A Proximal Gradient Algorithm For Composite Consensus Optimization Over Directed Graphs
2017 IEEE 7TH ANNUAL INTERNATIONAL CONFERENCE ON CYBER TECHNOLOGY IN AUTOMATION, CONTROL, AND INTELLIGENT SYSTEMS (CYBER)(2017)
摘要
This paper proposes a decentralized algorithm for solving a consensus optimization problem defined in a directed networked multi-agent system, where the local objective functions have the smooth+nonsmooth composite form. Examples of such problems include decentralized compressed sensing and constrained quadratic programming problems, as well as many decentralized regularization problems. We extend the existing algorithms PG-EXTRA and ExtraPush to a new algorithm PG-ExtraPush for composite consensus optimization over a directed network. This algorithm takes advantage of the proximity operator like in PG-EXTRA to deal with the nonsmooth term, and employs the push-sum protocol like in ExtraPush to tackle the bias introduced by the directed network. We show that PG-ExtraPush converges to an optimal solution under the boundedness assumption. In numerical experiments, with a proper step size, PG-ExtraPush performs surprisingly linear rates, and is significantly faster than Subgradient-Push, even when we hand-optimize the step sizes for the latter.
更多查看译文
关键词
proximal gradient algorithm,composite consensus optimization,directed graphs,decentralized algorithm,consensus optimization problem,local objective functions,smooth+nonsmooth composite form,decentralized regularizatlon problems,constrained quadratic programming problems,PG-EXTRA,directed networked multi-agent system,decentralized compressed sensing,PG-ExtraPush
AI 理解论文
溯源树
样例
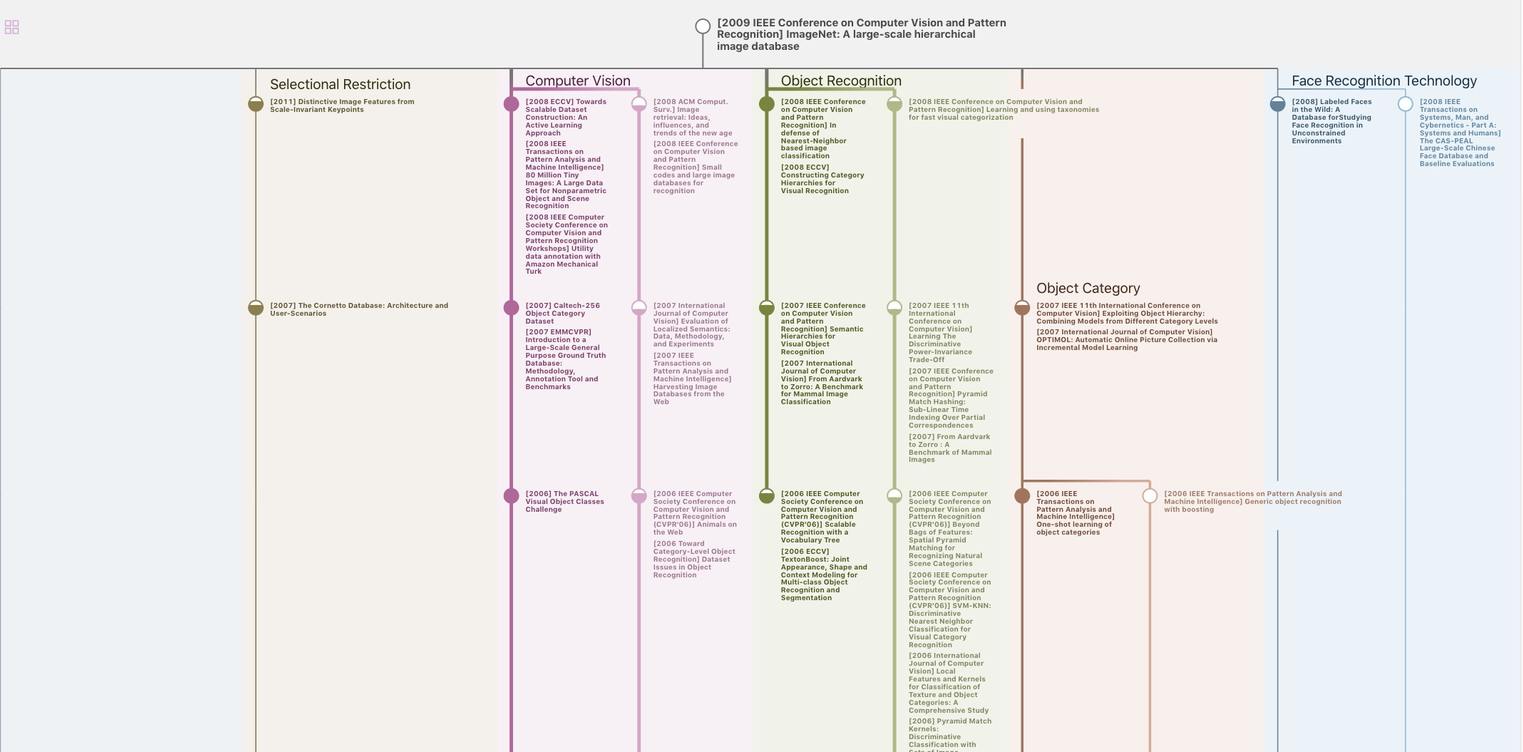
生成溯源树,研究论文发展脉络
Chat Paper
正在生成论文摘要