Semantic Segmentation Of Aerial Imagery Via Multi-Scale Shuffling Convolutional Neural Networks With Deep Supervision
ISPRS TC I MID-TERM SYMPOSIUM INNOVATIVE SENSING - FROM SENSORS TO METHODS AND APPLICATIONS(2018)
摘要
In this paper, we address the semantic segmentation of aerial imagery based on the use of multi-modal data given in the form of true orthophotos and the corresponding Digital Surface Models (DSMs). We present the Deeply-supervised Shuffling Convolutional Neural Network (DSCNN) representing a multi-scale extension of the Shuffling Convolutional Neural Network (SCNN) with deep supervision. Thereby, we take the advantage of the SCNN involving the shuffling operator to effectively upsample feature maps and then fuse multiscale features derived from the intermediate layers of the SCNN, which results in the Multi-scale Shuffling Convolutional Neural Network (MSCNN). Based on the MSCNN, we derive the DSCNN by introducing additional losses into the intermediate layers of the MSCNN. In addition, we investigate the impact of using different sets of hand-crafted radiometric and geometric features derived from the true orthophotos and the DSMs on the semantic segmentation task. For performance evaluation, we use a commonly used benchmark dataset. The achieved results reveal that both multi-scale fusion and deep supervision contribute to an improvement in performance. Furthermore, the use of a diversity of hand-crafted radiometric and geometric features as input for the DSCNN does not provide the best numerical results, but smoother and improved detections for several objects.
更多查看译文
关键词
Semantic Segmentation, Aerial Imagery, Multi-Modal Data, Multi-Scale, CNN, Deep Supervision
AI 理解论文
溯源树
样例
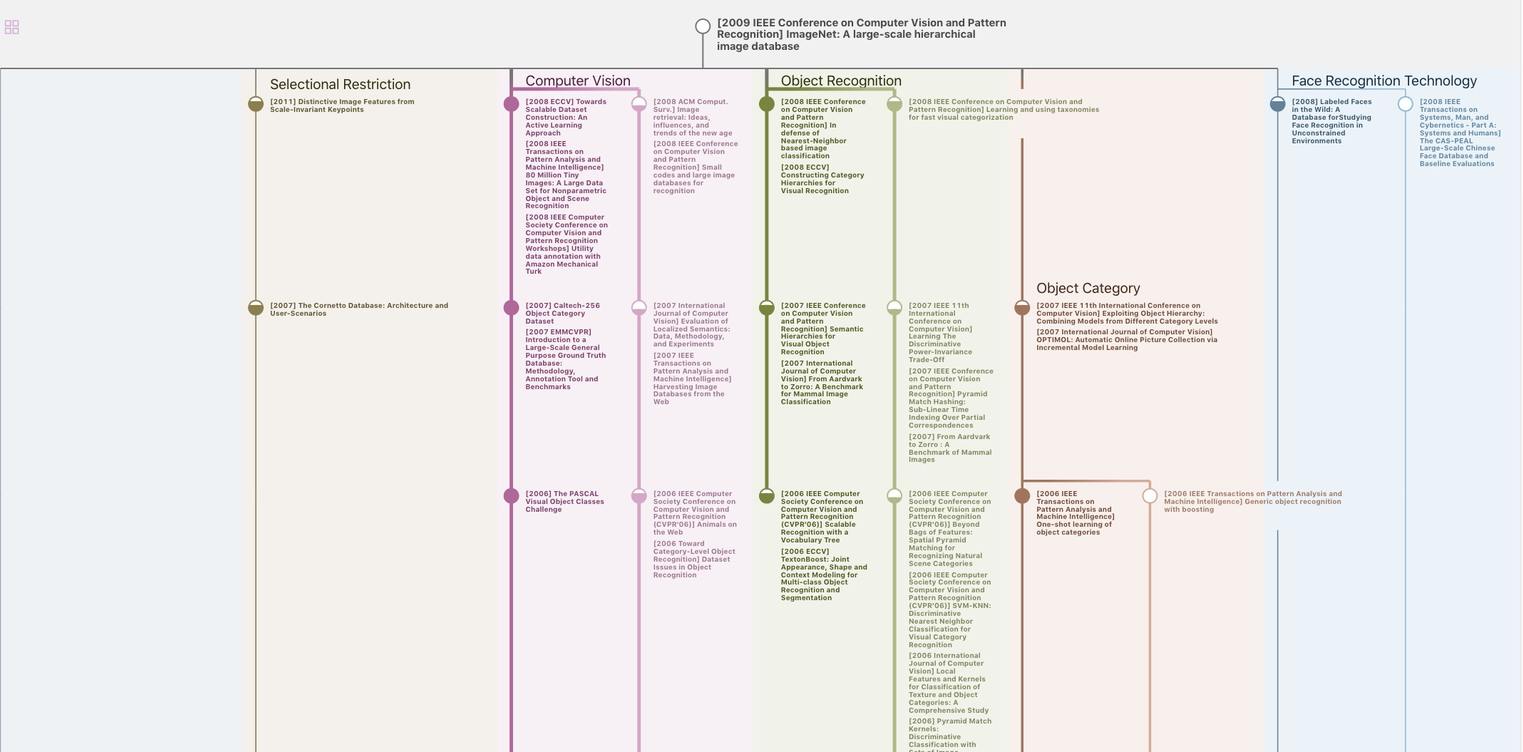
生成溯源树,研究论文发展脉络
Chat Paper
正在生成论文摘要