Feature importance ranking for classification in mixed online environments
Annals of Operations Research(2018)
摘要
Online learning is a growing branch of machine learning with applications in many domains. One of the less studied topics in this area is development of strategies for online feature importance ranking. In this paper we present two methods for incremental ranking of features in classification tasks. Our ranking strategies are based on measurement of the sensitivity of the classification outcome with respect to individual features. The two methods work for different types of classification environments with discrete, continuous and mixed feature types with minimum prior assumptions. The second method, which is a modification of the original method, is designed to handle concept drift while avoiding cumbersome computations. Concept drift is described as sudden or slow changes in characteristics of the learning features which happens in many online learning tasks such as online marketing analysis. If the rankings are not adaptable, during the time, these changes will make the rankings obsolete. Moreover, we investigate different feature selection schemes for feature reduction in online environments to effectively remove irrelevant features from the classification model. Finally, we present experimental results which verify the efficacy of our methods against currently available online feature ranking algorithms.
更多查看译文
关键词
Feature ranking, Online learning, Stochastic gradient descent, Mixed feature space
AI 理解论文
溯源树
样例
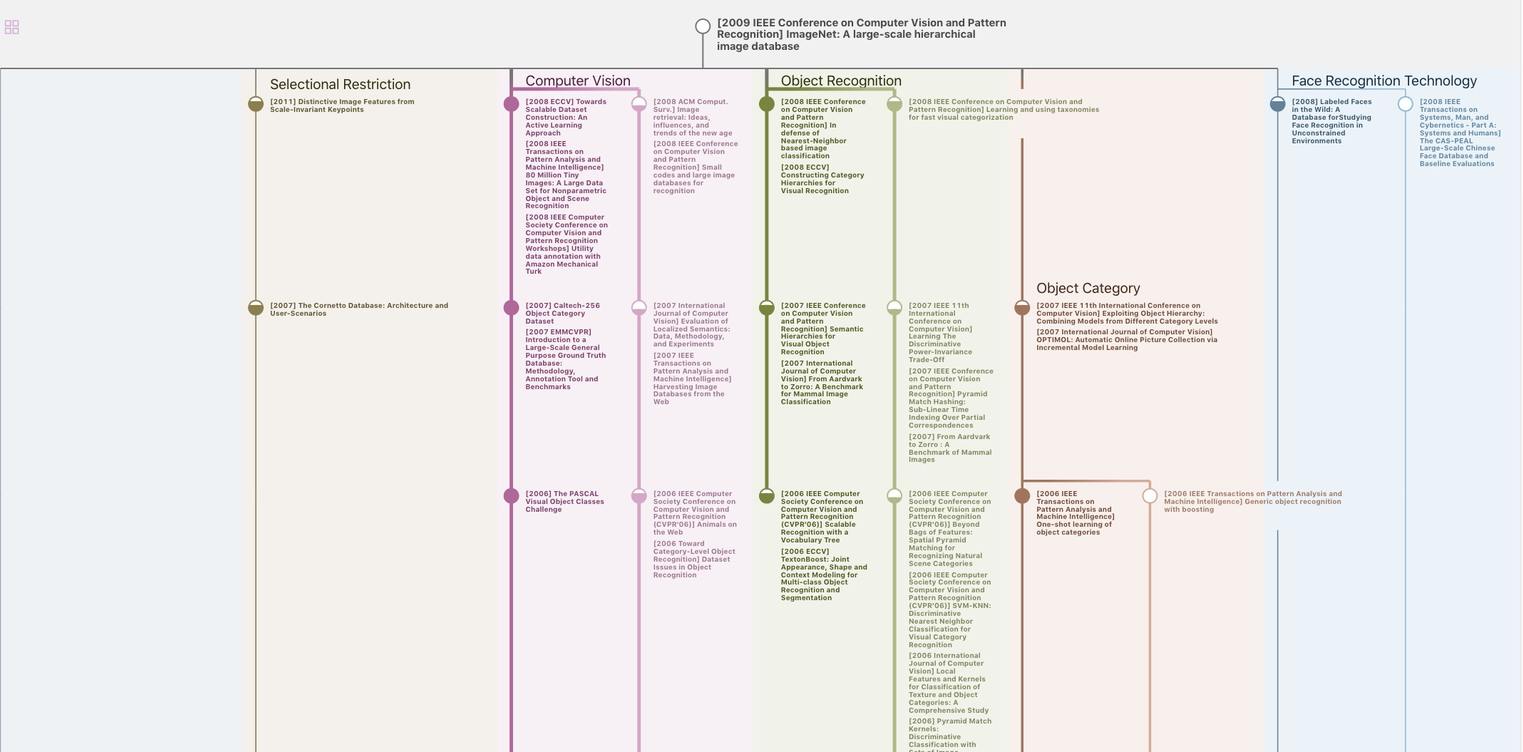
生成溯源树,研究论文发展脉络
Chat Paper
正在生成论文摘要