Simultaneous multiple low-dimensional subspace dimensionality reduction and classification
International Journal of Computational Science and Engineering(2019)
摘要
Fisher linear discriminant (FLD) for supervised learning has recently emerged as a computationally powerful tool for extracting features for a variety of pattern classification problems. However, it works poorly with multimodal data. Local Fisher linear discriminant (LFLD) is proposed to reduce the dimensionality of multimodal data. Through experiments tried out on the multimodal but binary datasets created from several multi-class datasets, it has been shown to be better than FLD in favour of performance. However, LFLD has a serious limitation, which is that it is limited to the use on small-scale datasets. In order to address the above disadvantages, in this paper, we develop a multiple low-dimensionality dimensionality reduction technique (MSDR) of performing the dimensionality reduction (DR) of input data. In contrast to FLD and LFLD finding an optimal low-dimensional subspace, the new algorithm attempts to seek multiple optimal low-dimensional subspaces that best make the data sharing the same labels more compact. Inheriting the advantages of NC, MSDR reduces the dimensionality of data and directly performs classification tasks without needing to train the model. Experiments of comparing MSDR with the existing traditional approaches tried out on UCI, show the effectiveness and efficiency of MSDR.
更多查看译文
关键词
Fisher linear discriminant, FLD, local Fisher linear discriminant, LFLD, dimensionality reduction, multiple low-dimensional subspaces
AI 理解论文
溯源树
样例
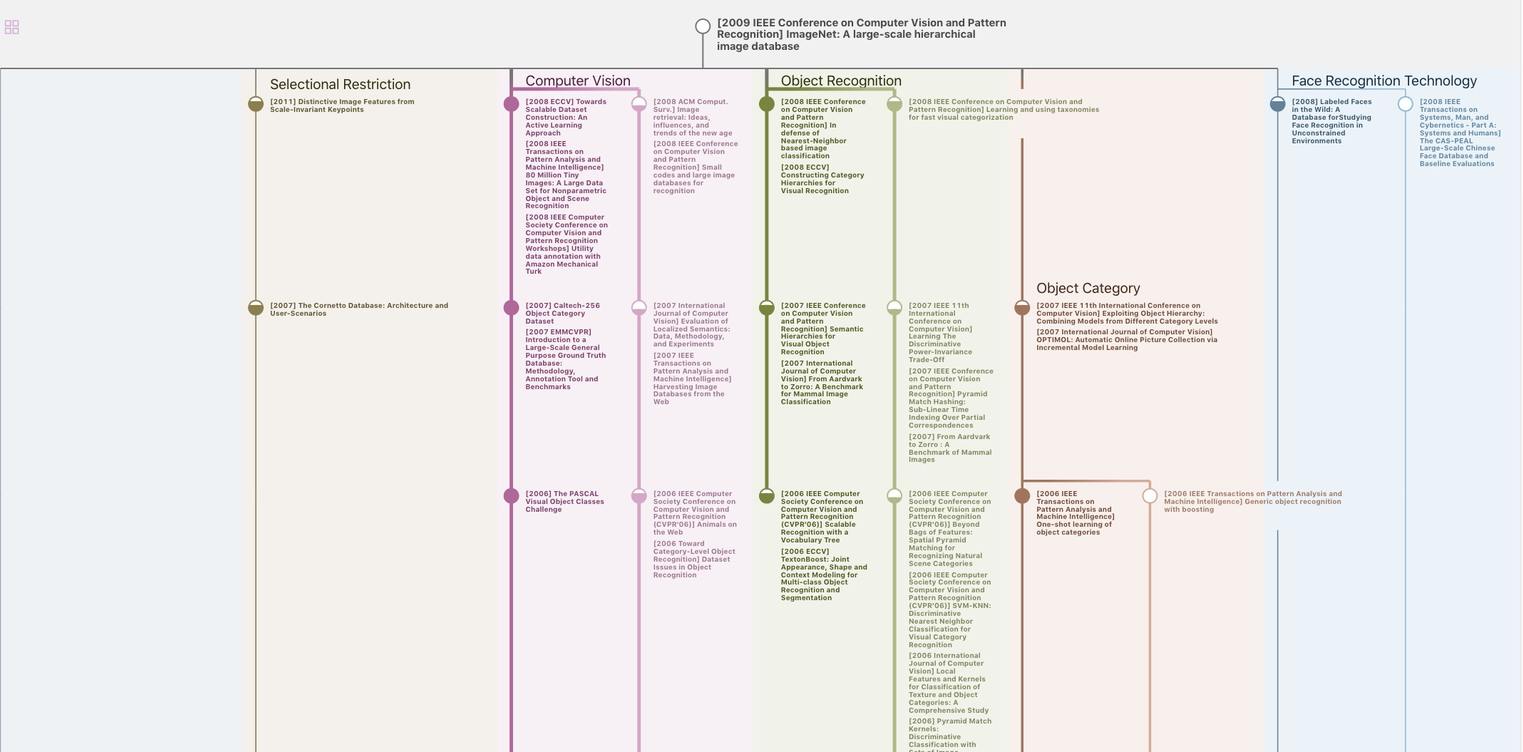
生成溯源树,研究论文发展脉络
Chat Paper
正在生成论文摘要