Pagoda: A Hybrid Approach to Enable Efficient Real-time Provenance Based Intrusion Detection in Big Data Environments
IEEE Transactions on Dependable and Secure Computing(2020)
摘要
Efficient intrusion detection and analysis of the new security threat in big data environments has gradually become a main challenge for todayu0027s personal or enterprisesu0027 users. As the intrusion behavior can be described by provenance graphs that record the dependency relationships between intrusion processes and the infected files, the existing intrusion detection methods typically analyze and identify the anomaly either in a single provenance path or the whole provenance graph, neither of which can achieve the benefit on both detection accuracy and detection time. We propose Pagoda, a hybrid approach that takes into account the anomaly degree of both a single provenance path and the whole provenance graph. It can identify intrusion quickly if a serious compromise has been found on one path, and can further improve detection rate by considering the behavior representation in the whole provenance graph. Pagoda uses a persistent memory database to store provenance and aggregates multiple similar items into one provenance record to maximumly reduce unnecessary I/O during the detection analysis. In addition, it encodes duplicate items in the rule database and filters noise that is not related to intrusion. The experimental results on a wide variety of real-world applications demonstrate its performance and efficiency.
更多查看译文
关键词
Provenance,intrusion detection,big data,real-time
AI 理解论文
溯源树
样例
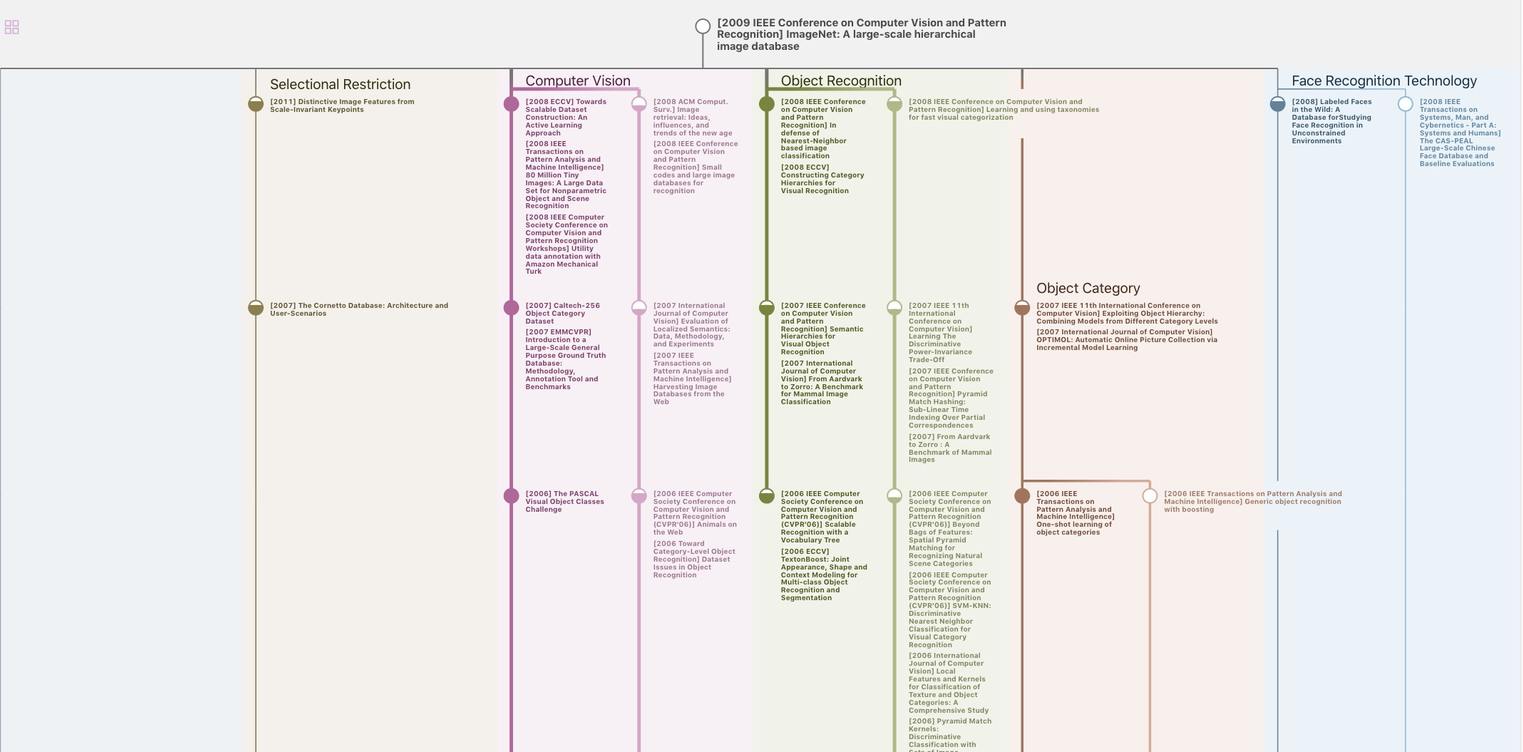
生成溯源树,研究论文发展脉络
Chat Paper
正在生成论文摘要