Nonlinear Time-series Mining of Social Influence
BIG DATA APPLICATIONS AND SERVICES 2017(2019)
摘要
Given a large collection of time-evolving online user activities, such as Google Search queries for multiple keywords of various categories (celebrities, events, diseases, etc.), which consist of d keywords/activities, for l countries/locations of duration n, how can we find patterns and rules? For example, assume that we have the online search volume for "Harry Potter", "Barack Obama", and "Amazon", for 232 countries/territories, from 2004 to 2015, which include external shocks, sudden change of search volume, and more. How do we go about capturing nonlinear evolutions of local activities and forecasting future patterns? In this paper, we present.-SPOT, a unifying analytical nonlinear model for analyzing large-scale web search data, which is sensemaking, automatic, scalable, and free of parameters. Delta-SPOT can also forecast long-range future dynamics of the keywords/queries. We use the Google Search, Twitter, and MemeTracker dataset for extensive experiments, which show that our method outperforms other effective methods of nonlinear mining in terms of accuracy and in both fitting and forecasting.
更多查看译文
关键词
Time-series analysis,Social influence analysis,Parameter-free
AI 理解论文
溯源树
样例
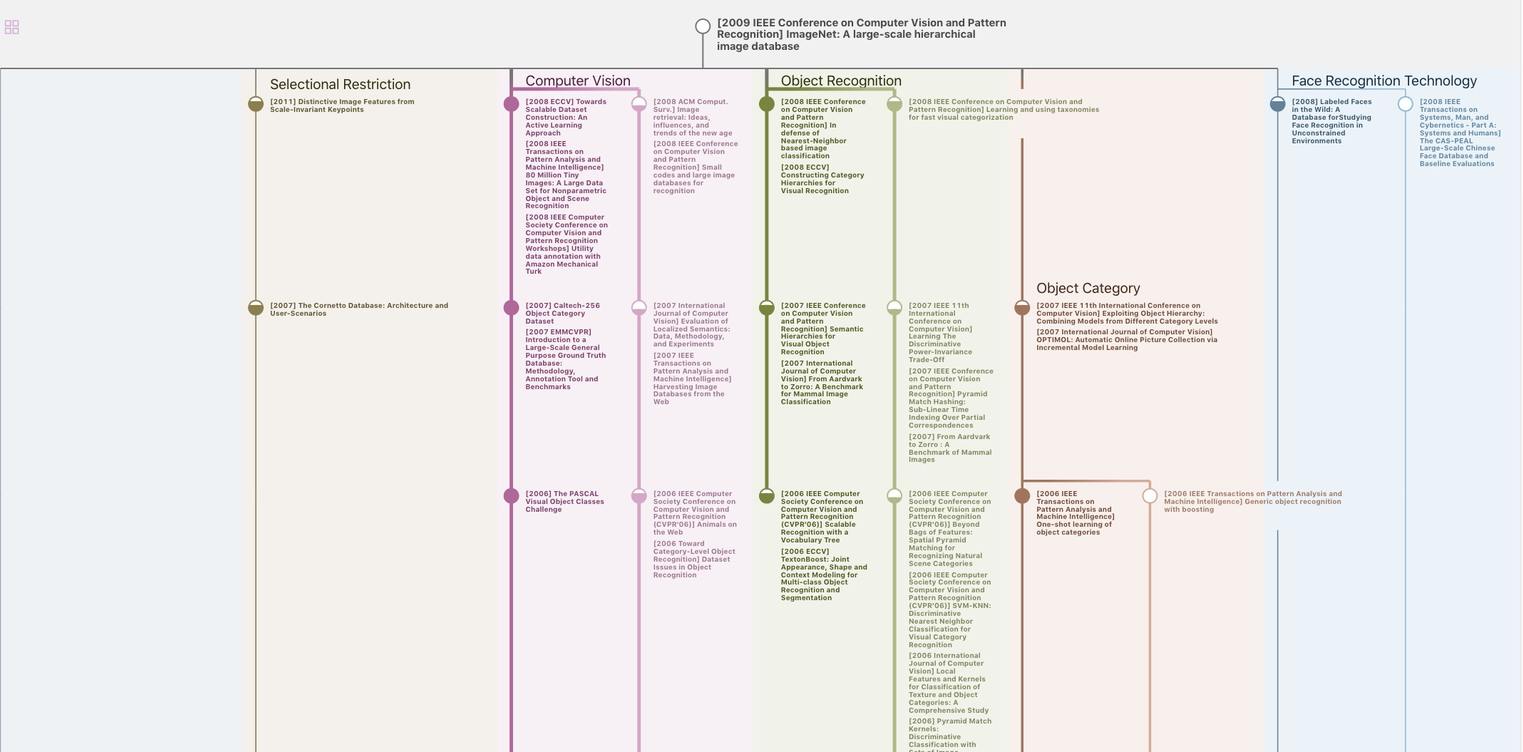
生成溯源树,研究论文发展脉络
Chat Paper
正在生成论文摘要