Demographic inference using particle filters for continuous Markov jump processes
bioRxiv(2020)
摘要
Demographic events shape a population9s genetic diversity, with the coalescent-with-recombination (CwR) model quantifying how the two are related through a sequence of genealogies. However, inferring demography from genome sequences is challenging because genealogies are only weakly constrained by polymorphisms, and the space of genealogies over genomes is huge. Here we show that particle filters are a promising approach to demographic inference. We introduce a continuous-sequence approximation of the CwR, resulting in a continuous-time and -space Markov jump process. We develop a particle filter algorithm for such processes, and introduce the unbounded-lookahead particle filter, generalising the unit-lookahead Auxiliary Particle Filter for discrete-time models. Using Variational Bayes for parameter inference, we can analyze up to four diploid samples simultaneously, resulting in accurate inferences of past population sizes over a larger range of epochs than was previously possible.
更多查看译文
关键词
Continuous-time continuous-space Markov jump process,Particle filter,Sequential Monte Carlo,Variational Bayes,Demo-graphic Inference
AI 理解论文
溯源树
样例
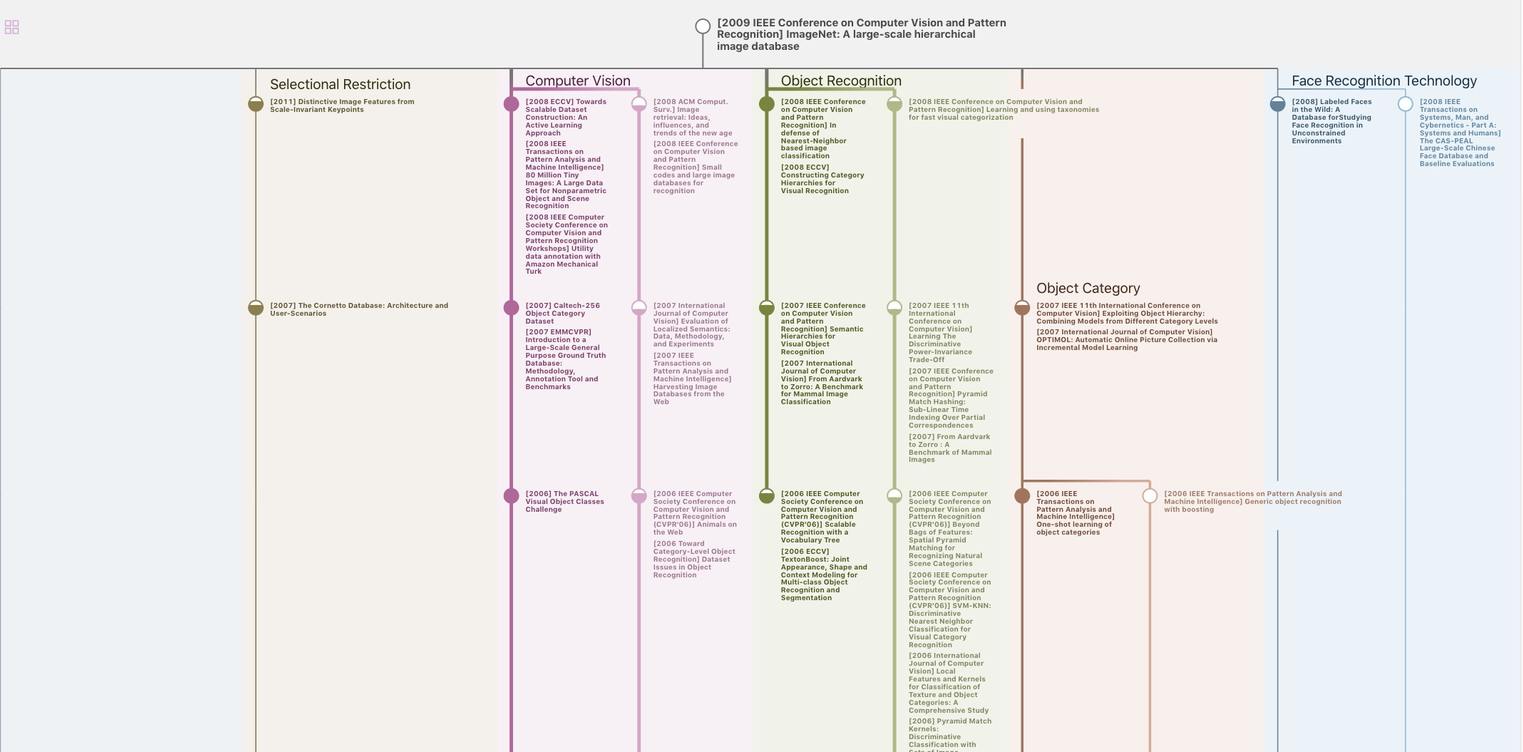
生成溯源树,研究论文发展脉络
Chat Paper
正在生成论文摘要