Modeling functional enrichment improves polygenic prediction accuracy in UK Biobank and 23andMe data sets
bioRxiv(2020)
摘要
Genetic variants in functional regions of the genome are enriched for complex trait heritability. Here, we introduce a new method for polygenic prediction, LDpred-funct, that leverages trait-specific functional enrichments to increase prediction accuracy. We fit priors using the recently developed baseline-LD model, which includes coding, conserved, regulatory and LD-related annotations. We analytically estimate posterior mean causal effect sizes and then use cross-validation to regularize these estimates, improving prediction accuracy for sparse architectures. LDpred-funct attained higher prediction accuracy than other polygenic prediction methods in simulations using real genotypes. We applied LDpred-funct to predict 16 highly heritable traits in the UK Biobank. We used association statistics from British-ancestry samples as training data (avg N=365K) and samples of other European ancestries as validation data (avg N=22K), to minimize confounding. LDpred-funct attained a +27% relative improvement in prediction accuracy (avg prediction R 2 =0.173; highest R 2 =0.417 for height) compared to existing methods that do not incorporate functional information, consistent with simulations. For height, meta-analyzing training data from UK Biobank and 23andMe cohorts (total N=1107K; higher heritability in UK Biobank cohort) increased prediction R 2 to 0.429. Our results show that modeling functional enrichment substantially improves polygenic prediction accuracy, bringing polygenic prediction of complex traits closer to clinical utility.
更多查看译文
AI 理解论文
溯源树
样例
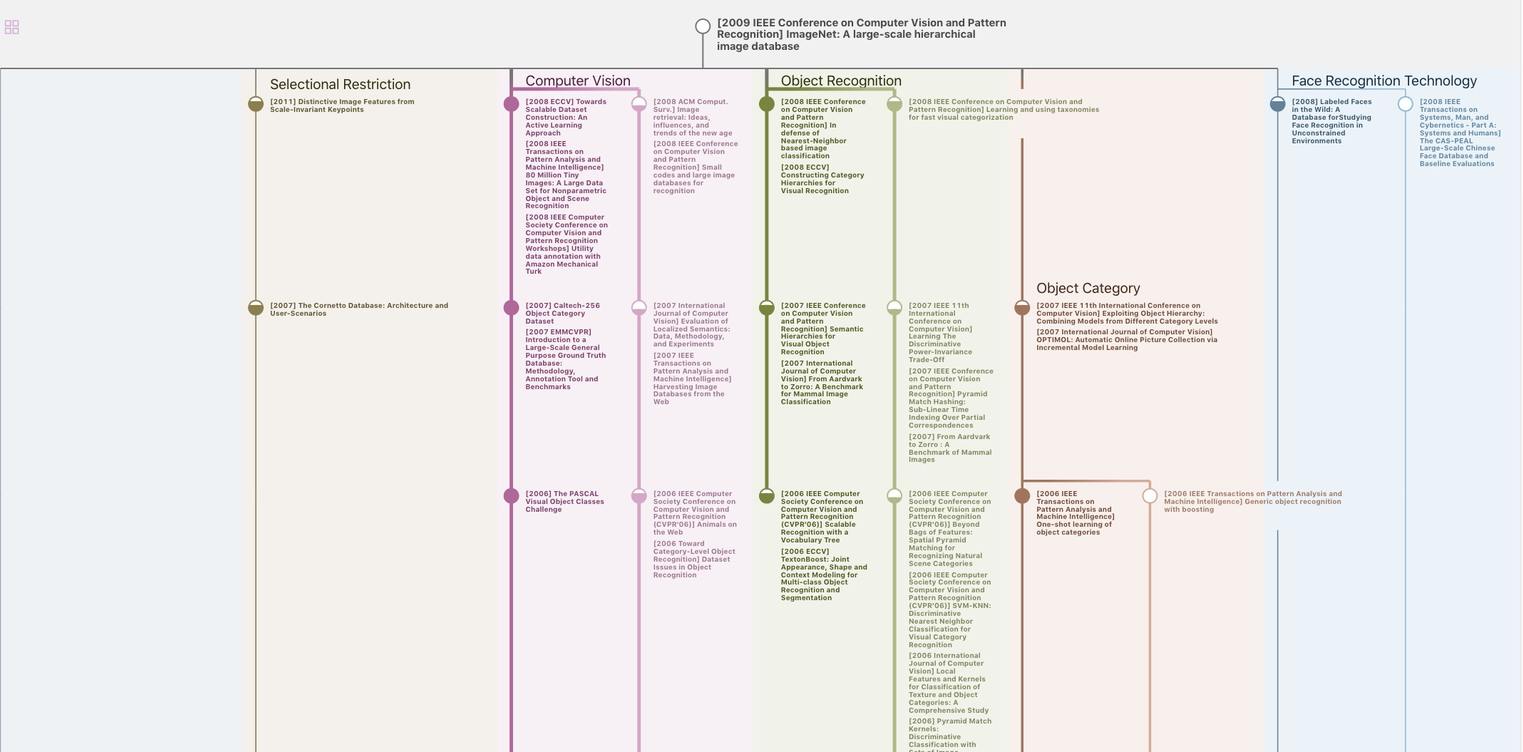
生成溯源树,研究论文发展脉络
Chat Paper
正在生成论文摘要