High Performance Vegetable Classification From Images Based On Alexnet Deep Learning Model
INTERNATIONAL JOURNAL OF AGRICULTURAL AND BIOLOGICAL ENGINEERING(2018)
摘要
Deep learning techniques can automatically learn features from a large number of image data set. Automatic vegetable image classification is the base of many applications. This paper proposed a high performance method for vegetable images classification based on deep learning framework. The AlexNet network model in Caffe was used to train the vegetable image data set. The vegetable image data set was obtained from ImageNet and divided into training data set and test data set. The output function of the AlexNet network adopted the Rectified Linear Units (ReLU) instead of the traditional sigmoid function and the tanh function, which can speed up the training of the deep learning network. The dropout technology was used to improve the generalization of the model. The image data extension method was used to reduce overfitting in the learning process. With AlexNet network model used for training different number of vegetable image data set, the experimental results showed that the classification accuracy decreases as the number of data set decreases. The experimental verification indicated that the accuracy rate of the deep learning method in the test data set reached as high as 92.1%, which was greatly improved compared with BP neural network (78%) and SVM classifier (80.5%) methods.
更多查看译文
关键词
vegetable classification, deep learning, Caffe, AlexNet Network, ImageNet
AI 理解论文
溯源树
样例
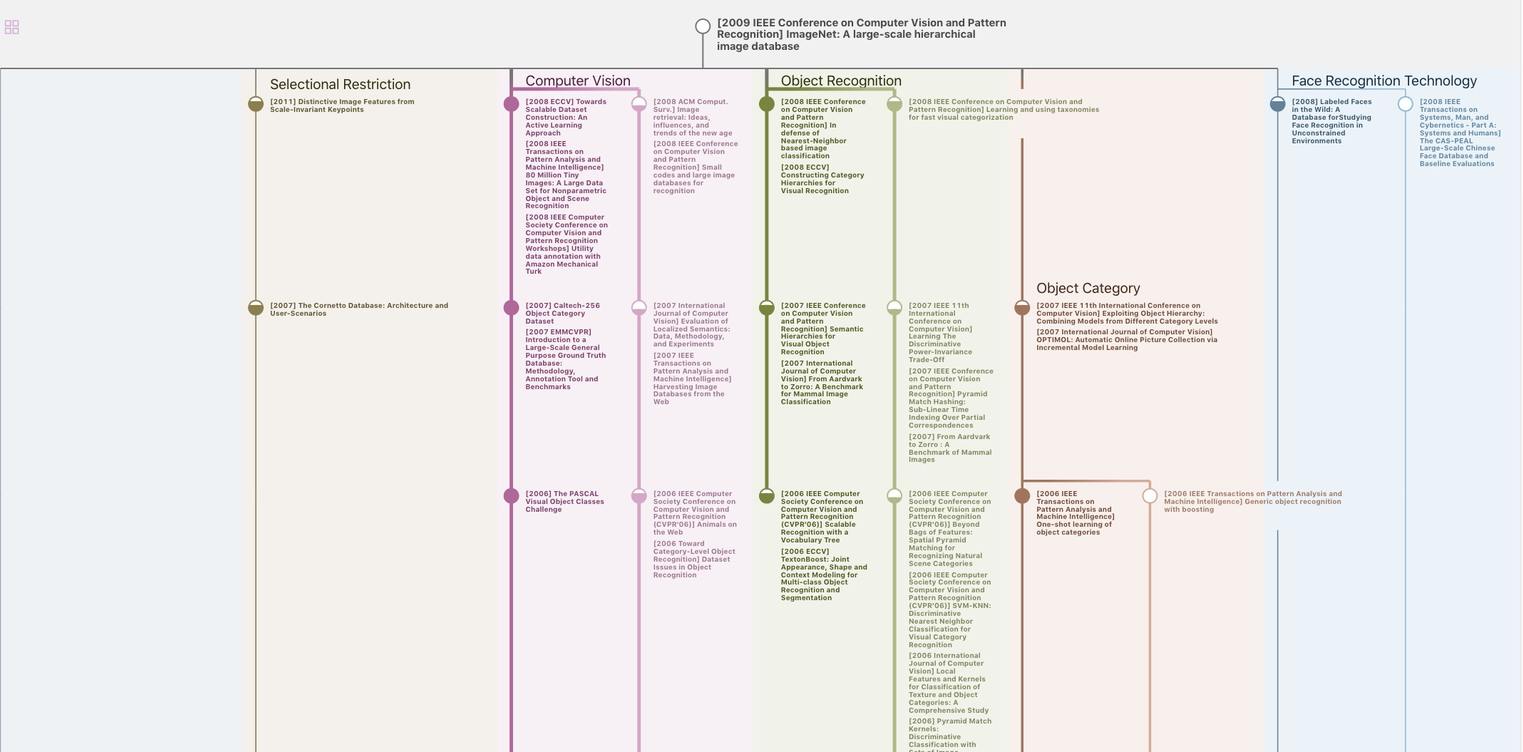
生成溯源树,研究论文发展脉络
Chat Paper
正在生成论文摘要