Statistical inference on asymptotic properties of two estimators for the partially linear single-index models
STATISTICS(2018)
摘要
The outer product of gradients (OPG) estimation procedure based on least squares (LS) approach has been presented by Xia et al. [An adaptive estimation of dimension reduction space. J Roy Statist Soc Ser B. 2002; 64: 363-410] to estimate the single-index parameter in partially linear single-index models (PLSIM). However, its asymptotic property has not been established yet and the efficiency of LS-based method can be significantly affected by outliers and heavy-tailed distributions. In this paper, we firstly derive the asymptotic property of OPG estimator developed by Xia et al. [An adaptive estimation of dimension reduction space. J Roy Statist Soc Ser B. 2002; 64: 363-410] in theory, and a novel robust estimation procedure combining the ideas ofOPGand local rank (LR) inference is further developed for PLSIM along with its theoretical property. Then, we theoretically derive the asymptotic relative efficiency (ARE) of the proposed LR-based procedure with respect to LS-based method, which is shown to possess an expression that is closely related to that of the signed-rank Wilcoxon test in comparison with the t-test. Moreover, we demonstrate that the new proposed estimator has a great efficiency gain across a wide spectrum of non-normal error distributions and almost not lose any efficiency for the normal error. Even in the worst case scenarios, the ARE owns a lower bound equalling to 0.864 for estimating the single-index parameter and a lower bound being 0.8896 for estimating the nonparametric function respectively, versus the LS-based estimators. Finally, some Monte Carlo simulations and a real data analysis are conducted to illustrate the finite sample performance of the estimators.
更多查看译文
关键词
Partially linear single-index models,outer product of gradients,local rank regression,asymptotic property,asymptotic relative efficiency
AI 理解论文
溯源树
样例
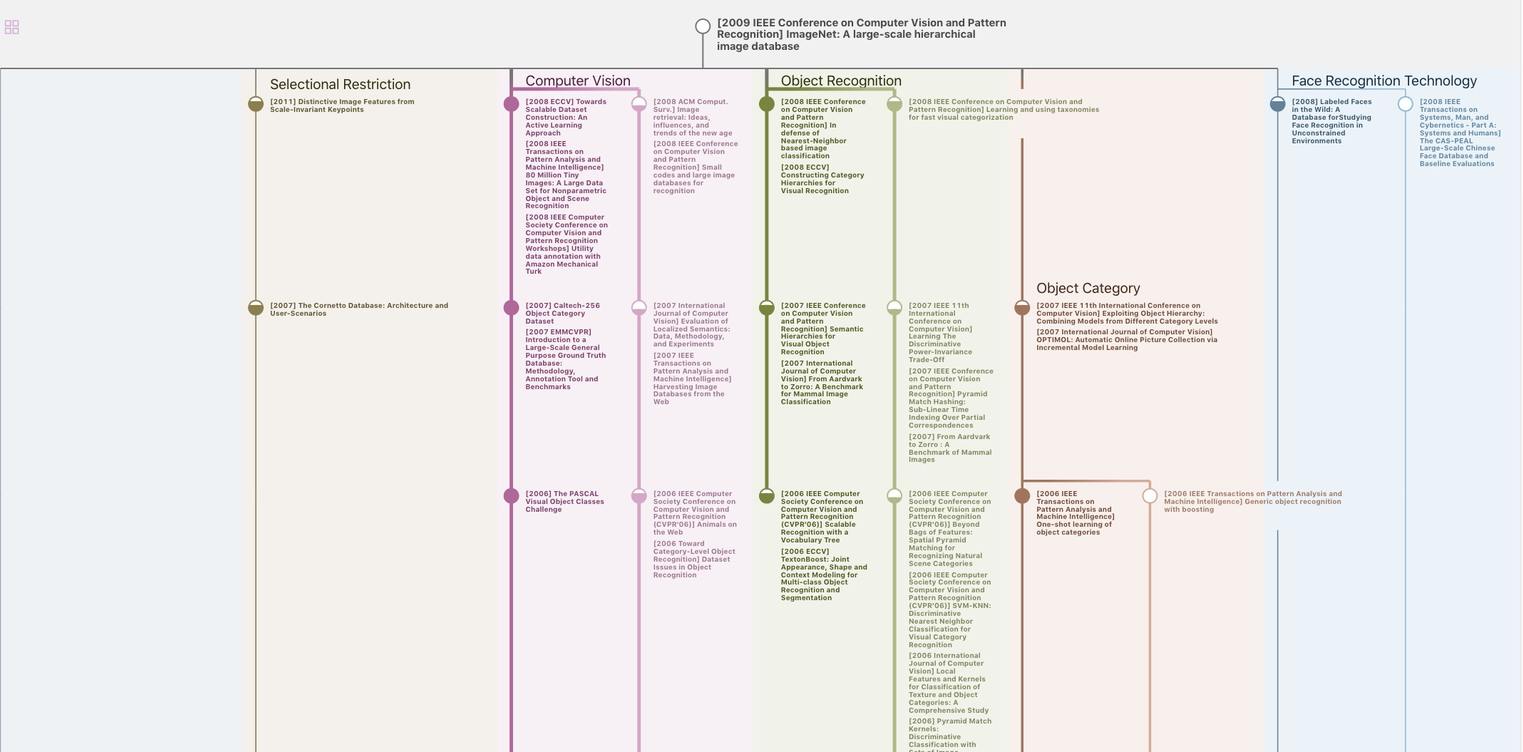
生成溯源树,研究论文发展脉络
Chat Paper
正在生成论文摘要