Machine learning versus econometric jump models in predictability and domain adaptability of index options
Physica A: Statistical Mechanics and its Applications(2019)
摘要
Econometric jump models dealing with key stylized facts in financial option markets have an explicit underlying asset process based on stochastic differential equations. Machine learning models with improved prediction accuracy have elicited considerable attention from researchers in the field of financial application. An intensive empirical study is conducted to compare two methods in terms of model estimation, prediction, and domain adaptation using S&P 100 American/European put options. Results indicated that econometric jump models demonstrate better prediction performance than the best-performing machine learning models, and the estimation results of the former are similar to those of the latter. The former also exhibited significantly better domain adaptation performance than the latter regardless of domain adaptation techniques in machine learning.
更多查看译文
关键词
Financial time series,Lévy process,Bayesian neural network,Neural network,Support vector regression,Gaussian process regression,Domain adaptation
AI 理解论文
溯源树
样例
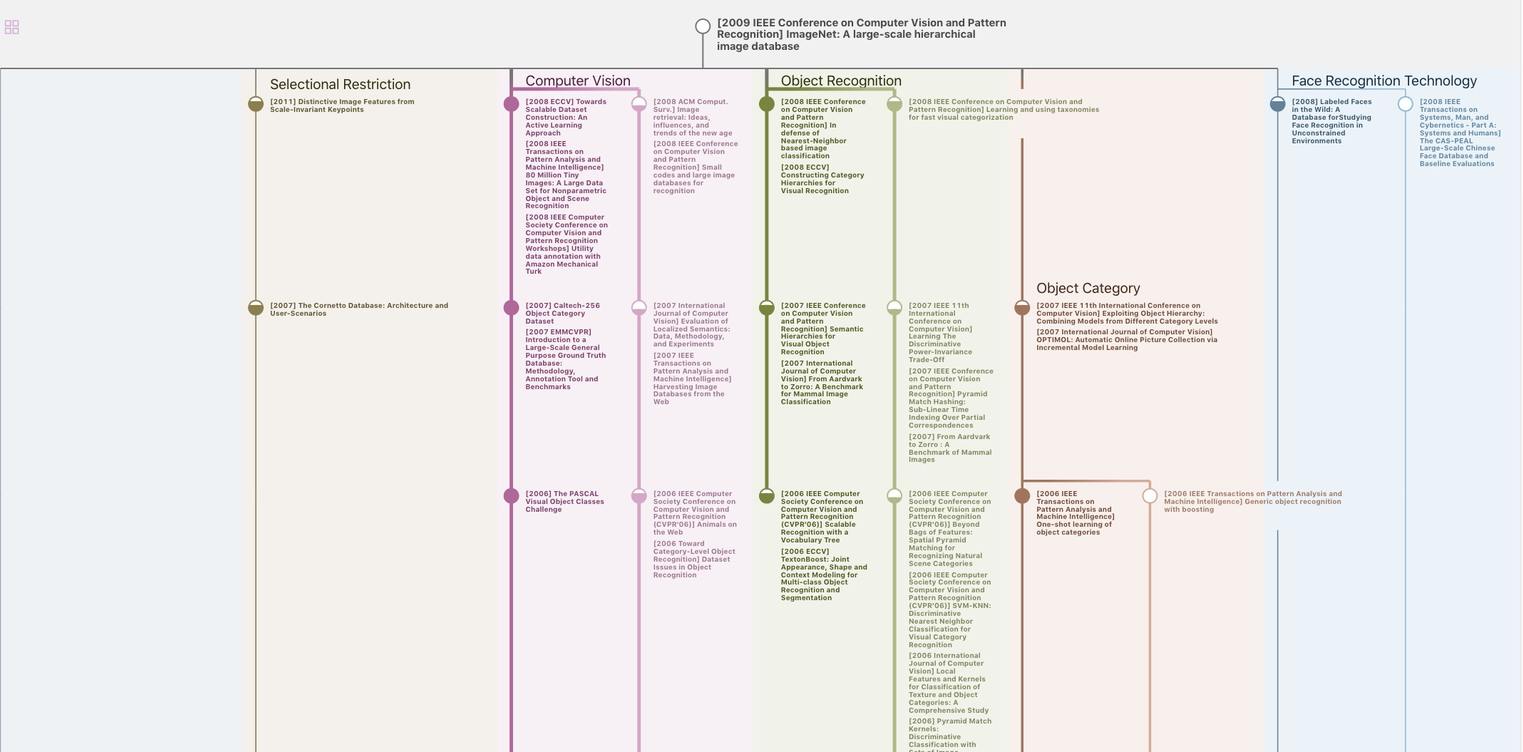
生成溯源树,研究论文发展脉络
Chat Paper
正在生成论文摘要