Reweighting a parton shower using a neural network: the final-state case
Journal of High Energy Physics(2019)
摘要
bstract The use of QCD calculations that include the resummation of soft-collinear logarithms via parton-shower algorithms is currently not possible in PDF fits due to the high computational cost of evaluating observables for each variation of the PDFs. Unfortunately the interpolation methods that are otherwise applied to overcome this issue are not readily generalised to all-order parton-shower contributions. Instead, we propose an approximation based on training a neural network to predict the effect of varying the input parameters of a parton shower on the cross section in a given observable bin, interpolating between the variations of a training data set. This first publication focuses on providing a proof-of-principle for the method, by varying the shower dependence on α S for both a simplified shower model and a complete shower implementation for three different observables, the leading emission scale, the number of emissions and the Thrust event shape. The extension to the PDF dependence of the initial-state shower evolution that is needed for the application to PDF fits is left to a forthcoming publication.
更多查看译文
关键词
Jets
AI 理解论文
溯源树
样例
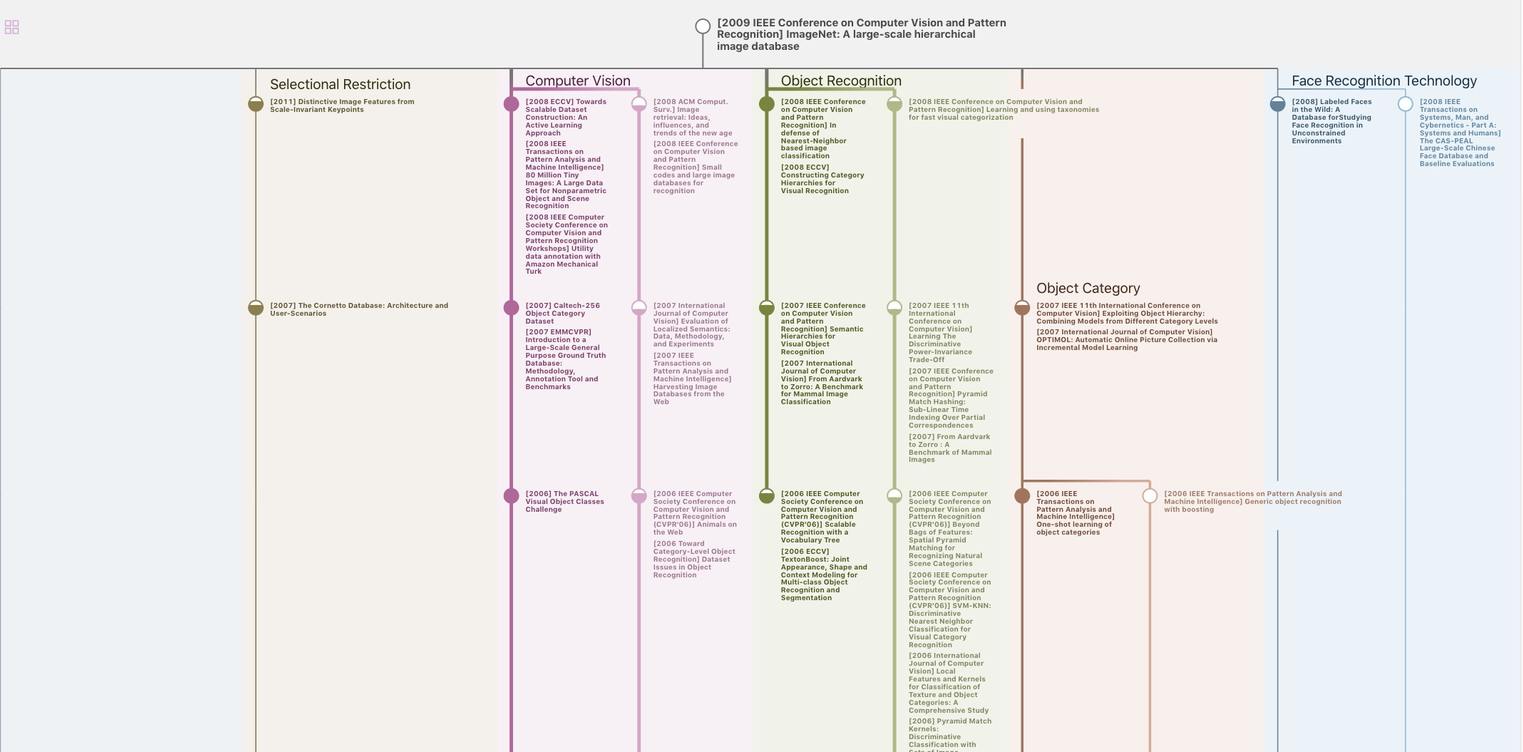
生成溯源树,研究论文发展脉络
Chat Paper
正在生成论文摘要