Robustness in the Optimization of Risk Measures
OPERATIONS RESEARCH(2022)
摘要
We study issues of robustness in the context of Quantitative Risk Management and Optimization. We develop a general methodology for determining whether a given risk-measurement-related optimization problem is robust, which we call "robustness against optimization." The new notion is studied for various classes of risk measures and expected utility and loss functions. Motivated by practical issues from financial regulation, special attention is given to the two most widely used risk measures in the industry, Value-at-Risk (VaR) and Expected Shortfall (ES). We establish that for a class of general optimization problems, VaR leads to nonrobust optimizers, whereas convex risk measures generally lead to robust ones. Our results offer extra insight on the ongoing discussion about the comparative advantages of VaR and ES in banking and insurance regulation. Our notion of robustness is conceptually different from the field of robust optimization, to which some interesting links are derived.
更多查看译文
关键词
robustness, value-at-risk, expected shortfall, optimization, financial regulation
AI 理解论文
溯源树
样例
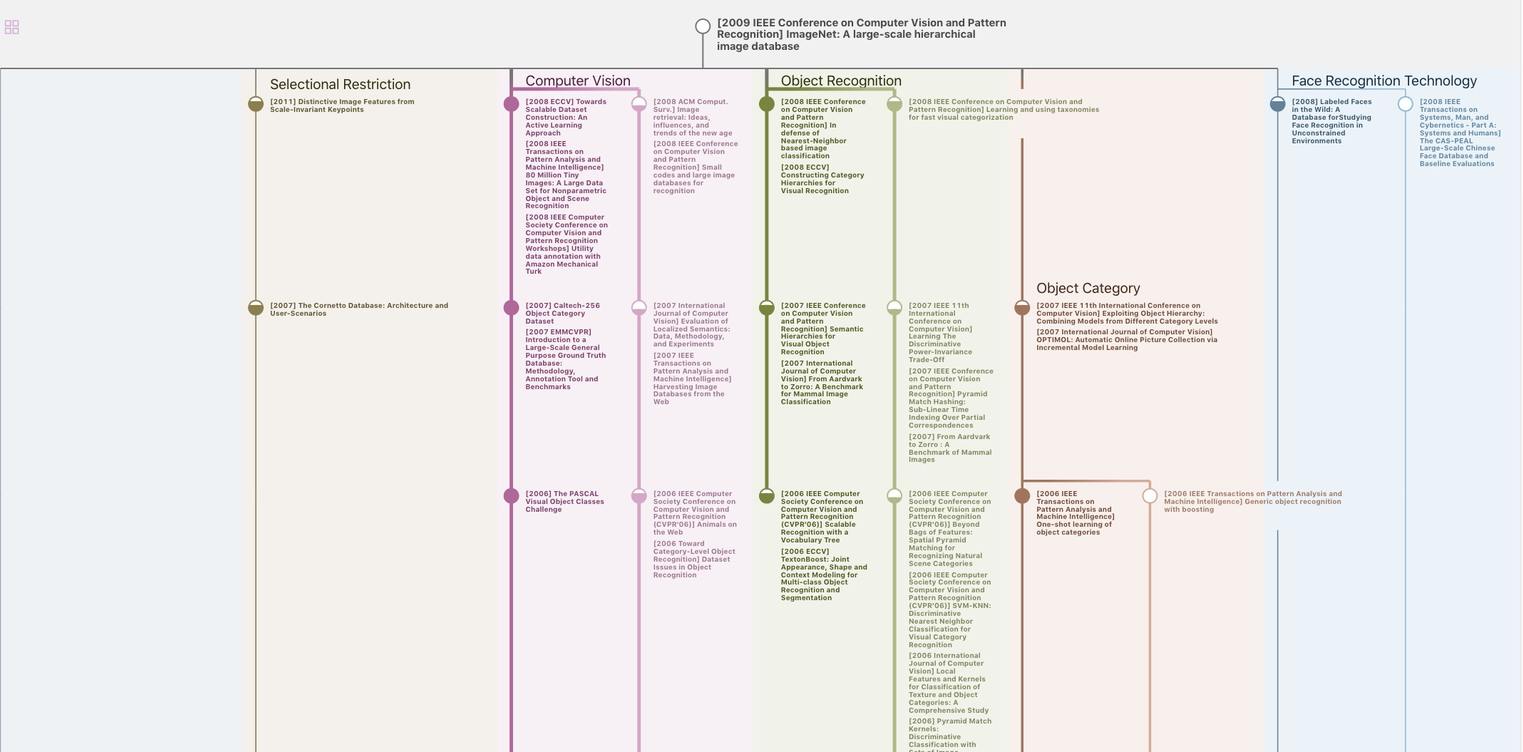
生成溯源树,研究论文发展脉络
Chat Paper
正在生成论文摘要