Connecting natural and artificial neural networks in functional brain imaging using structured sparsity
bioRxiv(2018)
摘要
Artificial neural network models have long proven useful for understanding healthy, disordered, and developing cognition, but this work has often proceeded with little connection to functional brain imaging. We consider how analysis of functional brain imaging data is best approached if the representational assumptions embodied by neural networks are valid. Using a simple model to generate synthetic data, we show that four contemporary methods each have critical and complementary blind-spots for detecting distributed signal. The pattern suggests a new approach based on structured sparsity that, in simulation, retains the strengths of each method while avoiding its weaknesses. When applied to functional magnetic resonance imaging data the new approach reveals extensive distributed signal missed by the other methods, suggesting radically different conclusions about how brains encode cognitive information in the well-studied domain of visual face perception.
更多查看译文
关键词
neural network models,multivariate pattern analysis,fMRI,face representation,structured sparsity
AI 理解论文
溯源树
样例
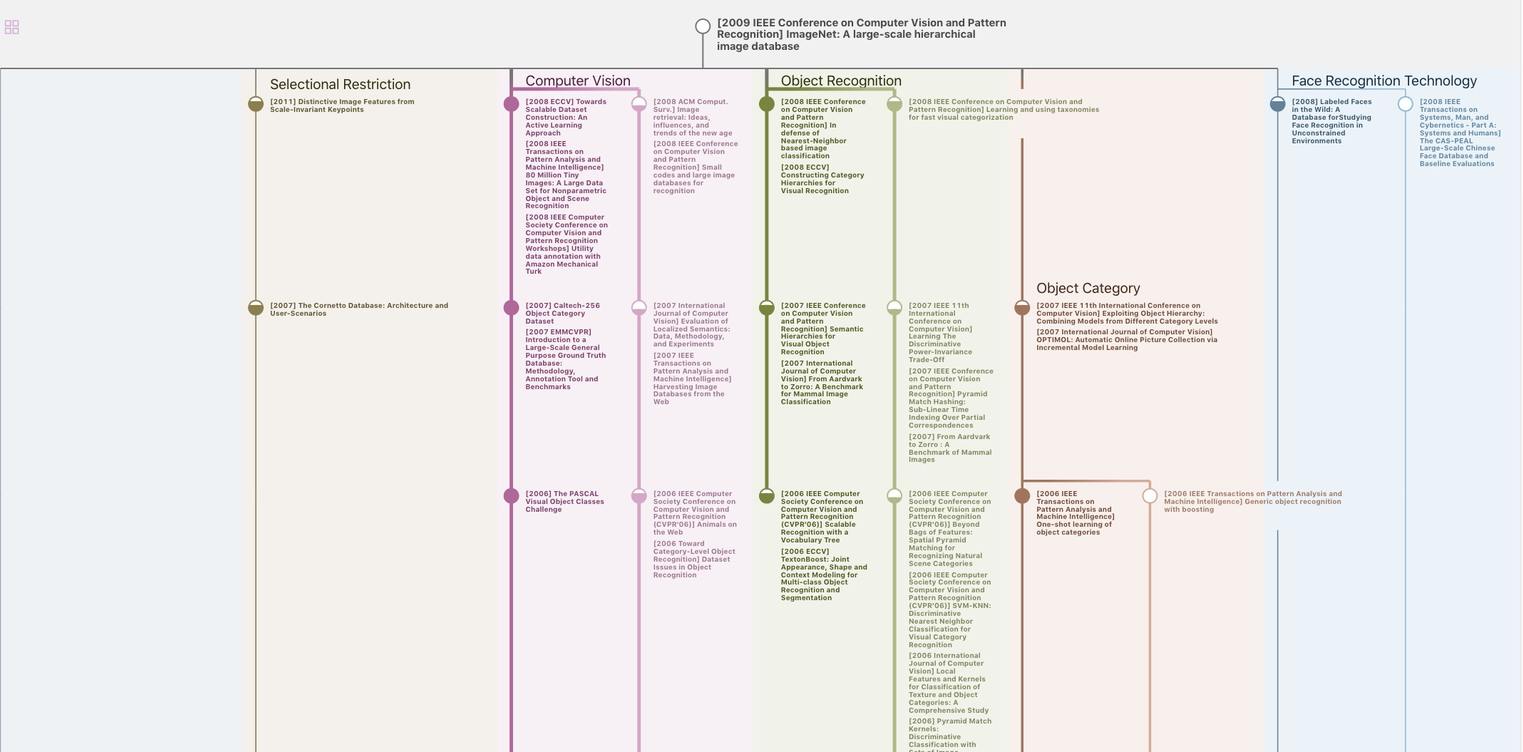
生成溯源树,研究论文发展脉络
Chat Paper
正在生成论文摘要