Accelerated Bregman proximal gradient methods for relatively smooth convex optimization
COMPUTATIONAL OPTIMIZATION AND APPLICATIONS(2021)
摘要
We consider the problem of minimizing the sum of two convex functions: one is differentiable and relatively smooth with respect to a reference convex function, and the other can be nondifferentiable but simple to optimize. We investigate a triangle scaling property of the Bregman distance generated by the reference convex function and present accelerated Bregman proximal gradient (ABPG) methods that attain an O(k^-γ) convergence rate, where γ∈ (0,2] is the triangle scaling exponent (TSE) of the Bregman distance. For the Euclidean distance, we have γ =2 and recover the convergence rate of Nesterov’s accelerated gradient methods. For non-Euclidean Bregman distances, the TSE can be much smaller (say γ≤ 1 ), but we show that a relaxed definition of intrinsic TSE is always equal to 2. We exploit the intrinsic TSE to develop adaptive ABPG methods that converge much faster in practice. Although theoretical guarantees on a fast convergence rate seem to be out of reach in general, our methods obtain empirical O(k^-2) rates in numerical experiments on several applications and provide posterior numerical certificates for the fast rates.
更多查看译文
关键词
Convex optimization, Relative smoothness, Bregman divergence, Proximal gradient methods, Accelerated gradient methods
AI 理解论文
溯源树
样例
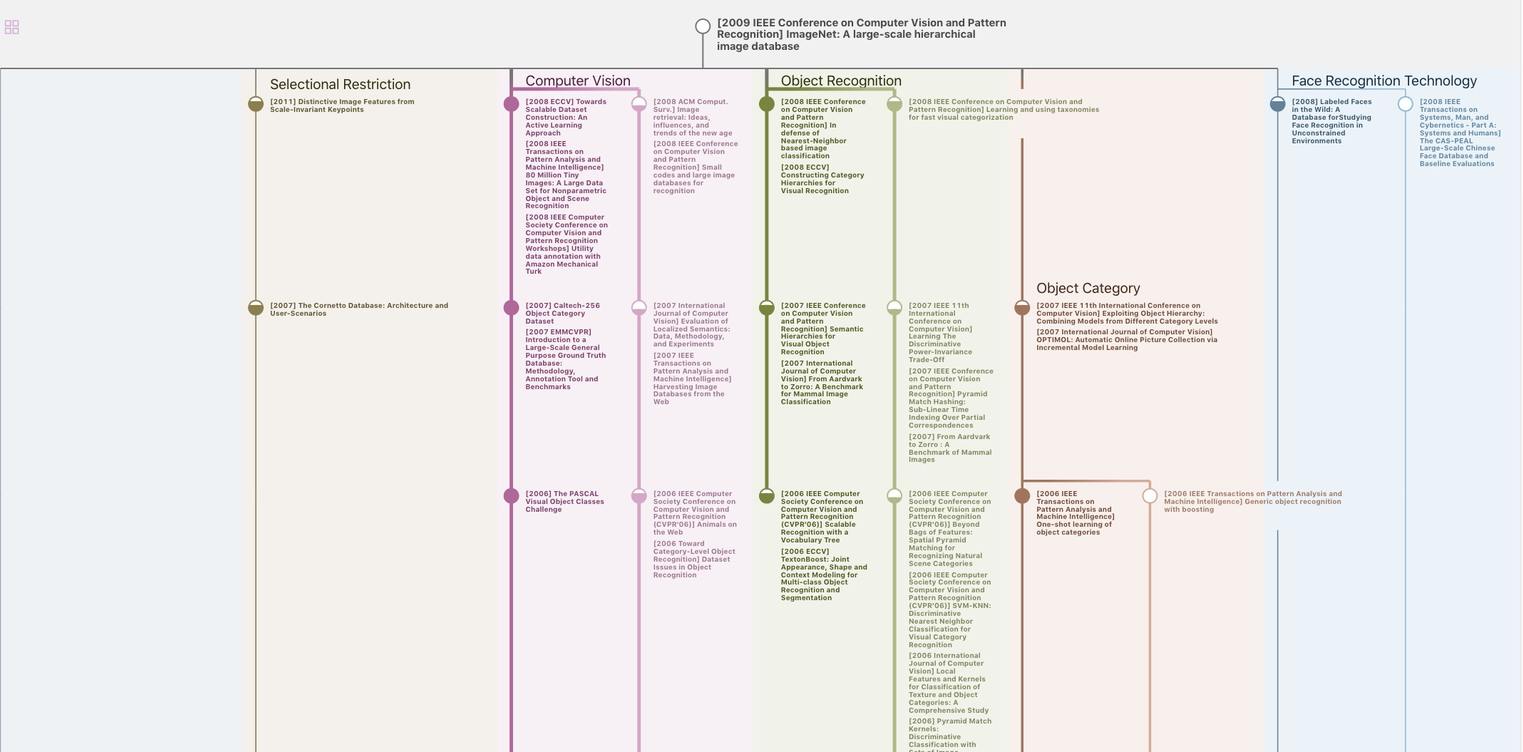
生成溯源树,研究论文发展脉络
Chat Paper
正在生成论文摘要