Parameter identifiability of a respiratory mechanics model in an idealized preterm infant
arXiv: Tissues and Organs(2018)
摘要
The complexity of mathematical models describing respiratory mechanics has grown in recent years to integrate with cardiovascular models and incorporate nonlinear dynamics. However, additional model complexity has rarely been studied in the context of patient-specific observable data. This study investigates parameter identification of a previously developed nonlinear respiratory mechanics model (Ellwein Fix, PLoS ONE 2018) tuned to the physiology of 1 kg preterm infant, using local deterministic sensitivity analysis, subset selection, and gradient-based optimization. The model consists of 4 differential state equations with 31 parameters to predict airflow and dynamic pulmonary volumes and pressures generated under six simulation conditions. The relative sensitivity solutions of the model state equations with respect to each of the parameters were calculated with finite differences and a sensitivity ranking was created for each parameter and simulation. Subset selection identified a set of independent parameters that could be estimated for all six simulations. The combination of these analyses produced a subset of 6 independent sensitive parameters that could be estimated given idealized clinical data. All optimizations performed using pseudo-data with perturbed nominal parameters converged within 40 iterations and estimated parameters within 8 feasibility of performing parameter estimation on real patient-specific data set described by a nonlinear respiratory mechanics model for studying dynamics in preterm infants.
更多查看译文
AI 理解论文
溯源树
样例
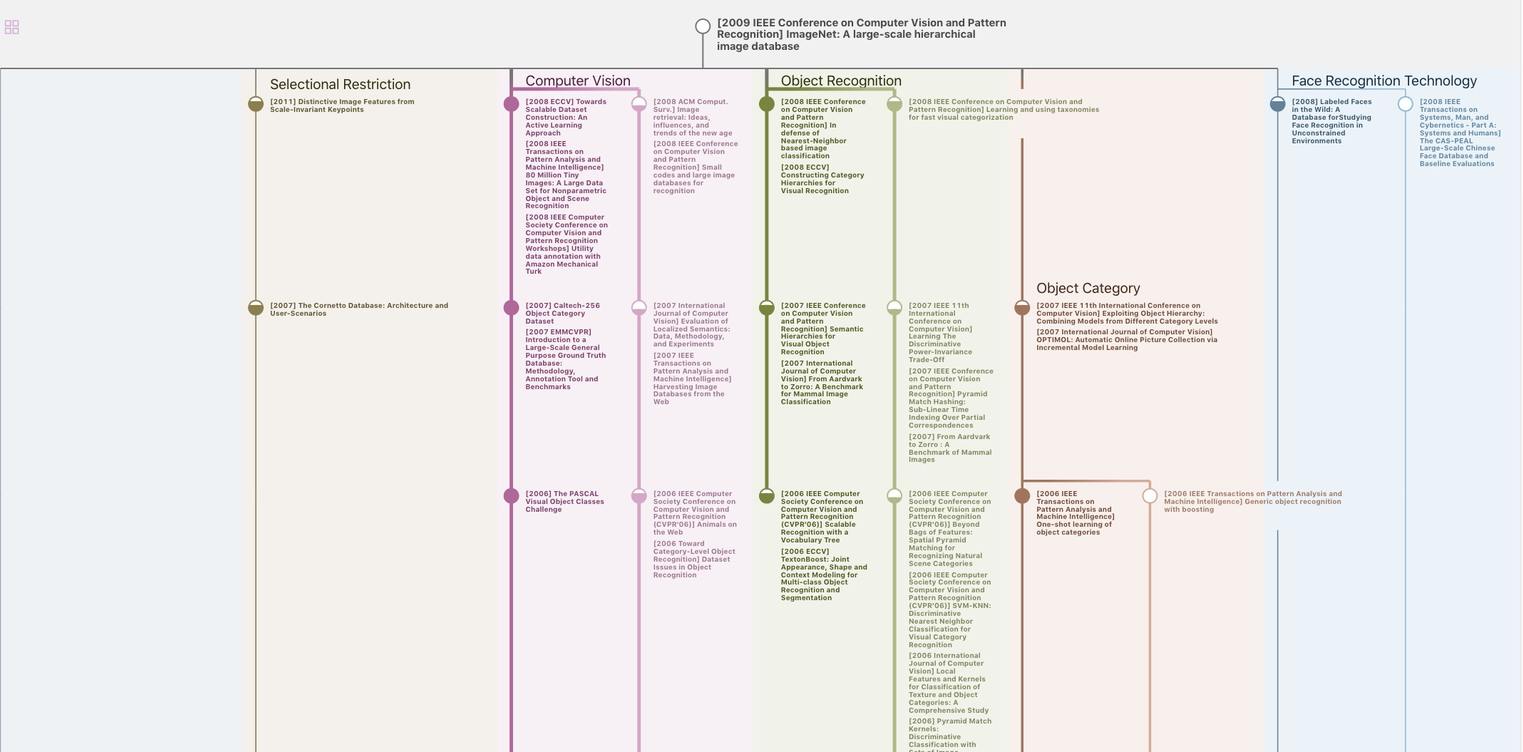
生成溯源树,研究论文发展脉络
Chat Paper
正在生成论文摘要