Nonnegative Matrix Factorization Regularized With Trust Relationships For Solving Cold-Start Problem In Recommender Systems
26TH IRANIAN CONFERENCE ON ELECTRICAL ENGINEERING (ICEE 2018)(2018)
摘要
Matrix factorization has shown as an effective collaborative filtering approach to build a successful recommender system. However, these systems have poor performance while facing cold -start users (items). To tackle this issue, in this paper, a social regularization method called TrustANLF is proposed which combines the social network information of users in a nonnegative matrix factorization framework. The proposed method integrates multiple information sources such as user -item ratings and trust statements to reduce the cold -start and data sparsity issues. Moreover, the alternating direction method is used to improve the convergence speed and reduce the computational cost. To evaluate the proposed method, several experiments are performed on two real -world datasets. The results report the effectiveness of the proposed method compared to several stateof-the-art methods.
更多查看译文
关键词
Recommender systems, Matrix factorization, Social trust, Alternating direction method, Collaborative filtering
AI 理解论文
溯源树
样例
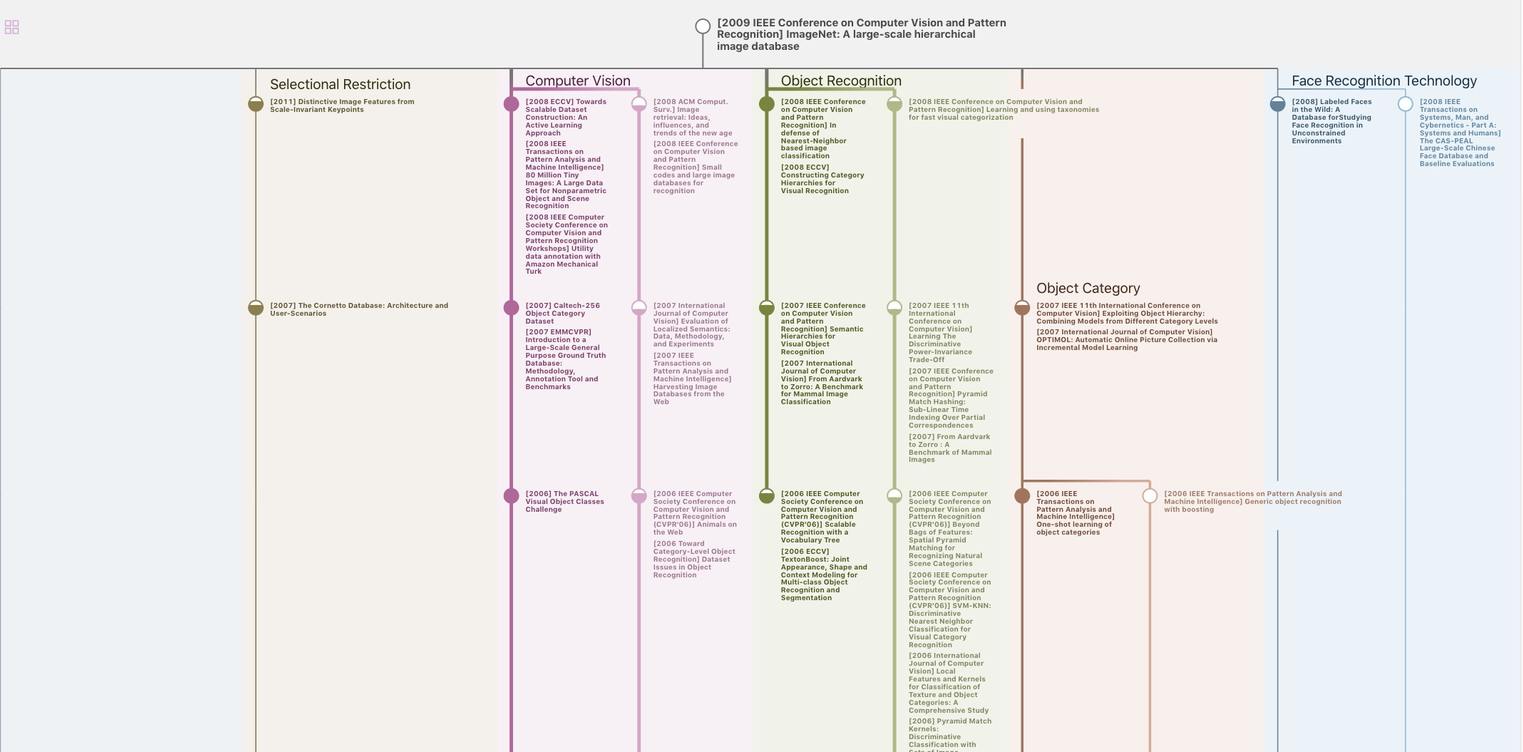
生成溯源树,研究论文发展脉络
Chat Paper
正在生成论文摘要