Robust Ship Tracking via Multi-view Learning and Sparse Representation
JOURNAL OF NAVIGATION(2019)
摘要
Conventional visual ship tracking methods employ single and shallow features for the ship tracking task, which may fail when a ship presents a different appearance and shape in maritime surveillance videos. To overcome this difficulty, we propose to employ a multi-view learning algorithm to extract a highly coupled and robust ship descriptor from multiple distinct ship feature sets. First, we explore multiple distinct ship feature sets consisting of a Laplacian-of-Gaussian (LoG) descriptor, a Local Binary Patterns (LBP) descriptor, a Gabor filter, a Histogram of Oriented Gradients (HOG) descriptor and a Canny descriptor, which present geometry structure, texture and contour information, and more. Then, we propose a framework for integrating a multi-view learning algorithm and a sparse representation method to track ships efficiently and effectively. Finally, our framework is evaluated in four typical maritime surveillance scenarios. The experimental results show that the proposed framework outperforms the conventional and typical ship tracking methods.
更多查看译文
关键词
Ship tracking,Multi-view learning,Sparse representation,Smart ship
AI 理解论文
溯源树
样例
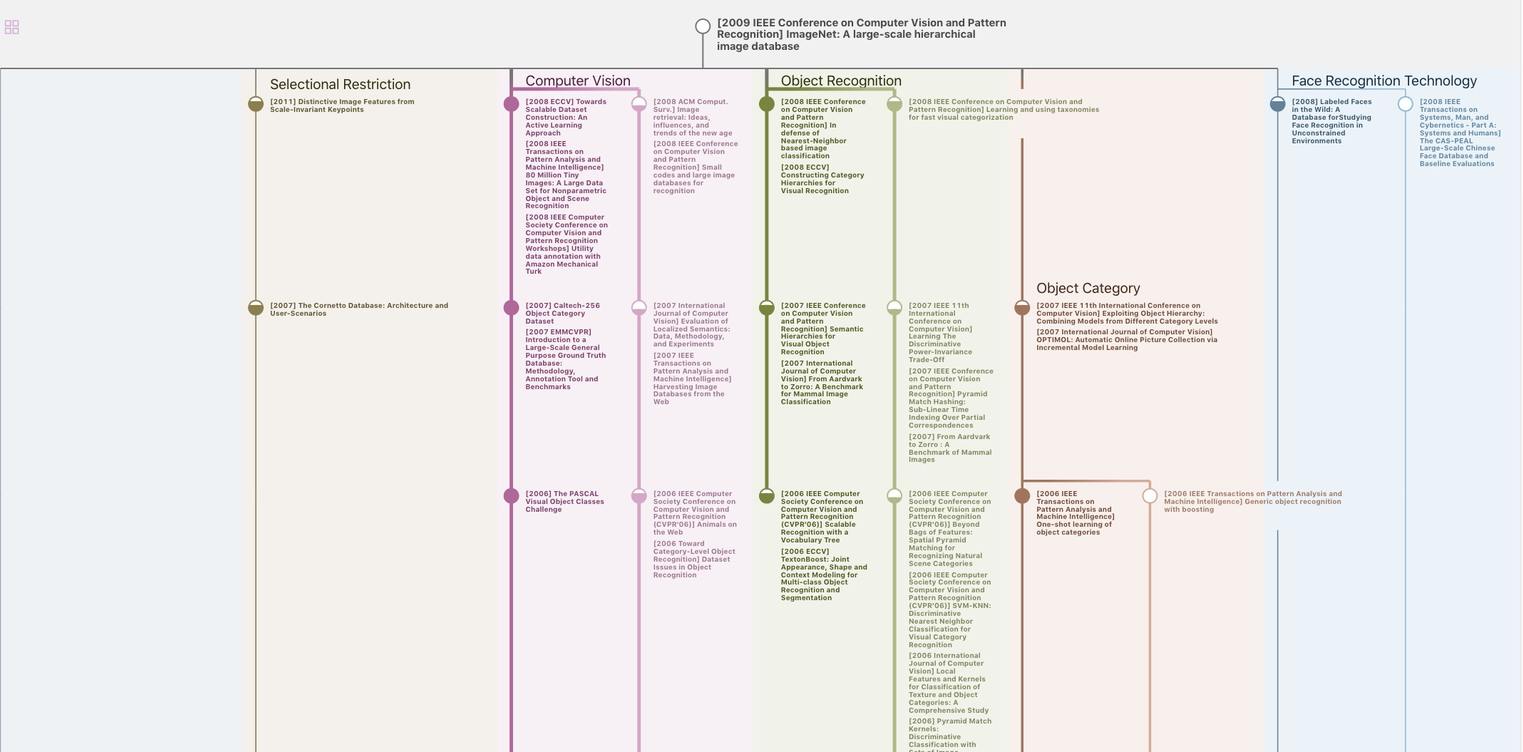
生成溯源树,研究论文发展脉络
Chat Paper
正在生成论文摘要