Optimized Bp Neural Network For Dissolved Oxygen Prediction
IFAC PAPERSONLINE(2018)
摘要
To solve the low accuracy, slow convergence and poor robustness problem of traditional neural network method for water quality forecasting, a new model of dissolved oxygen content prediction is proposed based on sliding window, particle swarm optimization (PSO) and BP neural network. dissolved oxygen content prediction model in water quality is established by handling dissolved oxygen content data through sliding window, and using particle swarm optimization algorithm to obtain BP neural network parameters. This model is applied to prediction analysis of dissolved oxygen with online monitoring of regional groundwater in Xilin Gol League on July 25, 2017 to December 5, 2017. Experimental results show that the model has better prediction effect, and mean square error (MSE), root mean square error(RMSE), mean absolute error(MAE) value of PSO algorithm to optimize the BP neural network based on sliding window are 0.437% and 6.611%, 0.251% respectively, which are better than single forecasting method by using sliding window, PSO, and BP neural network individually. The Optimized BP neural network not only has fast convergence speed and high prediction accuracy, but also provides decision-making basis for water pollution control and water management. (C) 2018, IFAC (International Federation of Automatic Control) Hosting by Elsevier Ltd. All rights reserved.
更多查看译文
关键词
water quality prediction, sliding window, particle swarm optimization, BP neural network
AI 理解论文
溯源树
样例
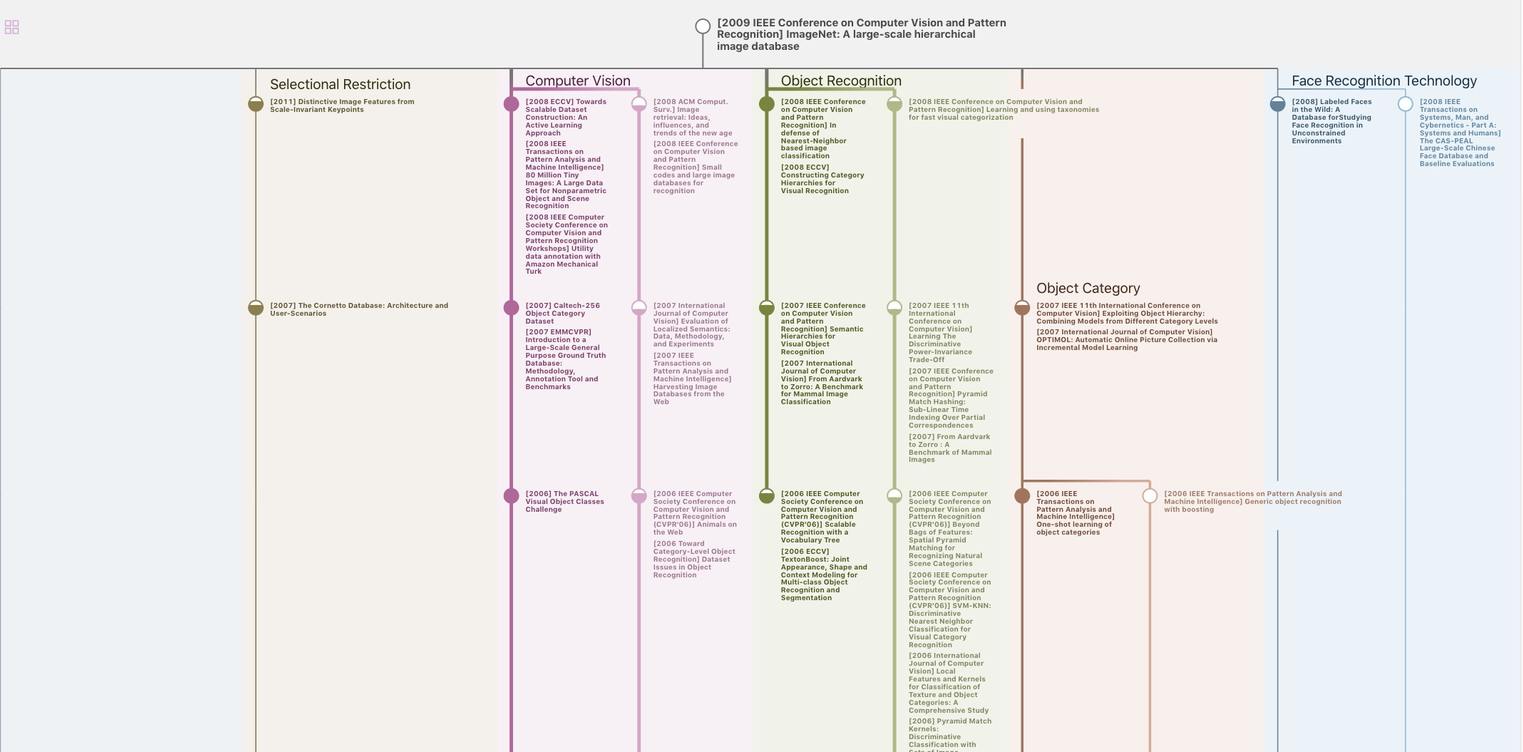
生成溯源树,研究论文发展脉络
Chat Paper
正在生成论文摘要