Multitask Sparse Bayesian Learning with Applications in Structural Health Monitoring
Periodicals(2019)
摘要
AbstractAbstractWe focus on a Bayesian approach to learn sparse models by simultaneously utilizing multiple groups of measurements that are marked by a similar sparseness profile. Joint learning of sparse representations for multiple models has been mostly overlooked, although it is a useful tool for exploiting data redundancy by modeling informative relationships within groups of measurements. To this end, two hierarchical Bayesian models are introduced and associated algorithms are proposed for multitask sparse Bayesian learning (SBL). It is shown that the data correlations for different tasks are taken into account more effectively by using the hierarchical model with a common prediction‐error precision parameter across all related tasks, which leads to a better learning performance. Numerical experiments verify that exploiting common information among multiple related tasks leads to better performance, for both models that are highly and approximately sparse. Then, we examine two applications of multitask SBL in structural health monitoring: identifying structural stiffness losses and recovering missing data occurring during wireless transmission, which exploit information about relationships in the temporal and spatial domains, respectively. These illustrative examples demonstrate the potential of multitask SBL for solving a wide range of sparse approximation problems in science and technology.
更多查看译文
AI 理解论文
溯源树
样例
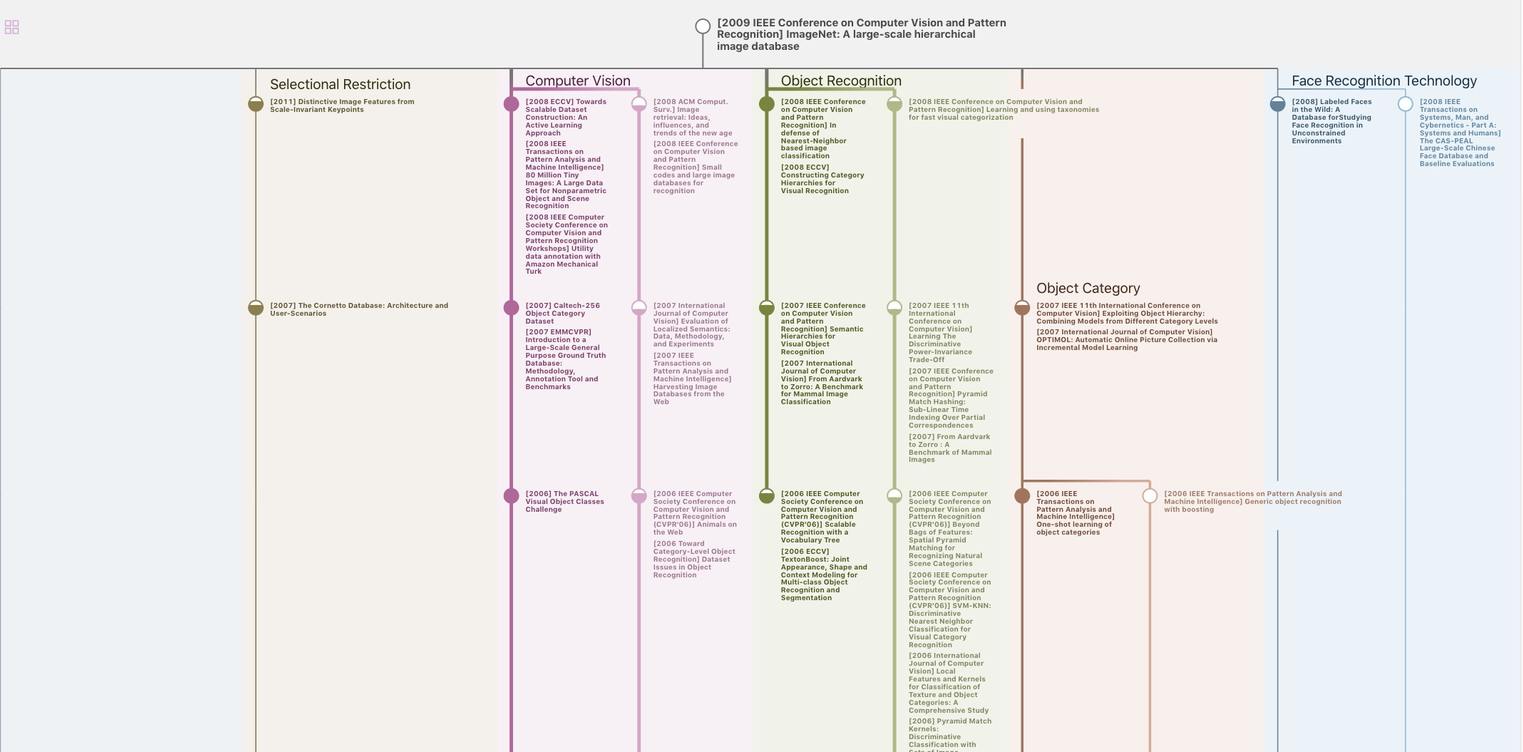
生成溯源树,研究论文发展脉络
Chat Paper
正在生成论文摘要