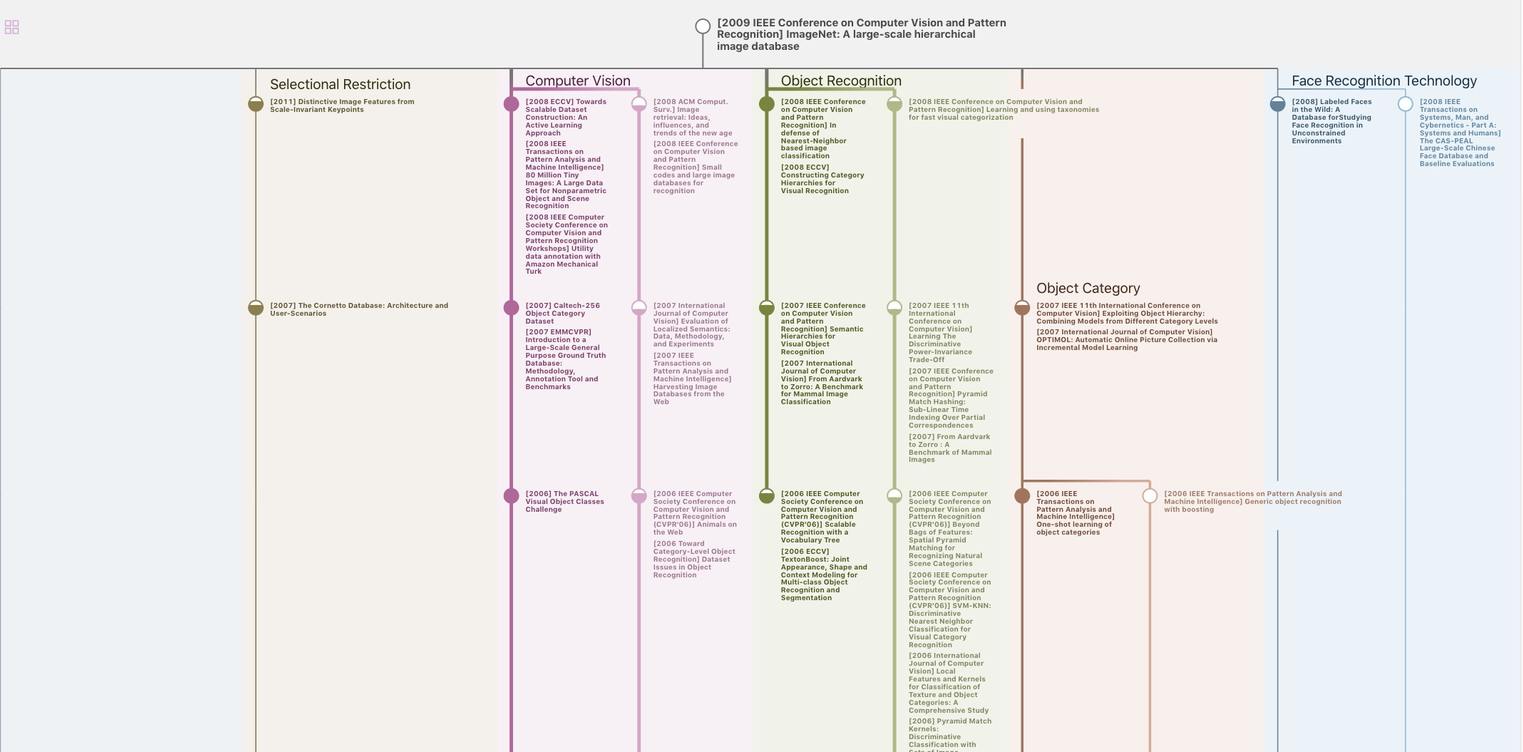
生成溯源树,研究论文发展脉络
Chat Paper
正在生成论文摘要